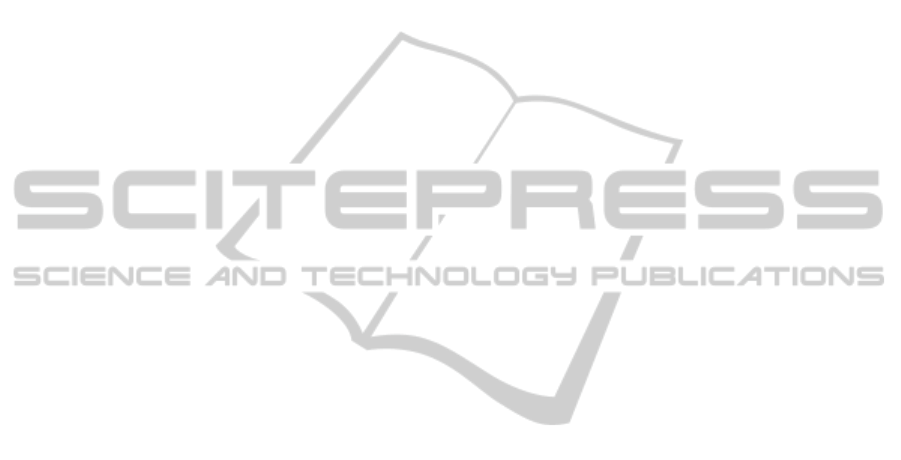
4 CONCLUSIONS AND
PROSPECTS
In this paper, in a first part, we have proposed and pre-
sented different descriptors of the depth map. Follow-
ing of the tests results the Average descriptor provides
performance equivalent to HOG descriptor which is
our reference.
In a second part, we have proposed a change of
the Adaboost algorithm taking account of the algo-
rithmic cost λ
c
for the selection of each weak classi-
fier. This new algorithm (HAB) has been evaluated on
the fusion of a appearance descriptor (HOG) with de-
scriptors of the depth map (CovVar, HOG-depth and
Average). The ROC curve which has a fixed process-
ing time, is superior to the conventional Adaboost ap-
proach. The output of HAB algorithm evaluates Al-
gorithms cost of strong classifier Λ
t
also.
The HAB algorithm could be adapted to the use
of a cascade (Viola and Jones, 2001) by setting a pro-
cessing time of each floor. In addition, the computa-
tional cost of each classifier can be redefined at each
stage in order to favour slow but efficient detectors at
the end of the cascade.
REFERENCES
Begard, J., Allezard, N., and Sayd, P. (2008). Real-time hu-
man detection in urban scenes: Local descriptors and
classifiers selection with adaboost-like algorithms. In
Computer Vision and Pattern Recognition Workshops,
2008. CVPRW ’08. IEEE Computer Society Confer-
ence on, pages 1–8.
Dalal, N. and Triggs, B. (2005). Histograms of oriented gra-
dients for human detection. In Computer Vision and
Pattern Recognition, 2005. CVPR 2005. IEEE Com-
puter Society Conference on, volume 1, pages 886–
893.
Dalal, N., Triggs, B., and Schmid, C. (2006). Human de-
tection using oriented histograms of flow and appear-
ance. In In European Conference on Computer Vision.
Springer.
Enzweiler, M. and Gavrila, D. (2011). A multilevel
mixture-of-experts framework for pedestrian classifi-
cation. Image Processing, 20(10):2967–2979.
Ess, A., Leibe, B., Schindler, K., , and van Gool, L. (2008).
A mobile vision system for robust multi-person track-
ing. In IEEE Conference on Computer Vision and Pat-
tern Recognition (CVPR’08). IEEE Press.
Freund, Y. and Schapire, R. E. (1999). A short introduction
to boosting. Journal of Japanese Society for Artificial
Intelligence, 14(5):771–780.
Friedman, J., Hastie, T., and Tibshirani, R. (1998). Addi-
tive logistic regression: a statistical view of boosting.
Statistics, Stanford University Technical Report.
Tuzel, O., Porikli, F., and Meer, P. (2008). Pedestrian
detection via classification on riemannian manifolds.
Transactions on Pattern Analysis and Machine Intel-
ligence, 30:1713–1727.
Viola, P. and Jones, M. (2001). Rapid object detection using
a boosted cascade of simple features. Computer Vision
and Pattern Recognition.
Walk, S., Schindler, K., and Schiele, B. (2010). Disparity
statistics for pedestrian detection: Combining appear-
ance, motion and stereo. ECCV, pages 182–195.
Wojek, C., Walk, S., and Schiele, B. (2009). Multi-cue on-
board pedestrian detection. In Computer Vision and
Pattern Recognition, 2009. CVPR 2009. IEEE Con-
ference on, pages 794–801.
Yoa, J. and Odobez, J. (2008). Fast human detection from
videos using covariance features. In 8th European
Conference on Computer Vision Visual Surveillance
workshop, Marseille.
VISAPP 2012 - International Conference on Computer Vision Theory and Applications
546