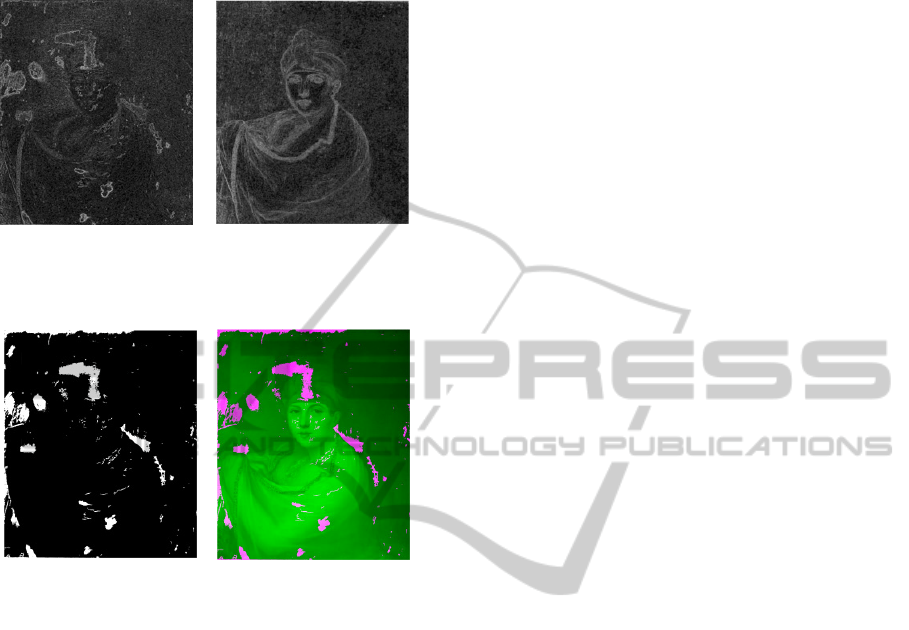
applied to eight pairs of UV and visible images of
fine-art paintings.
(
a)
(b)
Figure 10: Visualized local values of conditional entropies
(|)
UV
(a) and
(|)
VU
(b).
(a)
(b)
Figure 11: The resultant mask of the repainting areas (a)
and the mask combined with the photograph of the
painting (b).
5 CONCLUSIONS
The combined technique for detecting objects in
multimodal images based on specific object
detectors and image difference measure is presented.
Two information-theoretical measures of image
difference are proposed. The conditions of
applicability of these measures for detecting
artefacts in multimodal images are formulated. The
computing experiment has shown the efficiency of
the proposed measures. The combined technique is
successfully applied for detecting repainting and
retouching areas of fine-art paintings. The objects
having grayscale relief similar to the relief of the
repainting area are localized in UV image thought
the instrumentality of specific detectors.
Subsequently, the “true” objects are selected using
considered information-theoretical difference
measure. The proposed technique requires
segmentation of only one of the analyzed images,
unlike the technique described in (Heitz, 1990). The
future research will be aimed at the development
automated procedures for choosing window size and
number of quantization levels for estimating
conditional entropies.
ACKNOWLEDGEMENTS
This work was supported by the Russian Foundation
for Basic Research, grant No 09-07-00368.
REFERENCES
Daly S., 1993. The visible differences predictor: an
algorithm for the assessment of image fidelity. Digital
images and human vision, MIT Press, Cambridge.
Escolano, F., Suau, P., Bonev, B., 2009. Information
Theory in Computer Vision and Pattern Recognition.
London: Springer Verlag.
Heitz, F., Maitre, H. de Couessin, C., 1990. Event
Detection in Multisource Imaging: Application to Fine
Arts Painting Analysis. IEEE trans. on acoustics,
speech, and signal processin, 38(1), 695-704.
Kammerer, P., Hanbury, A., Zolda, E., 2004. A
Visualization Tool for Comparing Paintings and Their
Underdrawings. In EVA’2004, Conference on
Electronic Imaging & the Visual Arts, 148–153.
Kirsh, A., and Levenson, R. S., 2000. Seeing through
paintings: Physical examination in art historical
studies. Yale U. Press, New Haven, CT.
Minakhin, V., Murashov, D., Davidov, Yu., Dimentman,
D., 2009. Compensation for Local Defects in an Image
Created Using a Triple-Color Photo Technique.
Pattern Recognition and Image Analysis: Advances in
Mathematical Theory and Applications. MAIK
"Nauka/Interperiodica". 19, 1. 137 – 158.
Niblack, W., 1986. An Introduction to Digital Image
Processing. Prentice Hall, Englewood Cliffs, NJ.
Petrovic, V., Xydeas, C., 2004. Evaluation of Image
Fusion Performance with Visible Differences.
ECCV'2004, LNCS, 3023, 380-391.
Rajwade, A., Banerjee, A., Rangarajan, A., 2006.
Continuous Image Representations Avoid the
Histogram Binning Problem in Mutual Information
Based Image Registration, Proc. of IEEE International
Symposium on Biomedical Imaging (ISBI). 840-843.
Rockinger, O., Fechner, T., 1998. Pixel-Level Image
Fusion: The Case of Image Sequences. SPIE Proc.
Signal Processing, Sensor Fusion, and Target
Recognition VII, Ivan Kadar, Editor, 3374, 378-388.
Soille,;P., 2004. Morphological Image Analysis:
Principles and Applications, Springer-Verlag. Berlin.
Viola, P., 1995. Alignment by Maximization of Mutual
Information. PhD Thesis, MIT.
Zhang, J., Rangarajan, A. 2004. Affine image registration
using a new information metric. In: IEEE Computer
Vision and Pattern Recognition (CVPR), 1, 848-855.
VISAPP 2012 - International Conference on Computer Vision Theory and Applications
732