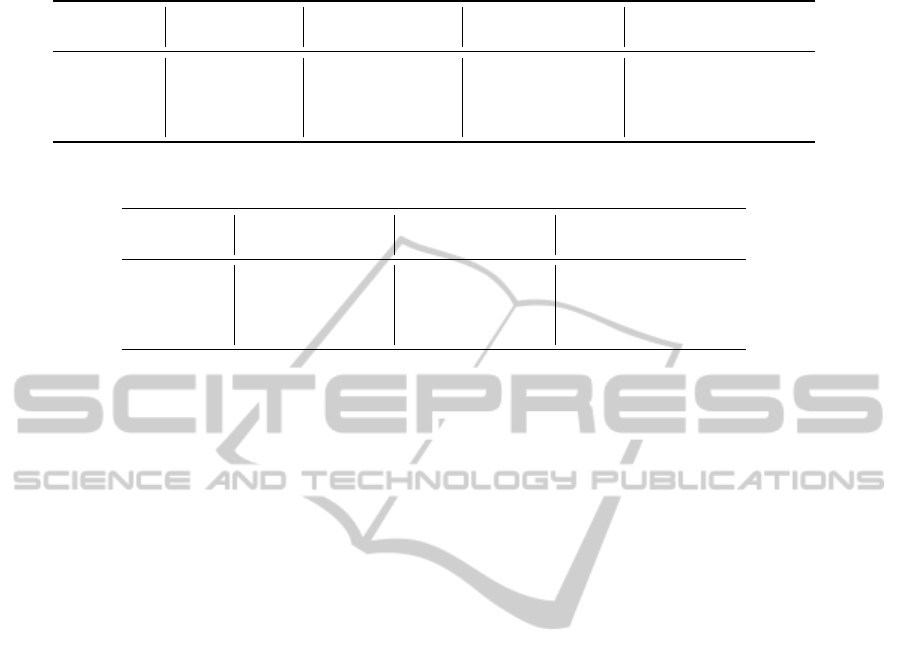
Table 1: Numeric results per method.
Manual Height Threshold Generative model Reconstruction model
In Out In Out In Out In Out
Entire video 14276 10934 4871 3688 10046 6741 11425 8789
Busy 779 1124 109 230 331 461 557 719
Quiet 417 43 273 23 435 56 446 46
Night 22 597 29 237 15 482 45 549
Table 2: Error percentages per method.
Height Threshold Generative model Reconstruction model
In Out In Out In Out
Entire video -65.9% -66.3% -29.6% -38.3% -20.0% -19.6%
Busy -86.0% -79.5% -57.5% -59.0% -28.5% -36.0%
Quiet -34.5% -46.5% 4.3% 30.2% 7.0% 7.0%
Night 31.8% -60.3% -31.8% -19.3% 104.5% -8.0%
to explain but is seen when people bring in baby car-
riages or when children carry helium filled balloons.
Undercounting is most prominent in the na
¨
ıve
method. The reason is that people do not produce
separate blobs when the cut-off is applied. Rais-
ing the cut-off height will not solve this problem un-
til it is raised to above shoulder height, but at then
short people will be overlooked. Moreover, with fore-
ground fattening (Scharstein and Szeliski, 2002) peo-
ple’s blobs may merge even at head height.
Another remarkable result is the over 100% er-
ror margin of people entering in the night sequence
for the reconstruction method. The only people en-
tering in that sequence are personnell bringing in ob-
jects such as trash bins as illustrated in figure 5 which
match the template close enough.
6 CONCLUSIONS
We have shown two novel template based people
counting and localisation methods that work with
range images by stereo cameras. The methods were
tested on a dataset that featured many people in view
at once, a changing background and big changes in
lighting conditions. We found that as the methods
make more use of the available information from the
range image the detection and tracking results im-
prove. The methods run in real-time, making them
suitable for live deployment.
ACKNOWLEDGEMENTS
The research reported in this paper was supported
by the Foundation Innovation Alliance (SIA - Sticht-
ing Innovatie Alliantie) with funding from the Dutch
Ministry of Education, Culture and Science (OCW),
in the framework of the ‘Mens voor de Lens’ project.
REFERENCES
Bahadori, S., Iocchi, L., Leone, G., Nardi, D., and Scoz-
zafava, L. (2007). Real-time people localization and
tracking through fixed stereo vision. Applied Intelli-
gence, 26(2):83–97.
Englebienne, G. and Kr
¨
ose, B. (2010). Fast bayesian peo-
ple detection. In proceedings of the 22nd benelux AI
conference (BNAIC 2010).
Gavrila, D. (2000). Pedestrian detection from a moving ve-
hicle. Computer VisionECCV 2000, pages 37–49.
Nguyen, H., Worring, M., and Van Den Boomgaard, R.
(2001). Occlusion robust adaptive template tracking.
Computer Vision, IEEE International Conference on,
1:678.
Scharstein, D. and Szeliski, R. (2002). A taxonomy and
evaluation of dense two-frame stereo correspondence
algorithms. International journal of computer vision,
47(1):7–42.
Viola, P. and Jones, M. (2001). Rapid object detection us-
ing a boosted cascade of simple features. Computer
Vision and Pattern Recognition, IEEE Computer So-
ciety Conference on, 1:511.
Williams, C. and Titsias, M. (2004). Greedy learning
of multiple objects in images using robust statis-
tics and factorial learning. Neural Computation,
16(5):10391062.
Zhao, T., Nevatia, R., and Wu, B. (2007). Segmentation
and tracking of multiple humans in crowded environ-
ments. IEEE transactions on pattern analysis and ma-
chine intelligence, pages 1198–1211.
VISAPP 2012 - International Conference on Computer Vision Theory and Applications
408