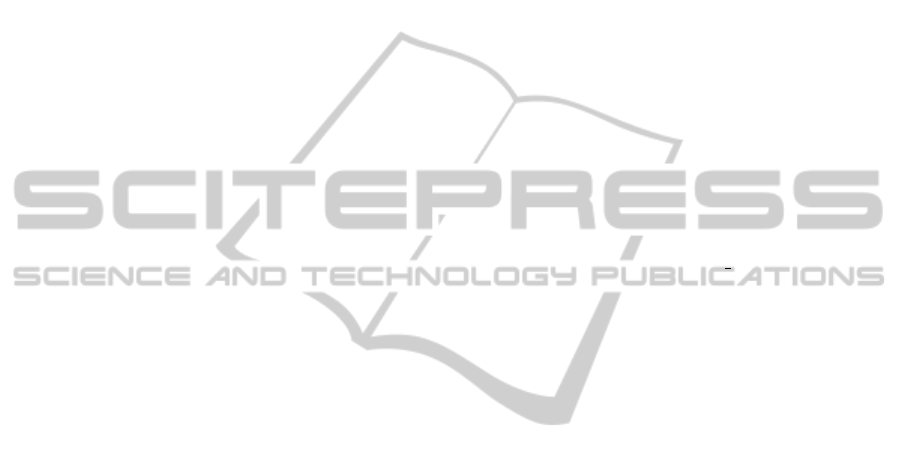
5 CONCLUSIONS
Due to the increasing demand for high resolution
images and sensor limitations, super-resolution tech-
niques are fundamental tools to obtain high resolution
data.
This work proposed a super-resolution technique
based on the fusion of multiple images through resid-
ual compensation using a learning technique and
feature extraction. The comparisons performed in
our experiments showed that the proposed method
achieved higher accuracy when compared to other ap-
proaches available in the literature.
ACKNOWLEDGEMENTS
The authors are grateful to FAPESP, CAPES and
CNPq for the financial support. This research was
partially supported by FAPESP grant 2010/10618-3.
REFERENCES
Baker, S. and Kanade, T. (2002). Limits on Super-
Resolution and How to Break Them. IEEE Transac-
tions on Pattern Analysis and Machine Intelligence,
24(9):1167–1183.
Bascle, B., Blake, A., and Zisserman, A. (1996). Motion
Deblurring and Super-resolution from an Image Se-
quence. In Fourth European Conference on Computer
Vision, pages 573–582. Springer-Verlag.
Borman, S. and Stevenson, R. L. (1998). Super-Resolution
from Image Sequences - A Review. In Midwest Sym-
posium on Circuits and Systems, pages 374–378.
Chang, H., Yeung, D.-Y., and Xiong, Y. (2004). Super-
Resolution through Neighbor Embedding. In IEEE
Conference on Computer Vision and Pattern Recogni-
tion (CVPR), pages 275–282.
Chaudhuri, S. (2001a). Super-Resolution Imaging. The
Springer International Series in Engineering and
Computer Science.
Chaudhuri, S. (2001b). Super-Resolution Imaging. Kluwer
Academic Publishers.
Farsiu, S., Robinson, D., Elad, M., and Milanfar, P. (2004a).
Advances and Challenges in Super-Resolution. Inter-
national Journal of Imaging Systems and Technology,
14:47–57.
Farsiu, S., Robinson, M. D., Elad, M., and Milanfar, P.
(2004b). Fast and Robust Multiframe Super Res-
olution. IEEE Transactions on Image Processing,
13(10):1327–1344.
Gonzalez, R. and Woods, R. (2007). Digital Image Process-
ing. Prentice Hall.
Irani, M. and Peleg, S. (1991). Improving Resolution by Im-
age Registration. Graphical Models and Image Pro-
cessing, 53(3):231–239.
Lin, F. C., Fookes, C. B., Chandran, V., and Sridha-
ran, S. (2005). Investigation into Optical Flow
Super-Resolution for Surveillance Applications. In
APRS Workshop on Digital Image Computing: Pat-
tern Recognition and Imaging for Medical Applica-
tions, Brisbane, Australia.
Liu, X., Song, D., Dong, C., and Li, H. (2008). MAP-Based
Image Super-Resolution Reconstruction. In Proceed-
ings of World Academy of Science, pages 208–211.
Lowe, D. (2004). Distinctive Image Features from Scale-
Invariant Keypoints. International Journal of Com-
puter Vision, 60:91–110.
Lucien, W. (1999). Definitions and Terms of Reference in
Data Fusion. IEEE Transactions on Geosciences and
Remote Sensing, 37(3):1190–1193.
Lucien, W., Ranchin, T., and Mangolini, M. (1997). Fu-
sion of Satellite Images of Different Spatial Res-
olutions: Assessing the Quality of Resulting Im-
ages. Photogrammetric Engineering & Remote Sens-
ing, 63:691–699.
Nagel, H.-H. (2011). Image Sequence
Server. Institut f
¨
ur Algorithmen und
Kognitive Systeme, Universit
¨
at Karlsruhe.
http://i21www.ira.uka.de/image sequences/.
Park, S. C., Park, M. K., and Kang, M. G. (2003).
Super-Resolution Image Reconstruction: A Techni-
cal Overview. IEEE Signal Processing Magazine,
20(3):21–36.
Patti, A. J. and Altunbasak, Y. (2001). Artifact Reduc-
tion for Set Theoretic Super Resolution Image Recon-
struction with Edge Adaptive Constraints and Higher-
Order Interpolants. IEEE Transactions on Image Pro-
cessing, 10(1):179–186.
Schwartz, W., da Silva, R., Davis, L., and Pedrini, H.
(2011). A Novel Feature Descriptor Based on the
Shearlet Transform. In IEEE International Confer-
ence on Image Processing, Brussels, Belgium.
Stark, H. (1988). Theory of Convex Projection and its Ap-
plication to Image Restoration. IEEE International
Symposium on Circuits and Systems, pages 963–964.
Sun, J., Sun, J., Xu, Z., and Shum, H.-Y. (2008). Image
Super-resolution using gradient profile prior. IEEE
Conference on Computer Vision and Pattern Recogni-
tion.
Sun, J., Sun, J., Xu, Z., and Shum, H.-Y. (2010). Gradi-
ent Profile Prior and Its Applications in Image Super-
Resolution and Enhancement. IEEE Transactions on
Image Processing.
Wang, Z., Bovik, A., Sheikh, H., and Simoncelli, E. (2004).
Image Quality Assessment: From Error Visibility to
Structural Similarity. IEEE Transactions on Image
Processing, 13(4):600–612.
Yu, H., Xiang, M., Hua, H., and Chun, Q. (2008). Face
Image Super-Resolution through POCS and Residue
Compensation. IET Conference Publications, pages
494–497.
VISAPP 2012 - International Conference on Computer Vision Theory and Applications
144