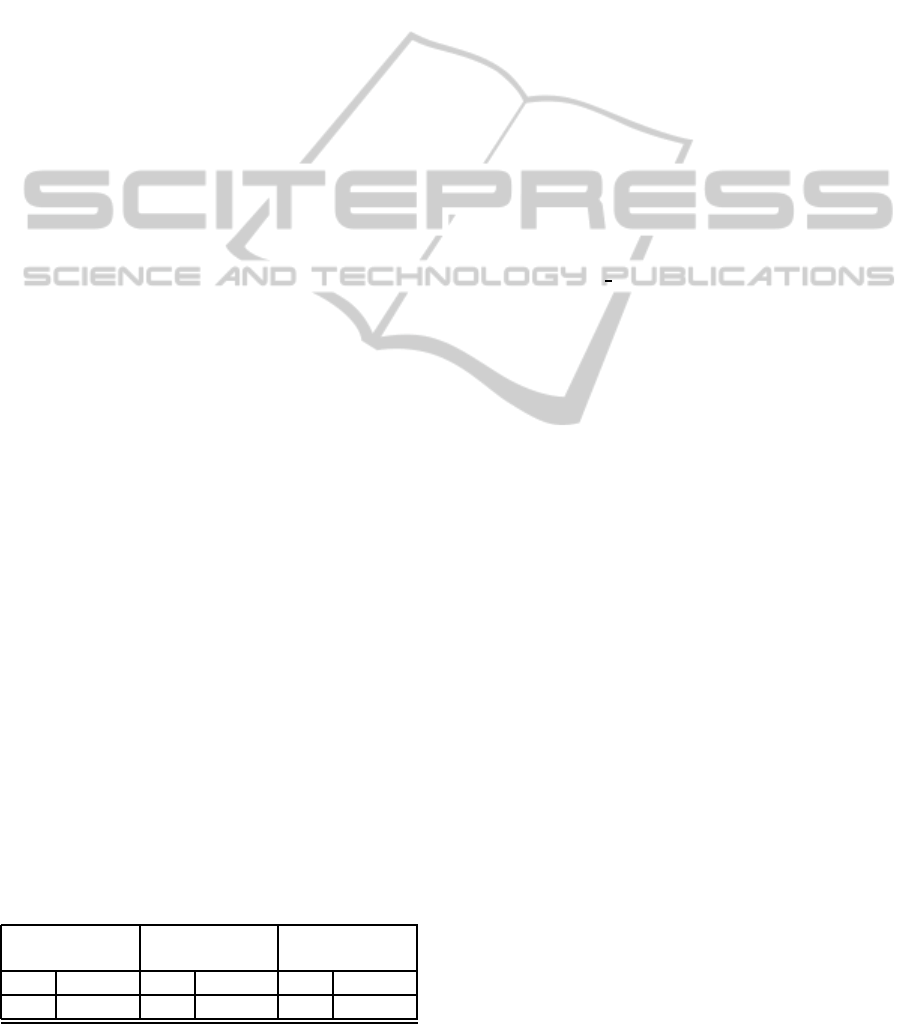
structure modification at a specific level of signif-
icance.
Indeed, we first established a fixed number of re-
gions (i.e., 7), a set of arbitrary thresholds T =
{0.75, 0.76,0.77,0.78, 0.79,0.80} and a set of arbi-
trary rays R = [6]. Then, for each combination of
δ ∈T and K ∈R, we evaluated (for each class of tests)
the number of significant tests rejecting the null hy-
pothesis over the spectra. In tab. 1, we report, for
each class, both the pair (δ,K) employed to detect the
lowest number (i.e., n = 1) of tests rejecting the null,
and the mass-to-charge ranges which identify the re-
jection regions at a 5% significance level.
5 CONCLUSIONS
This study showed the possibility to use the extracted
peptides to separate healthy subjects from tumor pa-
tients and mostly to distinguish non-ccRCC from
RCC. By testing hypotheses on a specific graph prop-
erty (i.e., density), we derived decision procedures
able to provide the clinical modeler with lists of Dal-
ton ranges where it has been detected distinguishing
regions. We point out that, from a clinical perspec-
tive, in order to apply this approach (for example, to
decide the membership group of new subjects), it will
be necessary to compute a correlation matrix (whose
components are given by Eq. 2) over a set of techni-
cal replicates. This will be the most obvious extension
for our next work when new (biological and technical)
samples will be available. Moreover, we can sum-
marize, as follow, some further extensions which we
are immediately interested to: (I) We need to deter-
mine conclusively the identity of the lists of signals
in any differentially expressed region. The theoret-
ical framework of section 3 was employed to detect
spectral signals for their biological importance (for
instance, to suggest potential biomarkers for future
analyses) even their identity is not yet ensured. Identi-
fication of the peptides/proteins, generating these sig-
nals, is a very laborious process implying the analy-
sis of the urine extract with different MS approaches.
Therefore, in order to recognize candidate multiple
biomarkers, for a specific disease, it’s important first
to determine their diagnostic “power” and then to in-
vestigate better their biological role in the disease
Table 1: Mass-to-Charge regions for Control vs. Case.
CVR CVNR RVNR
δ = 0.75, K = 2 δ = 0.75,K = 2 δ = 0.75,K = 2
From To From To From To
1719 2084 1719 2084 4625 5374
mechanisms. (II) The dominant approach to classi-
fier design in clinical studies has been to minimize the
probability of error – see for example, (Dudoit et al.,
2002). Yet it is clear that failing to detect a malignant
tumor has drastically different consequences than er-
roneously flagging a benign tumor. In other words,
classification requirements are often constrained by a
given true positive (type I error) and false positive rate
(type II error) to ensure that the classification error
for the most important class is within a desired limit.
In order, for our procedures to take into account all of
these two requirements, it is necessary to constrain the
type II error. We point out that, here by constraining
only the type I error, we applied a methodology ap-
proach mainly to provide the list of modified regions.
ACKNOWLEDGEMENTS
This work was supported by grants of the Ital-
ian Ministry of Research: PRIN 2006, FIRB 2007
(RBRN07BMCT
11), FAR 2006–2011, and in part
by both the EuroKUP COST Action (BM0702) and
the NEDD project of the “Regione Lombardia”.
REFERENCES
Bosso, N., Chinello, C., Picozzi, S., Gianazza, E., Mainini,
V., Galbusera, C., Raimondo, F., Perego, R., Casel-
lato, S., Rocco, F., Ferrero, S., Bosari, S., Mocarelli,
P., Kienle, M. G., and Magni, F. (2008). Human urine
biomarkers of renal cell carcinoma evaluated by clin-
prot. Proteomics - Clin. App., 2:1036–1046.
Brandes, U. and Erlebach, T., editors (2005). Network Anal-
ysis: Methodological Foundations, volume 3418 of
Lect. Notes in Computer Science. Springer.
Brannon, A. and Rathmell, W. (2010). Renal cell carci-
noma: where will the state-of-the-art lead us? Curr.
Oncol. Rep., 12:193–201.
Drucker, B. (2005). Renal cell carcinoma: current status
and future prospects. Cancer Treat. Rev., 31:536–545.
Dudoit, S., Fridlyand, J., and Speed, T. (2002). Compari-
son of discrimination methods for the classification of
tumors using gene expression data. J. of the American
Stat. Assoc., 97(457):77–87.
Getoor, L. and Taskar, B. (2007). Introduction to Statistical
Relational Learning. The MIT Press.
Latterich, M., Abramovitz, M., and Leyland-Jones, B.
(2008). Proteomics: New technologies and clinical
applications. Eur. Jour. Cancer., 44:2737–2741.
Solassol, J., Jacot, W., Lhermitte, L., Boulle, N., Maude-
londe, T., and Mang, A. (2006). Clinical proteomics
and mass spectrometry profiling for cancer detection.
Expert Rev. Proteomics, 3(3):311–320.
BIOINFORMATICS 2012 - International Conference on Bioinformatics Models, Methods and Algorithms
256