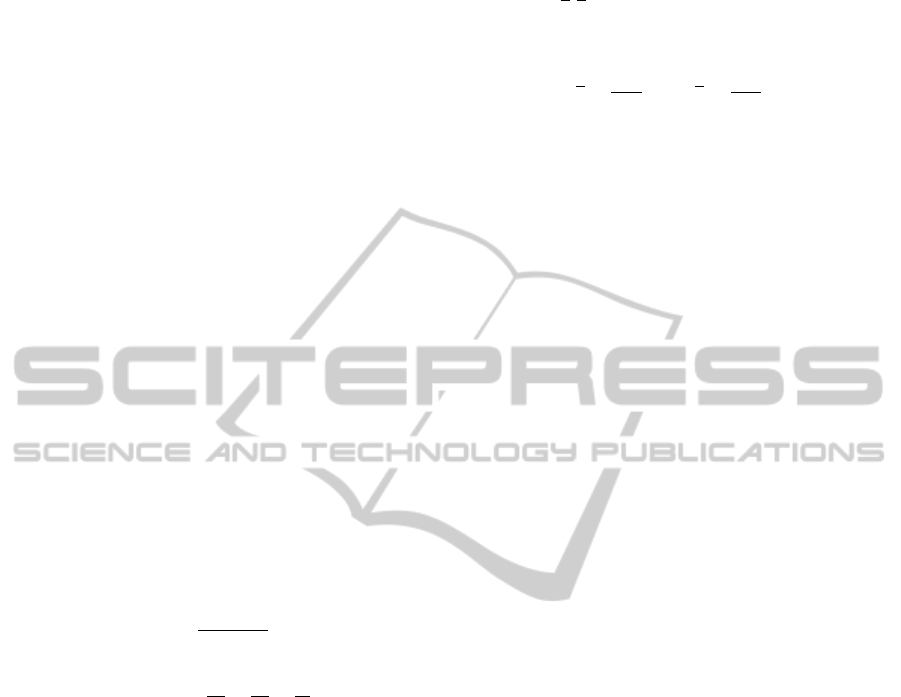
3 FEATURE DESCRIPTION
By interpreting a region’s associated pixels as a two-
dimensional Gaussian distribution in the image plane,
a region can be implicitly described by means of sta-
tistical parameters, namely the two mean values m
x
and m
y
, the two variances σ
2
x
and σ
2
y
, and the covari-
ance σ
xy
. A generalization of these specific parame-
ters are the statistical moments, which can be directly
applied in our context in discretized form (Hu, 1962):
the two mean values correspond to the two moments
of first order (m
10
and m
01
), whereas the two vari-
ances and the covariance correspond to the central-
ized (or central) moments of second order (µ
20
, µ
02
and µ
11
). In addition, the mass of a region is equiva-
lent to the moment of zeroth order (m
00
).
Since the central moments can be directly derived
from the moments through second order (Hu, 1962),
the basic feature descriptor for representing an ex-
tracted region is given by the following set M of mo-
ments:
M = {m
pq
|p + q ≤ 2}
= {m
00
, m
10
, m
01
, m
11
, m
20
, m
02
}
(4)
For efficiently computing the required five mo-
ments, the runs of a region can be directly used by
applying the Delta ”δ” Method (Zakaria et al., 1987).
In this context, S1
i
and S2
i
are defined as follows:
S1
i
=
δ
i
−1
∑
k=0
k =
(δ
2
i
− δ
i
)
2
S2
i
=
δ
i
−1
∑
k=0
k
2
=
δ
3
i
3
−
δ
2
i
2
+
δ
i
6
,
(5)
with δ
i
corresponding to the length l
i
of a single run
run
i
. The required geometric moments m
00
i
, m
10
i
,
m
01
i
, m
11
i
, m
20
i
and m
02
i
of run
i
can then be com-
puted in the following way:
m
00
i
= δ
i
m
01
i
= δ
i
· y
i
m
02
i
= δ
i
· y
2
i
,
m
10
i
= δ
i
· x
i
+ S1
i
m
11
i
= y
i
· [δ
i
· x
i
+ S1
i
] = y
i
· m
10
i
m
20
i
= δ
i
· x
2
i
+ 2 · S1
i
· x
i
+ S2
i
(6)
Finally, the moments of an entire region R correspond
to the sums of the particular moments of all associated
n runs:
M = {m
pq
|m
pq
=
n
∑
i=1
m
pq
i
∧ p + q ≤ 2} (7)
For representing a feature in a more explicit man-
ner, additional geometric attributes can be derived
from M and the associated central moments (Teague,
1980; Prokop and Reeves, 1992). In this context, the
coordinates (x, y) of the center of mass of an extracted
feature in the image plane are defined by the moments
of zeroth and first order:
x =
m
10
m
00
and y =
m
01
m
00
(8)
Furthermore, due to the statistical interpretation in
terms of a Gaussian distribution, a feature is equiv-
alent to a elliptical disk with constant intensity, hav-
ing definite size, orientation and eccentricity and be-
ing centered at the origin of the image plane. The
lengths of its major and minor axes as well as its an-
gle of inclination can be computed with the use of the
associated central moments (Teague, 1980). By com-
bining both the center of mass and the elliptical disk,
a feature can be geometrically described in terms of
an ellipse that is located at the center of mass of the
respective feature (cf. Figure 3).
4 RESULTS
The image segmentation approach was implemented
in C/C++ and successfully applied to the BeBot.
While capturing a YUV image of size 320x240 pix-
els, the algorithm enables us to process at least 10
entire images per second. Regarding the load factor
of the BeBot during our experiments, the overall per-
formance of the segmentation algorithm heavily de-
pends on the number of different regions that are con-
structed, which in turn depends on the values of the
calibration parameters in combination with the homo-
geneity of the original image.
Figure 2 shows different segmentation results with
respect to different values of the calibration parame-
ters c
1
and c
2
of heuristic H
yuv
. Whereas the goal of
the algorithm was to clearly extract the colored ob-
jects (four balls and one rectangular block), we con-
structed the background (the horizon) as inhomoge-
neous as possible (see Figure 2(a)). From Figure 2(c)
to Figure 2(d), only parameter c
1
for modifying the
influence of the luma component Y was changed. By
increasing c
1
, the segmented image becomes more
and more homogeneous in comparison to Figure 2(b).
The same holds true when the second parameter c
2
of
heuristic H
yuv
is exclusively modified (Figure 2(e) to
Figure 2(f)). However, when comparing Figure 2(c)
and Figure 2(f), changing the allowed range of luma
similarity (c
1
) seems to have a greater influence than
changing the allowed range of chrominance similarity
(c
2
). Keeping that in mind, Figure 2(g) shows a great
overall result with respect to the homogeneity of the
IMAGE SEGMENTATION FOR OBJECT DETECTION ON A DEEPLY EMBEDDED MINIATURE ROBOT
443