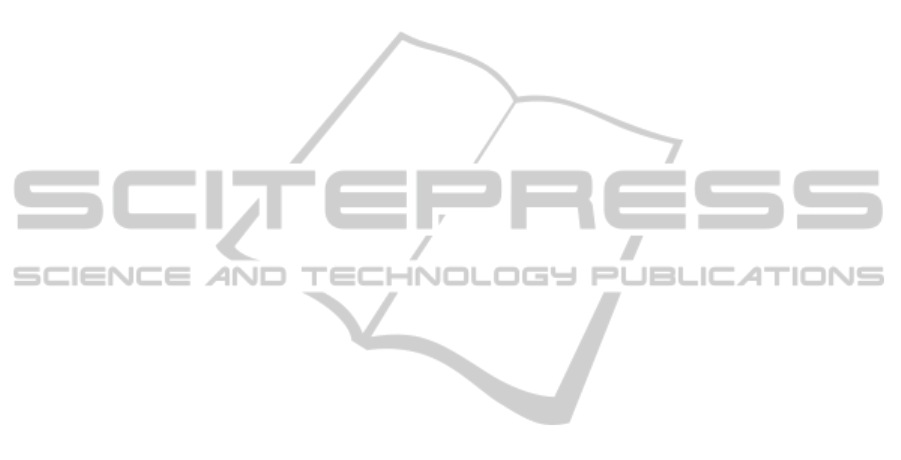
healthcare insurance scheme in the Czech Republic.
Differentiation of positive or negative results as
they relate to quality of service is crucial for
efficient allocation of funds; as such it is (or should
be) of primary interest to the insurance companies.
In order to create and validate an administrative
prediction model, a clinical model, i.e., risk-adjusted
clinical model, should be built first as a “gold
standard”.
For our experiments with risk-adjusted clinical
models we chose hospital mortality in acute
myocardial infarction, because: (1) this indicator is
reliably detectable from available data; (2) treatment
of acute myocardial infarction has a significant
socio-economic impact; (3) prediction of mortality
based on admission findings is the subject of many
research papers, thus we have a good benchmark for
our experimental results; and (4) no outcome-
prediction models are currently used in the Czech
Republic; thus they are a necessary novelty in
quality measurement.
Our work has been motivated by the work
published at the Yale University (Krumholz, H. M.,
et al, 2007).
2 METHODS
2.1 Risk Adjustment
Risk adjustment is a statistical process used to
identify and adjust for variations in patient outcomes
that stem from differences in patient characteristics
(or risk factors) across healthcare organizations in
order to achieve better comparison of patient
outcomes between different organizations and to
improve the interpretability of results. The quality of
healthcare can be measured by several types of
indicators: by mortality, by re-hospitalization rate or
by complication rate. In this part, we will show
reasons and principles of risk adjustment of a
mortality indicator. Standardization of other types of
indicators follows the same principles.
A straightforward comparison of mortality rate
of two different healthcare facilities will not give
objective results as it also depends on the presence
of risk factors at the time of health care encounters.
Patients may experience different outcomes
regardless of the quality of care provided by the
healthcare organization, so comparing patient
outcomes across healthcare organizations without an
appropriate risk adjustment could be misleading. By
adjusting for the risks associated with outcomes of
interest, risk adjustment facilitates a fairer and more
accurate inter-organizational comparison. (CMS,
2005).
There are two essential methods: (1) case
stratification, i.e., decomposition of cases into more
homogeneous sub-groups based, for example, on age
and/or sex grouping, or (2) standardization of results
(indicators), i.e., risk-adjustment. The first method,
however, is disadvantageous both in terms of
statistics (subgroups will often have small numbers
of patients) and in terms of subsequent interpretation
(different subgroups may have different comparative
results and it may not be clear how the provider
should actually be evaluated with respect to the
overall quality in the clinical area).
2.2 Selection of Risk Factors
The requirements for the selection of risk factors
applicable in the standardization process indicators
are as follows: (i) there must be a statistically
significant relationship between risk factor and the
outcome indicator. For example, if the probability of
death from myocardial infarction is related to the
value of blood pressure at the time of admission,
then it is included as a risk factor. The prediction
model should also reflect situations in which certain
combinations of factors have greater impact than
each of the individual factors alone; (ii) the
composition of patients, in terms of risk factors,
must be different in different healthcare facilities.
Otherwise, there is no need to carry out
standardization, although there is a strong
correlation between the indicator and risk factor – all
healthcare facilities are "disadvantaged" in the same
way. In practice, this requirement is usually met, as
healthcare facilities usually have different
distributions of risk factors among their patients; (iii)
each risk factor must clearly reflect the condition of
the patient upon admission to a healthcare facility
and may not be the result of the treatment process
itself; (iv) each risk factor has to be reliably
documented within the available data –
unfortunately, this is a very limiting restriction in
many cases, and especially in administrative data.
It is obvious that the correction of the
measurement bias is never perfect. Even after
standardization, residual bias remains. Residual
distortion can, for example, be caused by risk factors
that are not yet known or that are not reflected in the
data.
2.3 Standardization of Indicators
When standardizing the quality indicators it is first
HEALTHINF 2012 - International Conference on Health Informatics
454