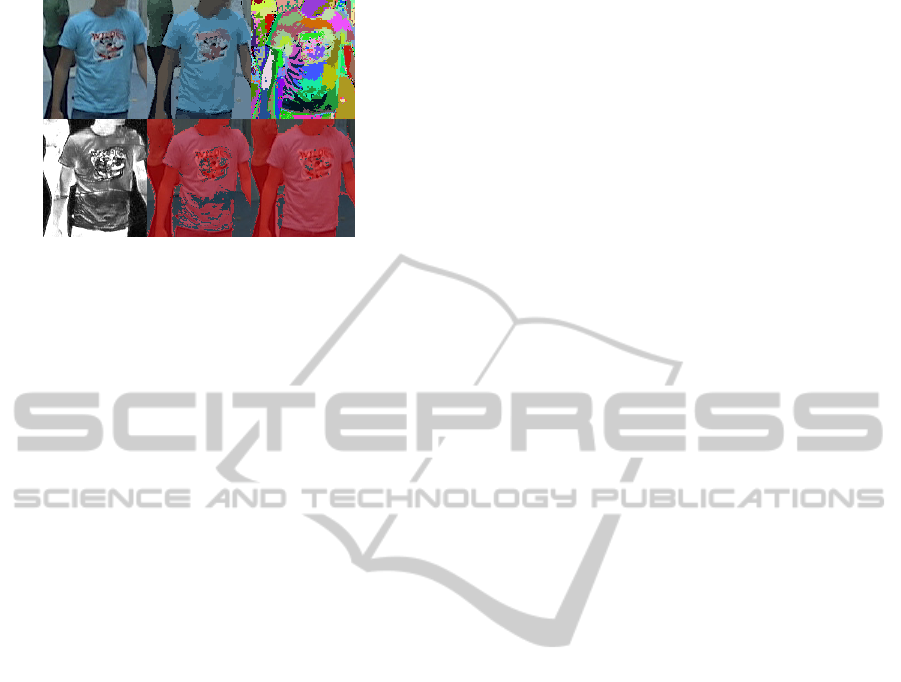
Figure 6: Grouping robustness: Top Line: Image source,
mode mean color, mode identification; Bottom line: Prob-
ability map from ”Stauffer & Grimson”, corresponding de-
tection results and detection result with our method.
tions are difficult due to direct outdoor lighting chang-
ing during the sequence and a light flicker. The results
are plotted in Figure 3 and Figure 2. The proposed
method outperforms the pixel-based method.
4.3 Detailed Analysis of some
Characteristics
Beyond these general performance, it is relevant to
focus on some algorithm behaviors:
Target Decomposition. Figure 4 highlights the gen-
erated modes decomposition on a person. Each
mode is represented by a random color for bet-
ter visualisation. It can be observed that the tar-
get and the background are clearly segmented
and also that the different body members are seg-
mented, which could be useful for a higher-level
analysis.
Shadow Separation. As many algorithms, the pro-
posed method classifies some shadows as mov-
ing objects. However, by analyzing the compo-
sition of the modes (Figure 5), we can notice
that shadows are segmented in dedicated modes.
Treatments for shadows removal are simplified as
group of pixels can directly be studied and com-
pared to old background.
Grouping Robustness. To illustrate the grouping
interest, it is relevant to focus on difficult cases
such as the Tee-shirt whose color is close to the
background color (Figure 6). The Stauffer and
Grimson method generates many misdetections.
The mode-based decision of the proposed algo-
rithm alleviates these problems.
Object Tracking. Finally, assuming that the move-
ment of objects from one image to the following is
relatively small, the corresponding modes follow
the object. If the object movement is more im-
portant, the ”old” component will disappear and a
corresponding new component will be automati-
cally created: the segmentation and labeling pro-
cess remain effective.
5 CONCLUSIONS
Conventional approaches typically performes a local
modeling independently on each pixel. Using blocks
of pixels of pre-determined shape and size to estimate
a descriptor often leads to more robustness, but the
spatial precision is then deteriorated. The proposed
approach provides a solution to overcome these limi-
tations by combining the accuracy of the pixel infor-
mation with the robustness of a decision made on a
set of coherent pixels. The experimental results pre-
sented emphasized that the proposed approach im-
proves performances, with respect to both Stauffer
and Grimson method and state-of-art SGMM. In par-
ticular, thanks to the spatial consistency, this approach
remains very stable in noisy and dynamic conditions.
REFERENCES
Dickinson, P., Hunter, A., and Appiah, K. (2009). A spa-
tially distributed model for foreground segmentation.
Image and vision computing.
Gallego, J., Pardas, M., and Haro, G. (2009). Bayesian
foreground segmentation and tracking using pixel-
wise background model and region based foreground
model. In Image Processing (ICIP).
Stauffer, C. and Grimson, W. (1999). Adaptive background
mixture models for real-time tracking. In CVPR.
Wang, H., Suter, D., Schindler, K., and Shen, C. (2007).
Adaptive object tracking based on an effective appear-
ance filter. IEEE transactions on pattern analysis and
machine intelligence.
Yu, T., Zhang, C., Cohen, M., Rui, Y., and Wu, Y. (2007).
Monocular video foreground/background segmenta-
tion by tracking spatial-color gaussian mixture mod-
els. In Motion and Video Computing.
VISAPP 2012 - International Conference on Computer Vision Theory and Applications
508