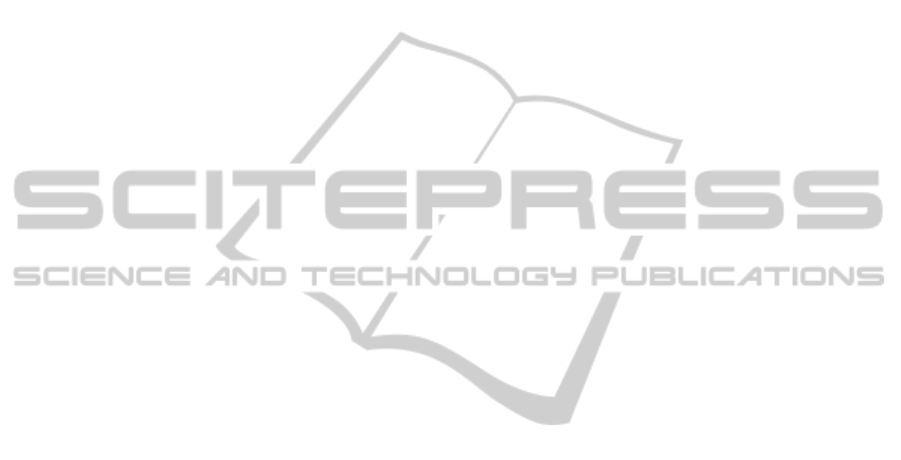
estimate a spatial filter, Parafac also takes into account
the time varying information represented along time
axis, in its 3-D data array representation. Thus, in
comparison to other spatial filters, Parafac offers the
unique possibility to consider time-varying SSVEP
responses, which can be viewed as an advantage: the
subject is not expected to always keep the same level
of concentration on the stimulus, but rather to become
disturbed and tired. The latter could be verified with
the presence of a temporary high alpha power in the
recordings.
One can argue against Parafac since the classifi-
cation accuracy is not much higher when considered
for BCI. But we want to point out that we showed
the classification results for the frequency pair and
quadruple that performed, on average, best among all
subjects. This means that it is a default setting in-
tended to be suited for a broad group of subjects. The
best set of frequencies is expected to be subject de-
pendent (for some subjects the SSVEP responses are
best at lower, for others at higher frequencies, and
even for some subject no detection performance could
be achieved (so called BCI illiteracy)). This was also
observed in our experiment, where the frequencies
leading to the highest detection accuracy were subject
dependent. Thus, for a particular subject we can find
the best frequencies through some calibration proce-
dure (scanning of the SSVEP responses to different
stimulation frequencies) and then construct the de-
coder based on them.
Another point of concern, when applying the de-
scribed methods to BCI, is that Parafac relies on an
iterative procedure for determining the coefficients of
the decomposition. This makes Parafac not suited for
real-time applications, however, we hasten to add that
adaptive algorithms for Parafac decomposition have
already been described, bringing on-line applications
within reach (Nion and Sidiropoulos, 2009).
As a conclusion, we can say that Parafac is po-
tentially useful for SSVEP detection, and for SSVEP-
based BCI, but further research is required to improve
the detection accuracy.
REFERENCES
Acar, E., Aykut-Bingol, C., Bingol, H., Bro, R., and Yener,
B. (2007). Multiway analysis of epilepsy tensors.
Bioinformatics, 23(13):i10.
Bro, R. (1997). Parafac. Tutorial and applications. Chemo-
metrics and intelligent laboratory systems, 38(2):149–
171.
Bro, R. and Kiers, H. (2003). A new efficient method for de-
termining the number of components in Parafac mod-
els. Journal of Chemometrics, 17(5):274–286.
Chumerin, N., Manyakov, N., Combaz, A., Robben, A.,
van Vliet, M., and Van Hulle, M. (2011). Subject-
adaptive steady-state visual evoked potential detection
for brain-computer interface. In The 6th IEEE Inter-
national Conference on Intelligent Data Acquisition
and Advanced Computing Systems: Technology and
Applications (IDAACS2011), pages 369–373.
Cichocki, A., Washizawa, Y., Rutkowski, T., Bakardjian,
H., Phan, A., Choi, S., Lee, H., Zhao, Q., Zhang,
L., and Li, Y. (2008). Noninvasive BCIs: Multiway
signal-processing array decompositions. Computer,
41(10):34–42.
Cichocki, A., Zdunek, R., Phan, A., and Amari, S. (2009).
Nonnegative matrix and tensor factorizations: appli-
cations to exploratory multi-way data analysis and
blind source separation. Wiley.
De Vos, M., De Lathauwer, L., Vanrumste, B., Van Huf-
fel, S., and Van Paesschen, W. (2007). Canonical de-
composition of ictal scalp EEG and accurate source
localisation: Principles and simulation study. Compu-
tational Intelligence and Neuroscience, 2007:1–8.
Friman, O., Volosyak, I., and Gr¨aser, A. (2007). Multiple
channel detection of steady-state visual evoked poten-
tials for brain-computer interfaces. IEEE Transactions
on Biomedical Engineering, 54(4):742–750.
Gao, Y., R., W., X., G., B., H., and S., G. (2006). A practical
VEP-based brain-computer interface. IEEE Transac-
tions on Neural Systems and Rehabilitation Engineer-
ing, 14(2).
Herrmann, C. (2001). Human EEG responses to 1–100 Hz
flicker: resonance phenomena in visual cortex and
their potential correlation to cognitive phenomena.
Experimental Brain Research, 137(3):346–353.
Manyakov, N., Chumerin, N., Combaz, A., Robben, A., and
Van Hulle, M. (2010). Decoding SSVEP Responses
Using Time Domain Classification. In Proc. of the
International Conference on Fuzzy Computation and
2nd International Conference on Neural Computa-
tion, pages 376–380.
Miwakeichi, F., Martinez-Montes, E., Vald´es-Sosa, P.,
Nishiyama, N., Mizuhara, H., and Yamaguchi, Y.
(2004). Decomposing EEG data into space-time-
frequency components using parallel factor analysis.
NeuroImage, 22(3):1035–1045.
Nion, D. and Sidiropoulos, N. (2009). Adaptive algo-
rithms to track the Parafac decomposition of a third-
order tensor. IEEE Transactions on Signal Processing,
57(6):2299–2310.
Segers, H., Combaz, A., Manyakov, N., Chumerin, N.,
Vanderperren, K., Van Huffel, S., and Van Hulle, M.
(2011). Steady state visual evoked potential (SSVEP)-
based brain spelling system with synchronous and
asynchronous typing modes. In 15th Nordic-Baltic
Conference on Biomedical Engineering and Medi-
cal Physics (NBC 2011), IFMBE Proceedings, vol-
ume 34, pages 164–167.
DECODING SSVEP RESPONSES BASED ON PARAFAC DECOMPOSITION
447