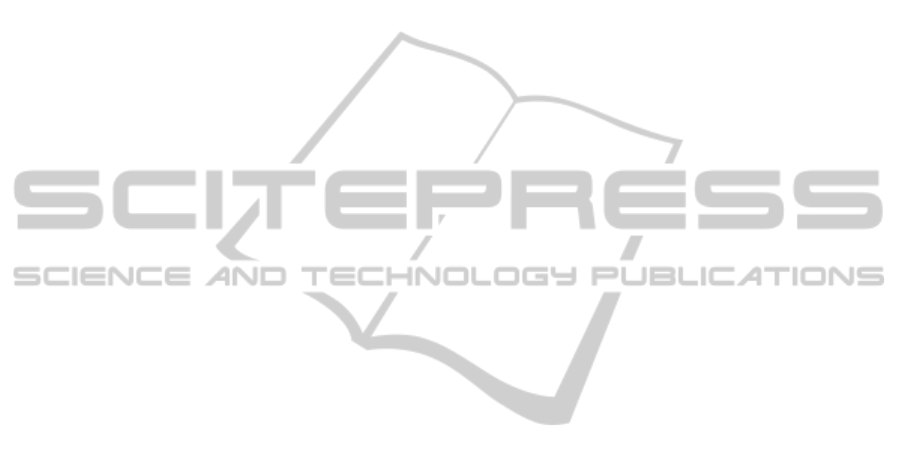
classification accuracy using only training set which
didn’t divide training and test sets. An average
accuracy of classification is necessary for repeated
sub-sampling validation using training and test sets
as the choice of training and test sets can affect the
results. Therefore, we will perform the average
classification in further analysis.
LDA and SOM had the lowest accuracy in
emotion recognition. We think that this result in
variability of physiological signals. The more or less
unique and person-independent physiological
response among different emotions may fall off the
recognition rate with the number of emotion
categories (Kim, Bang, Kim, 2004). These
uncertainties could be an important cause that
deteriorated the recognition ratio and troubled the
model selection of the LDA or SOM. Also, it is
possible that result of LDA which is one of the
linear models or SOM didn’t perform well because
our physiological signals didn’t linear variables and
the extracted features didn’t linearly separable and
large variability between the features used. To
overcome this, we needed performance of some
normalization of features being able to reduce large
variability.
Nevertheless, our results led to better chance to
recognize human emotions and to identify the
optimal emotion classification algorithm by using
physiological signals. This will be able to apply to
the realization of emotional interaction between man
and machine and play an important role in several
applications, e.g., the human-friendly personal robot
or other devices.
ACKNOWLEDGEMENTS
This research was supported by the Converging
Research Center Program funded by the Ministry of
Education, Science and Technology (No.
2011K000655 and 2011K000658).
REFERENCES
Wagner, J., Kim, J., Andre, E., 2005. From physiological
signals to emotions: Implementing and comparing
selected methods for feature extraction and
classification, IEEE International Conference on
Multimedia and Expo, Amsterdam, pp. 940-943.
Sebe, N., Cohen, I., Huang, T. S., 2005. Multimodal
emotion recognition, in Handbook of Pattern
Recognition and Computer Vision, Amsterdam:
Publications of the Universiteit van Amsterdam, pp. 1-23.
Picard, R. W., Vyzas, E., Healey J., 2001. Toward
machine emotional intelligence: Analysis of
affective physiological state, IEEE Transaction on
Pattern Analysis and Machine Intelligence, vol. 23,
pp. 1175-1191.
Cowie, R., Douglas-Cowie, E., Tsapatsoulis, N., Votsis,
G., Kollias, S., Fellenz, W., Taylor, J. G., 2001.
Emotion recognition in human computer interaction,
IEEE Signal Processing Magazine, Vol. 18, pp. 32-80.
Haag, A., Goronzy, S., Schaich, P., Williams, J., 2004.
Emotion recognition using bio-sensors: First steps
towards an automatic system, Affective Dialogue
Systems, vol. 3068, pp. 36-48.
Healey, J. A., 2000. Wearable and automotive systems for
affect recognition from physiology, Doctor of
Philosophy, Massachusetts Institute of Technology,
Cambridge, MA.
Nasoz, F., Alvarez, K., Lisetti, C. L., Finkelstein, N., 2003.
Emotion recognition from physiological signals for
user modelling of affect, International Journal of
Cognition, Technology and Work-Special Issue on
Presence, Vol. 6, pp. 1-8.
Drummond, P. D., Quah, S. H., 2001. The effect of
expressing anger on cardiovascular reactivity and
facial blood flow in Chinese and Caucasians,
Psychophysiology, vol. 38, pp. 190-196.
Calvo, R., Brown, I., Scheding, S., 2009. Effect of
experimental factors on the recognition of affective
mental states through physiological measures, AI
2009: Advances in Artificial Intelligence, vol. 5866,
pp. 62-70.
Kim, K. H., Bang, S. W., Kim, S. R., 2004. Emotion
recognition system using short-term monitoring of
physiological signals, Medical & Biological
Engineering & Computing, vol. 42, pp.419-427.
Takahashi, K., 2004. Remarks on emotion recognition
from bio-potential signals, 2nd International
Conference on Autonomous Robots and Agents,
Palmerston North, pp. 186-191.
EMOTION CLASSIFICATION BASED ON PHYSIOLOGICAL RESPONSES INDUCED BY NEGATIVE EMOTIONS
- Discrimination of Negative Emotions by Machine Learning Algorithms
457