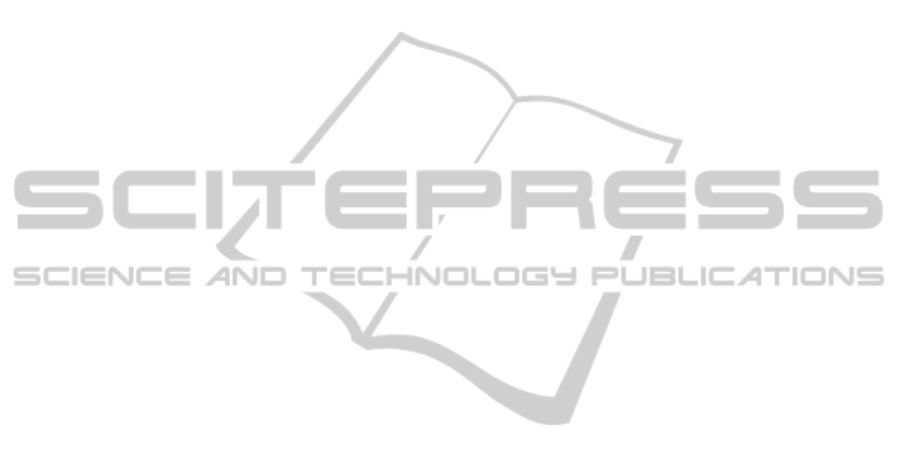
spection of rail wear and widening. At the time of
submitting this paper the project is in a development
stage, so that the results have been obtaining with a
preliminar prototype system. In the further stages of
the project, several improvements are planned and a
more detailed evaluation analysis which will integrate
localisation data to the detection of rail defects.
7 CONCLUSIONS
In this paper we have introduced a new methodology
for the early detection of rail wear and track widening
based on projective geometry concepts and a proba-
bilistic inference framework. Our approach presents
a scenario where its usage in regular services is possi-
ble, with low cost acquisition and processing equip-
ment, which is a competitive advantage over other
manual or contact methodologies. For that purpose,
we propose the use of powerful probabilistic infer-
ence tools that allow us to obtain 3D information
of the magnitudes to be measured from uncomplete
and ambiguous information. In this proposal we use
the overrelaxatedslice sampling technique, which im-
plies a step forward in MCMC methods due to its
reliability, versatility and greater computational effi-
ciency compared to other methods of the literature
that build Markov Chains.
ACKNOWLEDGEMENTS
This work has been partially supported by the Diputa-
cion Foral de Gipuzkoa under project RAILVISION.
REFERENCES
Alippi, C., Casagrande, E., Fumagalli, M., Scotti, F., Piuri,
V., and Valsecchi, L. (2002). An embedded system
methodology for real-time analysis of railways track
profile. In IEEE Technology Conference on Instru-
mentation and Measurement, pages 747–751.
Alippi, C., Casagrande, E., Scotti, F., and Piuri, V. (2000).
Composite real-time image processing for railways
track profile measurement. IEEE Transactions on In-
strumentation and Measurement, 49(3):559–564.
Arulampalam, M. S., Maskell, S., Gordon, N., and Clapp,
T. (2002). A tutorial on particle filters for on-
line nonlinear/non-gaussian bayesian tracking. IEEE
Transactions on Signal Processing, 50(2):174–188.
Barder, F. and Chateau, T. (2008). MCMC particle filter
for real-time visual tracking of vehicles. In IEEE In-
ternational Conference on Intelligent Transportation
Systems, pages 539–544.
Bishop, C. M. (2006). Pattern Recognition and Ma-
chine Learning (Information Science and Statistics).
Springer.
Cannon, D., Edel, K.-O., Grassie, S., and Sawley, K.
(2003). Rail defects: an overview. Fatigue & Fracture
of Engineering Materials & Structures, 26(10):865–
886.
DeMenthon, D. and Davis, L. S. (1995). Model-based ob-
ject pose in 25 lines of code. International Journal of
Computer Vision, pages 123–141.
di Scalea, F. L., Rizzo, P., Coccia, S., Bartoli, I., Fateh, M.,
Viola, E., and Pascale, G. (2005). Non-contact ul-
trasonic inspection of rails and signal processing for
automatic defect detection and classification. Insight
- Non-Destructive Testing and Condition Monitoring,
47(6):346–353.
Gilks, W., Richardson, S., and Spiegelhalter, D. (1996).
Markov Chain Monte Carlo Methods in Practice.
Chapman and Hall/CRC.
Gonzalez, R. and Woods, R. (2002). Digital Image Process-
ing. Prentice Hall.
Khan, Z., Balch, T., and Dellaert, F. (2005). MCMC-based
particle filtering for tracking a variable number of in-
teracting targets. IEEE Transactions on Pattern Anal-
ysis and Machine Intelligence, 27(11):1805–1819.
Neal, R. M. (1998). Suppressing random walks in
markov chain monte carlo using ordered overrelax-
ation. Learning in Graphical Models, pages 205–228.
Neal, R. M. (2003). Slice sampling. Annals of Statistics,
31:705–767.
Nocedal, J. and Wright, S. J. (2006). Numerical Optimiza-
tion. Springer.
VISAPP 2012 - International Conference on Computer Vision Theory and Applications
172