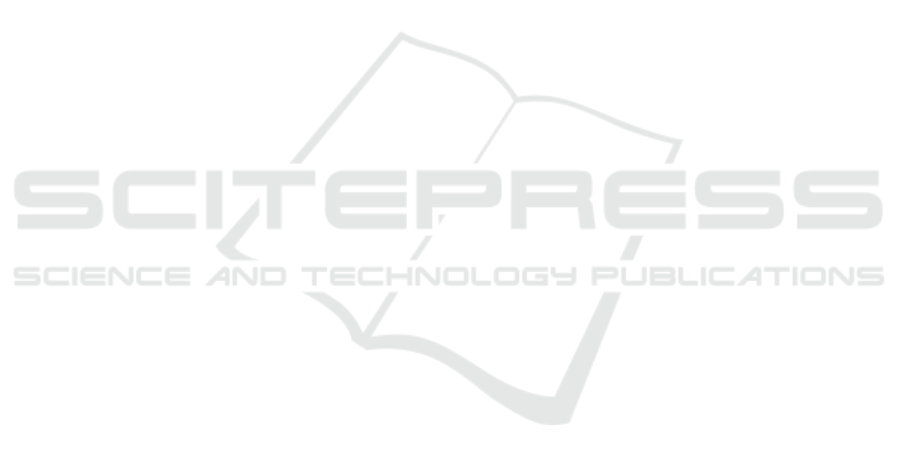
HOW TO DEAL WITH SMALL OPEN READING FRAMES?
Małgorzata Wa
´
nczyk, Paweł Bła
˙
zej, Paweł Mackiewicz and Stanisław Cebrat
Department of Genomics, Faculty of Biotechnology, University of Wrocław, ul. Przybyszewskiego 63/77, Wrocław, Poland
Keywords:
Gene finding, Coding potential, Small ORFs, Short genes.
Abstract:
Current ’classical’ algorithms recognizing protein coding sequences do not work effectively with sequences
of small length. To deal with this problem we have proposed some improvements of the existing gene finders
without any assumed arbitrary threshold. Introduced parameters describe position of tested sequences in the
ranking of all small Open Reading Frames and short protein coding genes found in the analyzed genome. The
sequences can be ranked according to the coding potential calculated by ’standard’ gene prediction algorithms.
As an example, we used two algorithms for gene recognition and tested the set of selected small ORFs which
were selected from prokaryotic genomes using sequence similarity methods. The applied approach enabled to
identify promising sequence that can code for small proteins.
1 INTRODUCTION
The first step in the identification of protein coding
sequences in prokaryotic genomes is searching these
genomes for Open Reading Frame (ORFs), i.e. se-
quences beginning with a start translation codon and
ending at a stop translation codon. There are several
computer annotation tools which are able to evaluate
the coding potential of such sequences (see for re-
views (Azad, 2008), (Majoros, 2007)). For example,
the most common gene finding programs, which are
based on Markov chains, i.e. GeneMark (Borodovsky
and Mcinich, 1993), GeneMark.hmm (Borodovsky
and Lukashin, 1998), Glimmer (Delcher et al., 2007),
and EasyGene (Larsen and Krogh, 2003), recognize a
proper reading frame based on coding potential fac-
tors (a posteriori probabilities) computed for each of
six reading frames. These algorithms work generally
well for long ORFs (e.g. longer than 300 bp). Un-
fortunately, these methods become less reliable for
small Open Reading Frames (smORFs) - see also Fig.
1, Fig. 2 and Fig. 3. Because there are the enor-
mous number of short spurious ORFs found in every
genome, usually ORFs longer than 300 bp are con-
sidered and annotated. It allows to avoid many false
positives.
The output of the gene finding programs depends
also on the model parameters, for example the arbi-
trary threshold assumed on the coding potential level.
As a result of this, a lot of useful information is ’hid-
den’ from a user. For example coding potential for
alternative reading frames and ORFs with the subop-
timal coding probability are usually not given. The
lack of this information makes the gene finders in-
appropriate tool for the detection of smORFs which
usually have very weak coding potential. However,
the capabilities of these programs still can be used to
rank smORFs. Therefore, we have proposed an other
method using the gene finders to verify the coding ca-
pacity of short sequences. Our approach is based on
the measure of coding potential computed for a given
sequence without any assumed arbitrary threshold. In
the paper we have applied two algorithms for gene
recognition and assessed the coding potential of short
ORFs which were collected using other methods by
(Warren et al., 2010).
2 MATERIALS AND METHODS
In the analyses, we included 254 prokaryotic
genomes whose data were downloaded from Gen-
Bank (www.ncbi.nlm.nih.gov). All ORFs with anno-
tated function in these genomes were considered cod-
ing and were used as learning sets in the gene recogni-
tion algorithms. From the genomes we extracted the
set of all small ORFs of the length 30− 300 bp to eval-
uate efficiency of the applied methods. We also tested
the set of short ORFs found in intergenic regions by
(Warren et al., 2010). These frames escaped usually
from recognition by standard gene finding algorithms
but were identified by BLAST searches based on se-
246
Wa
´
nczyk M., Bła
˙
zej P., Mackiewicz P. and Cebrat S..
HOW TO DEAL WITH SMALL OPEN READING FRAMES?.
DOI: 10.5220/0003856202460250
In Proceedings of the International Conference on Bioinformatics Models, Methods and Algorithms (BIOINFORMATICS-2012), pages 246-250
ISBN: 978-989-8425-90-4
Copyright
c
2012 SCITEPRESS (Science and Technology Publications, Lda.)