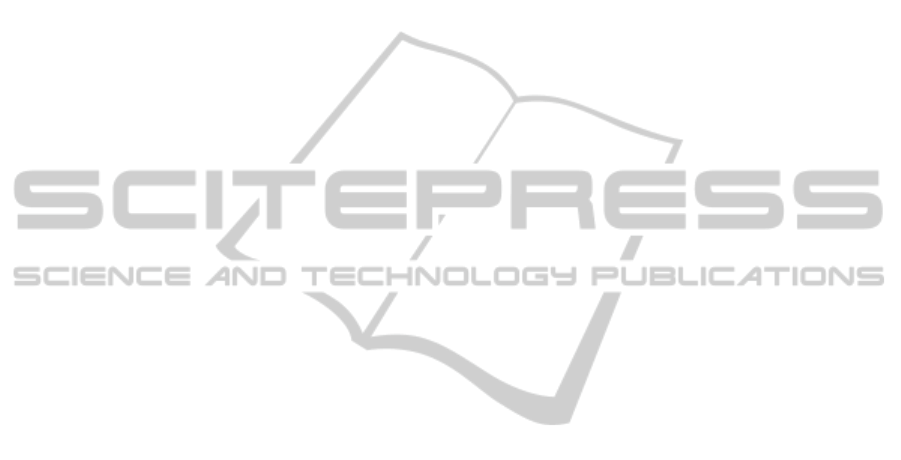
work, we will identify the data quality requirements
of a ProHTA simulation study in detail. Also, we will
study how to control and improve data quality by us-
ing stored knowledge. Additionally, we will refine
our approach to store conceptual models and to utilize
them to annotate stored datasets. Finally, the query
language for statistical simulation input data will be
improved.
ACKNOWLEDGEMENTS
This project is supported by the German Federal Min-
istry of Education and Research (BMBF), project
grant No. 01EX1013B.
REFERENCES
Ainsworth, J. D., Carruthers, E., Couch, P., Green, N.,
O’Flaherty, M., Sperrin, M., Williams, R., Asghar,
Z., Capewell, S., and Buchan, I. E. (2011). Impact:
A generic tool for modelling and simulating public
health policy. Methods of Information in Medicine,
5:454–463.
Batini, C., Cappiello, C., Francalanci, C., and Maurino, A.
(2009). Methodologies for data quality assessment
and improvement. ACM Comput. Surv., 41:16:1–
16:52.
Bengtsson, N., Shao, G., Johansson, B., Lee, Y., Leong, S.,
Skoogh, A., and Mclean, C. (2009). Input data man-
agement methodology for discrete event simulation.
In Winter Simulation Conference (WSC), Proceedings
of the 2009, pages 1335 –1344.
Boulonne, A., Johansson, B., Skoogh, A., and Aufenanger,
M. (2010). Simulation data architecture for sustain-
able development. In Proceedings of the 2010 Winter
Simulation Conference.
Cheng, R. C. H. and Holland, W. (2004). Calculation
of confidence intervals for simulation output. ACM
Trans. Model. Comput. Simul., 14:344–362.
Cyganiak, R., Reynolds, D., and Tennison, J. (2010). The
rdf data cube vocabulary. http://publishing-statistical-
data.googlecode.com/svn/trunk/specs/src/main/html/
cube.html.
F
¨
urber, C. and Hepp, M. (2010). Using semantic web re-
sources for data quality management. In Proceedings
of the 17th international conference on Knowledge en-
gineering and management by the masses, EKAW’10,
pages 211–225, Berlin, Heidelberg. Springer-Verlag.
Gowri, K. (2001). Enerxml - a schema for representing en-
ergy simulation data. In Proceedings of the Seventh
International IBPSA Conference.
Hausenblas, M., Halb, W., Raimond, Y., Feigenbaum, L.,
and Ayers, D. (2009). Scovo: Using statistics on the
web of data. In The Semantic Web: Research and Ap-
plications, volume 5554 of Lecture Notes in Computer
Science, pages 708–722. Springer Berlin / Heidelberg.
Kurze, C., Gluchowski, P., and Bohringer, M. (2010). To-
wards an ontology of multidimensional data structures
for analytical purposes. In System Sciences (HICSS),
2010 43rd Hawaii International Conference on, pages
1 –10.
Lassila, O., Swick, R. R., Wide, W., and Consor-
tium, W. (1999). Resource description frame-
work (rdf) model and syntax specification.
http://www.w3.org/TR/1999/REC-rdf-syntax-
19990222.
Lenz, H.-J. and Shoshani, A. (1997). Summarizability in
olap and statistical data bases. In Scientific and Sta-
tistical Database Management, 1997. Proceedings.,
Ninth International Conference on, pages 132 –143.
Lenz, R., Elstner, T., Siegele, H., and Kuhn, K. A. (2002).
A practical approach to process support in health in-
formation systems. Journal of the American Medical
Informatics Association, 9(6):571–585.
Nadkarni, P. M., Marenco, L., Chen, R., Skoufos, E., Shep-
herd, G., and Miller, P. (1999). Organization of het-
erogeneous scientific data using the eav/cr represen-
tation. Journal of the American Medical Informatics
Association, 6(6):478–493.
Niemi, T. and Niinim
¨
aki, M. (2010). Ontologies and sum-
marizability in olap. In Proceedings of the 2010 ACM
Symposium on Applied Computing, SAC ’10, pages
1349–1353, New York, NY, USA. ACM.
Niemi, T., Toivonen, S., Niinimaki, M., and Nummenmaa,
J. (2007). Ontologies with semantic web/grid in data
integration for olap. International Journal on Seman-
tic Web and Information Systems (IJSWIS), 3:25–49.
Prud’hommeaux, E. and Seaborne, A. (2008). Sparql query
language for rdf. http://www.w3.org/TR/2008/REC-
rdf-sparql-query-20080115/.
Reimann, P., Reiter, M., Schwarz, H., Karastoyanova, D.,
and Leymann, F. (2011). Simpl - a framework for
accessing external data in simulation workflows. In
Datenbanksysteme fr Business, Technologie und Web.
Robertson, N. and Perera, T. (2002). Automated data collec-
tion for simulation? Simulation Practice and Theory,
9(6-8):349 – 364.
Rogers, J., Simakov, R., Soroush, E., Velikhov, P., Balazin-
ska, M., DeWitt, D., Heath, B., Maier, D., Madden,
S., Patel, J., Stonebraker, M., Zdonik, S., Smirnov, A.,
Knizhnik, K., and Brown, P. G. (2010). Overview of
scidb, large scale array storage, processing and analy-
sis. In Proceedings of the SIGMOD’10.
Skoogh, A., Michaloski, J., and Bengtsson, N. (2010).
Towards continuously updated simulation models:
Combingin automated raw data collection and auto-
mated data processing. In Proceedings of the 2010
Winter Simulation Conference.
Stonebraker, M., Becla, J., DeWitt, D., Lim, K.-T., Maier,
D., Ratzesberger, O., and Zdonik, S. (2009). Require-
ments for science data bases and scidb. In Proceedings
of the CIDR 2009 Conference.
Wang, R. Y. and Strong, D. M. (1996). Beyond accuracy:
what data quality means to data consumers. J. Man-
age. Inf. Syst., 12:5–33.
Zhang, Y., Kersten, M., Ivanova, M., and Nes, N. (2011).
Sciql, bridging the gap between science and relational
dbms. In Proceedings of the IDEAS11.
HEALTHINF 2012 - International Conference on Health Informatics
280