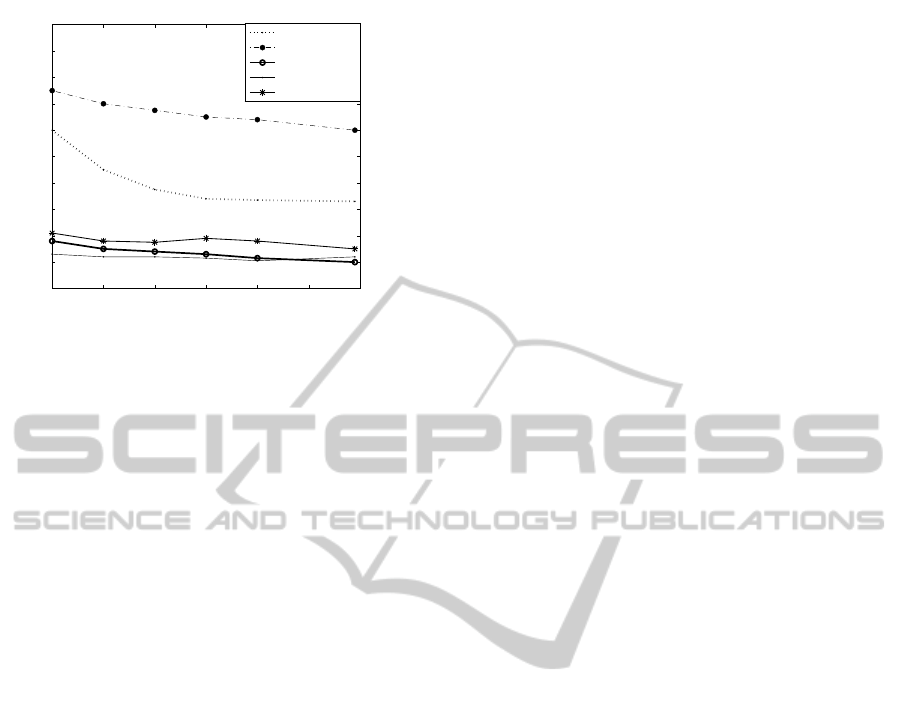
1000 2000 3000 4000 5000 6000 7000
0
0.02
0.04
0.06
0.08
0.1
0.12
0.14
0.16
0.18
0.2
Number of vertices
Weight thresholds
Average color
Edge histogram
Focus
LBP
Dominant color
Figure 7: Critical weight values in the graph of different
descriptors.
small dense components.
Our work presents the possibility of finding the
optimal threshold, depending on the selected descrip-
tor. This way we are also able to evaluate the ’quality’
of a descriptor. The lower the critical weight value is,
the smaller the chance of finding relevant dense clus-
ter cores.
6 CONCLUSIONS AND FUTURE
WORK
This paper presents the first steps towards an auto-
matic feature selection framework, investigating de-
scriptor behaviour based on the analysis of random
geometric graphs structures built from real data and
by using element distances based on several descrip-
tors and their distance / difference distributions along
with the generic behaviour of such graph types dur-
ing the appearance of the giant component. Our next
goal is to produce a descriptor evaluation framework
which analyses graph-connectednessweighted by dif-
ference distributions and their relation to the thresh-
olds associated to the estimated appearances of the
giant component, and rank descriptors (and combina-
tions of descriptors) based on these properties. His-
tograms of such distances combined with graph anal-
ysis based on random graph theory can provide a solid
foundation for image and video feature selection.
ACKNOWLEDGEMENTS
This work has been partially supported by Hungarian
Scientific Research Fund grants 83438 and 80352.
REFERENCES
Candes, E., Demanet, L., Donoho, D., and Ying, L. (2006).
Fast discrete curvelet transforms. Multiscale Model-
ing and Simulation, 5(3):861–899.
Chapelle, O., Vapnik, V., Bousquet, O., and Mukherjee,
S. (2002). Choosing multiple parameters for support
vector machines. Machine Learning, 46(1):131–159.
Comaniciu, D. and Meer, P. (2002). Mean shift: A ro-
bust approach toward feature space analysis. IEEE
Trans. on Pattern Analysis and Machine Intelligence,
24(5):603–619.
Erdos, P. and Renyi, A. (1960). On the evolution of random
graphs. Pbulication of the Mathematical Institute of
the Hungarian Academy of Sciences.
Guldogan, E. and Gabbouj, M. (2008). Feature selection
for content-based image retrieval. Signal, Image and
Video Processing, 2(3):241–250.
Kov´acs, L. and Szir´anyi, T. (2007). Focus area extraction
by blind deconvolution for defining regions of inter-
est. IEEE Tr. on Pattern Analysis and Machine Intel-
ligence, 29(6):1080–1085.
Manjunath, B. S., Ohm, J. R., Vasudevan, V. V., and Ya-
mada, A. (2001). Color and texture descriptors. IEEE
Trans. on Circuits and Systems for Video Technology,
2(6):703–715.
Morris, M. and Kender, J. (2009). Sort-merge feature selec-
tion and fusion methods for classification of unstruc-
tured video. In Proc. of IEEE international conference
on Multimedia and Expo, pages 578–581.
Ojala, T. and Pietikainen, M. (2002). Multiresolution gray-
scale and rotation invariant texture classification with
local binary patterns. IEEE Trans. on attern Analysis
and Machine Intelligence, 24(7).
Penrose, M. (2003). Random Geometric Graphs. Oxford
University Press.
Setia, L. and Burkhardt, H. (2006). Feature selection for
automatic image annotation. In Proc. of 28th Pat-
tern Recognition Symposium of the German Associ-
ation for Pattern Recognition. Springer.
Shen, Y., Lu, H., and Xue, X. (2007). A semi-automatic
feature selecting method for sports video highlight an-
notation. In Proc. of 9th Intl. Conference on Advances
in visual information systems, pages 38–48.
Sun, Y., Todorovic, S., and Goodison, S. (2010). Local
learning based feature selection for high dimensional
data analysis. IEEE Trans. on Pattern Analysis and
Machine Intelligence, 32(9):1610–1626.
Zhang, W., Men, S., Xu, L., and Xu, B. (2010). Feature
distribution based quick image retrieval. In Proc. of
Web Information Systems and Applications Confer-
ence, pages 23–28.
VISAPP 2012 - International Conference on Computer Vision Theory and Applications
380