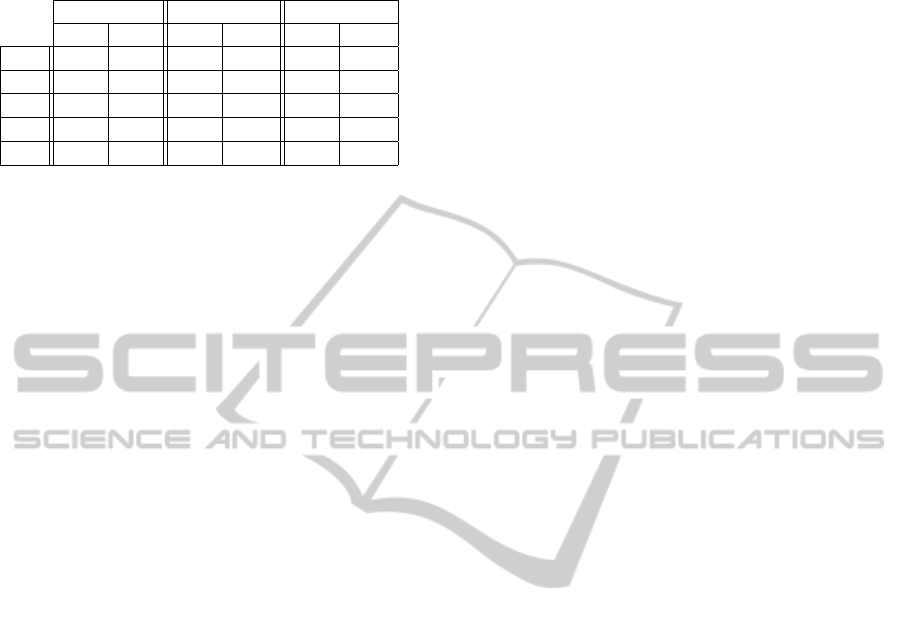
Table 2: Results for the registration of subsequences in an
obstacle avoidance.
REFA3D HOG/HOF SIFT3D
P Fr P Fr P Fr
ss1 99.4 100 98.7 99.5 99.2 100
ss2 91.3 95.6 89.2 90.3 86.7 93.3
ss3 79.1 87.6 67.3 72.3 71.2 81.3
ss4 85.4 92.2 79.6 84.4 81.3 88.4
ss5 94.7 97.3 91.3 91.5 95.1 95.6
5 CONCLUSIONS
We propose in this article a space-time generalization
of our method REFA. To do this, we use the detec-
tor hes-STIP, which has the highest repeatability rate
for this type of analysis. The optimization that we
bring on the limitation of exploration scales (spatial
and temporal). The mask of analysis is also modified
to add the time component in the histograms. The
ellipses are converted into ellipsoids and we use five
levels of description (Figure 2). Adding a temporal
adjustment results a stable three-dimensional explo-
ration of the sequence. To validate this space-time
generalization, we first propose several tests based on
sequences from a real camera and a simulator. The
results show that our approach generally gets the best
precision. We also observe a better stability and a
higher matching rate. In a second step, we study the
registration of subsequences. This type of process is
used to provide space-time informations of the object
(localization, trajectory for example). Our method
performs best for the precision and the rate of reg-
istered images.
Our future prospects is the integration of our ap-
proach REFA3D in intelligent vehicles. Our goal is to
improve again and again the precision of our method,
for the vehicules to be more reliable and secure. An
other prospect is to export our descriptor to three-
dimensional field to use it in medical imaging.
REFERENCES
Bay, H., Tuylelaars, T., and Gool, L. V. (2006). Surf :
Speeded up robust features. European Conference on
Computer Vision, pages 404–417.
Delmas, P. (2011). Gnration active des dplacements d’un
vhicule agricole dans son environnement. PhD thesis,
University Blaise Pascal - Clermont II.
Dollar, P., Rabaud, V., Cottrell, G., and Belongie, S. (2005).
Behavior recognition via sparse spatio-temporal fea-
tures. IEEE International Conference on Computer
Vision.
Grand-brochier, M., Tilmant, C., and Dhome, M. (2011).
Method of extracting interest points based on multi-
scale detector and local e-hog descriptor. Interna-
tional Conference on Computer Vision Theory and
Applications.
Klaser, A., Marszalek, M., and Schmid, C. (2008). A spatio-
temporal descriptor based on 3d-gradients. British
Machine Vision Conference, pages 995–1004.
Laptev, I., Caputo, B., Schuldt, C., and Lindeberg, T.
(2007). Local velocity-adapted motion events for
spatio-temporal recognition. Computer Vision and Im-
age Understanding, 108(3):207–229.
Laptev, I. and Lindeberg, T. (2003). Space-time interest
points. IEEE International Conference on Computer
Vision, 1:432–439.
Laptev, I. and Lindeberg, T. (2006). Local descriptors for
spatio-temporal recognition. Computer and Informa-
tion Science, 3667:91–103.
Lowe, D. (1999). Object recognition from local scale-
invariant features. IEEE International Conference on
Computer Vision, pages 1150–1157.
Lowe, D. (2004). Distinctive image features from scale-
invariant keypoints. International Journal of Com-
puter Vision, 60(2):91–110.
Malartre, F. (2011). Perception intelligente pour la navi-
gation rapide de robots mobiles en environnement na-
turel. PhD thesis, University Blaise Pascal - Clermont
II.
Scovanner, P., Ali, S., and Shah, M. (2007). A 3-
dimensional sift descriptor and its application to ac-
tion recognition. ACM Multimedia.
Wang, H., Ullah, M., Klaser, A., Laptev, I., and Schmid, C.
(2009). Evaluation of local spatio-temporal features
for action recognition. British Machine Vision Con-
ference.
Willems, G., Tuytelaars, T., and Gool, L. V. (2008). An effi-
cient dense and scale-invariant spatio-temporal inter-
est point detector. European Conference on Computer
Vision, 5303(2):650–663.
REFA3D: ROBUST SPATIO-TEMPORAL ANALYSIS OF VIDEO SEQUENCES
357