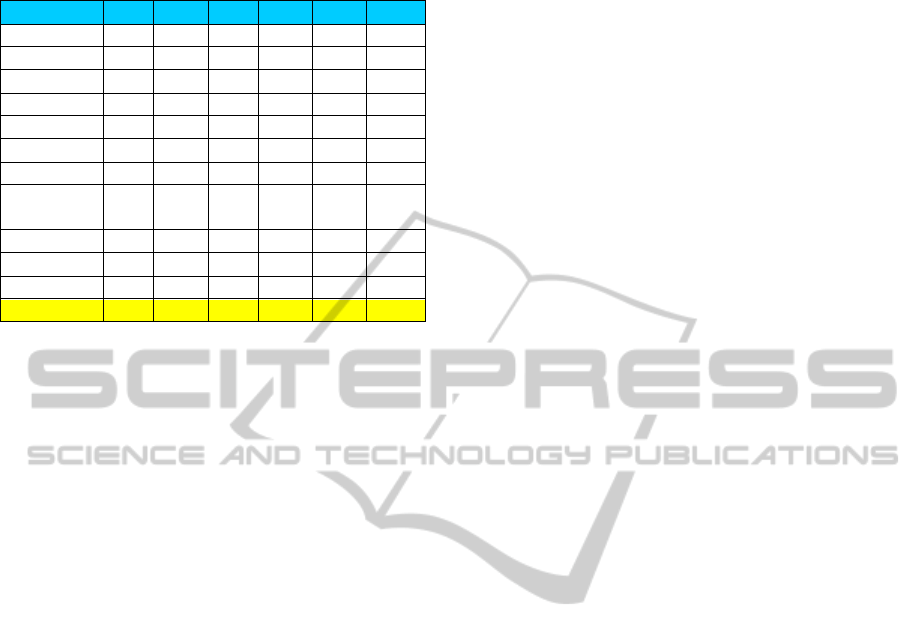
Table 2: Mean Values of correlation coefficients (YCbCr
Space).
INDEX CC R
2
MAE RMS KRCC SROCC
PSNR 0,7980 0,6368 7,7503 9,7064 0,5883 0,7917
MSSIM 0,9071 0,8228 5,1338 6,7813 0,7188 0,9004
VIF 0,9227 0,8513 4,4954 6,2133 0,7574 0,9241
SPSNR (ITTI) 0,7893 0,6230 7,8856 9,8883 0,5822 0,7835
SMSSIM (ITTI) 0,9131 0,8337 5,0010 6,5761 0,7330 0,9100
SVIF (ITTI) 0,9216 0,8494 4,4692 6,2549 0,7574 0,9236
SPSNR (GBVS) 0,7964 0,6343 7,7239 9,7391 0,5885 0,7907
SMSSIM
(GBVS)
0,9120 0,8318 5,0067 6,6060 0,7287 0,9073
SVIF(GBVS) 0,9214 0,8490 4,4496 6,2647 0,7578 0,9236
SPSNR(ACO) 0,8126 0,6603 7,4350 9,3867 0,6058 0,8077
SMSSIM(ACO) 0,9128 0,8332 5,0408 6,6088 0,7406 0,9149
SVIF(ACO) 0,9236 0,8530 4,3895 6,1824 0,7605 0,9251
5 CONCLUSIONS
In this work we presented a strong experimentation
about the comparison between traditional IQA
metrics and visual saliency based ones. All the test
confirmed that IQA saliency based methods
outperform traditional criteria. We also noted that
ACO saliency maps give a stronger support with
respect to the other saliency approaches, ITTI and
GBVS, especially in case of SVIF metric.
Furthermore we pointed out that the SVIF using
ACO saliency map also had the largest GapM of
several noise distributions. It could be very
interesting to stress this kind of experiments with a
lot of more saliency approaches. In this way we will
establish which could be the best Visual Saliency for
Image Quality Assessment.
ACKNOWLEDGEMENTS
The authors wish to acknowledge Alessandro Piero
Filippone for helping us in the implementation and
experimental phases.
REFERENCES
Z. Wang and A.C. Bovik, 2006. Modern Image Quality
Assessment. New York: Morgan & Claypool.
Recommendation, I, 2002. Methodology for the subjective
Assessment of the Quality of television pictures. ITU-
R Rec. BT. 500-11.
Ma, Q. And Zhang, L., 2008. Saliency-based image
quality assessment criterion. Advanced Intelligent
Computing Theories and Applications With Aspects of
Theoretical and Methodological Issues.
Ma, Q. And Zhang, L., 2008. Image Quality Assessment
with visual Attention. 19th ICPR.
Ma, L. and Li, S. And Ngan, K.N., 2010. Visual
Horizontal Effect for Image Quality Assessment.
Signal Processing Letters, IEEE.
I. Acvibas, B. Sankur, and K. Sayood, 2002. Statistical
Evaluation of image quality measures. J. Eletron. Imag.
N. Damera-Venkata, T. D. Kite, W. S. Geisler, B. L.
Evans and A.C. Bovik, 2000. Image quality
assessment based on a degradation model. IEEE
Trans. Image Process.
Wang, Z., Bovik, A.C., Sheikh, H.R., Simoncelli, E.P.
,2004. Image Quality Assessment: from Error visibility
to structural similarity. IEEE Trans. on Image
Processing.
Sheikh, H. R. , Bovik, A. C., 2006. Image Information and
Visual Quality. IEEE Trans. on Image Processing.
Wang, Z., Simoncelli, E., and Bovik, A. C., 2003. Multi-
scale structural similarity for image quality
assessment. Proc IEEE Asilomar Conf. on Signals,
Systems, and Computers, (Asilomar).
Moorthy, A. K. and Bovik, A. C. , 2009. Perceptually
significant spatial pooling techniques for image
quality assessment. Proc. Electronic Imaging.
Itti, L. and Koch, C. And Niebur, E., 1998. A model of
saliency-based visual attention for rapid scene
analysis. Pattern Analysis and Machine Intelligence,
IEEE Transactions on.
Harel, J. and Koch, C and Perona, P., 2007. Grahp-based
visual saliency. Advances in neural information
processing systems.
Ma, L. and Tian, J. And Yu, W., 2010. Visual Saliency
Detection in Image using Ant Colony Optimisation
and local phase coherence. Electronic Letters.
Frintrop, S. and Rome, E. And Christensen, H.I., 2010.
Computational visual attention systems and their
cognitive foundations: A survey. ACM Transactions
on Applied Perception (TAP).
Marchesotti, L. and Cifarelli, C. And Csurka, G., 2009. A
framework for visual saliency detection with
applications to image thumbnailing. 12th ICCV.
LIVE Database http://live.ece.utexas.edu/research/quality
Torralba Database http://people.csail.mit.edu/tjudd/ Where
PeopleLook/
Nagelkerke, N.J.D., 1991. A note on a general definition
of the coefficient of determination. Biometrika, (78).
Prokhorov, A.V., 2001. Kendall coefficient of rank
correlation. Hazewinkel, Michiel, Encyclopaedia of
Mathematics, Springer.
Brunnstrom, K. and Hands, D. and Speranza, F. and
Webster, A., 2009. VQeg validation and ITU
standardization of objective perceptual video quality
metrics. Signal Processing Magazine, IEEE.
Wilmott, C.J. and Matsuura, K., 2005. Advantages of the
mean absolute error (MAE) over the root mean square
error (RMSE) in assessing average model
performance. Climate Research.
Paul G. Gottschalk, John R. Dunn, 2005. The five-parameter
logistic: A characterization and comparison with the four-
parameter logistic. Analytical Bicheminstry.
IMAGE QUALITY ASSESSMENT BY SALIENCY MAPS
483