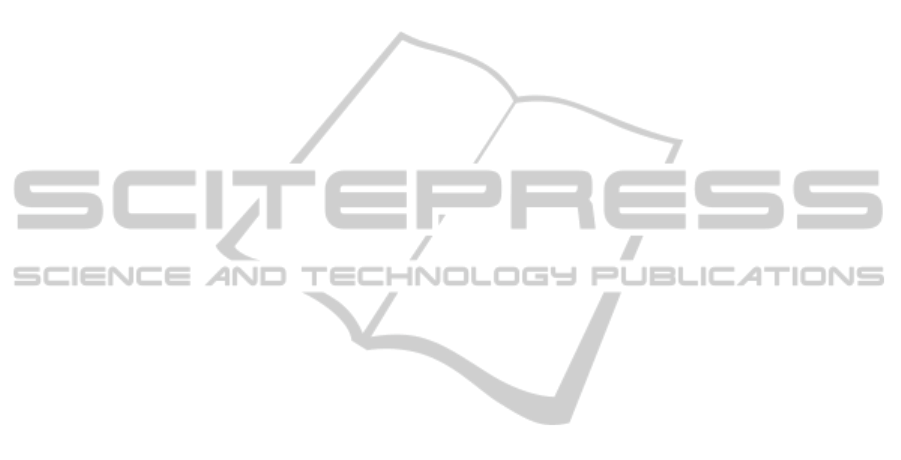
examined patient’s speech signal, may be helpful for
classification of a patient. In general, the speech anal-
ysis of patients with larynx diseases is a difficult task.
Therefore, the obtained results seem to be promising.
In the future, we plan to tune parameters reflect-
ing regularity and deviations in the speech spectrum,
especially by selecting proper regions of interest of
the spectrum and we are going to add some new coef-
ficients characterizing spectrum disturbances as well.
The proposed approach based on analysis of speech
signals in a frequency domain can be a part of a com-
puter tool based on multicriteria decision making pro-
cess. It should be treated as a supplementary element
for other techniques. An important challenge is to
design methods enabling distinction between differ-
ent larynx diseases (for example, laryngeal polyp and
Reinke’s edema). The approach presented in this pa-
per does not enable us to make this distinction.
ACKNOWLEDGEMENTS
This research has been supported by the grant No. N
N516 423938 from the Polish Ministry of Science and
Higher Education.
REFERENCES
Bazan, J. G., Nguyen, H. S., Nguyen, S. H., Synak, P., and
Wroblewski, J. (2000). Rough set algorithms in classi-
fication problem. In Polkowski, L., Tsumoto, S., and
Lin, T. Y., editors, Rough Set Methods and Applica-
tions, pages 49–88. Physica-Verlag, Heidelberg, Ger-
many.
Bazan, J. G. and Szczuka, M. S. (2005). The Rough Set
Exploration System. In Peters, J. and Skowron, A.,
editors, Transactions on Rough Sets III, pages 37–56.
Springer-Verlag, Berlin Heidelberg.
Błajdo, P., Grzymała-Busse, J., Hippe, Z., Knap, M.,
Marek, T., Mroczek, T., and Wrzesie´n, M. (2004). A
suite of machine learning programs for data mining:
chemical applications. In Debska, B. and Fic, G., edi-
tors, Information Systems In Chemistry 2, pages 7–14.
University of Technology Editorial Office, Rzeszow.
Breiman, L., Friedman, J., Olshen, R., and Stone, C. (1993).
Classification and Regression Trees. Chapman &
Hall, Boca Raton.
Cios, K., Pedrycz, W., Swiniarski, R., and Kurgan, L.
(2007). Data mining. A knowledge dis-covery ap-
proach. Springer, New York.
Grzymala-Busse, J. (1997). A new version of the rule induc-
tion system LERS. Fundamenta Informaticae, 31:27–
39.
Hippe, Z. (1997). Machine learning a promising strategy
for business information processing? In Abramowicz,
W., editor, Business Information Systems, pages 603–
622. Academy of Economics Editorial Office, Poznan.
MDVP (2011). Multi-dimensional voice program (MDVP).
http://www.kayelemetrics.com.
Paja, W. and Hippe, Z. (2005). Feasibility studies of
quality of knowledge mined from multiple secondary
sources. I. Implementation of generic operations. In
Klopotek, M., Wierzchon, S., and Trojanowski, K.,
editors, Intelligent Information Processing and Web
Mining, pages 461–465. Springer-Verlag, Berlin Hei-
delberg.
Pancerz, K., Szkoła, J., Warchoł, J., and Olchowik, G.
(2011). Spectrum disturbance analysis for computer-
aided diagnosis of laryngopathies: An exemplary
study. In Proc. of the International Workshop on
Biomedical Informatics and Biometric Technologies
(BT’2011), Zilina, Slovak Republic.
Quinlan, J. (1993). C4.5. Programs for machine learning.
Morgan Kaufmann Publishers.
Semmlow, J. (2009). Biosignal and Medical Image Pro-
cessing. CRC Press.
Szkoła, J., Pancerz, K., and Warchoł, J. (2010a). Computer-
based clinical decision support for laryngopathies us-
ing recurrent neural networks. In Hassanien, A.
et al., editors, Proc. of the ISDA’2010, pages 627–632,
Cairo, Egypt.
Szkoła, J., Pancerz, K., and Warchoł, J. (2010b). Com-
puter diagnosis of laryngopathies based on temporal
pattern recognition in speech signal. Bio-Algorithms
and Med-Systems, 6(12):75–80.
Szkoła, J., Pancerz, K., and Warchoł, J. (2011a). Improv-
ing learning ability of recurrent neural networks: Ex-
periments on speech signals of patients with laryn-
gopathies. In Babiloni, F. et al., editors, Proc. of the
BIOSIGNALS’2011, pages 360–364, Rome, Italy.
Szkoła, J., Pancerz, K., and Warchoł, J. (2011b). Recurrent
neural networks in computer-based clinical decision
support for laryngopathies: An experimental study.
Computational Intelligence and Neuroscience, 2011.
Article ID 289398.
Warchoł, J. (2006). Speech Examination with Correct and
Pathological Phonation Using the SVAN 912AE Anal-
yser (in Polish). PhD thesis, Medical University of
Lublin.
Warchoł, J., Szkoła, J., and Pancerz, K. (2010). Towards
computer diagnosis of laryngopathies based on speech
spectrum analysis: A preliminary approach. In Fred,
A., Filipe, J., and Gamboa, H., editors, Proc. of the
BIOSIGNALS’2010, pages 464–467, Valencia, Spain.
Winholtz, W. and Titze, I. (1998). Suitability of minidisc
(MD) recordings for voice perturbation analysis. Jour-
nal of Voice, 12(2):138–142.
Witten, I. H. and Frank, E. (2005). Data Mining: Practi-
cal Machine Learning Tools and Techniques. Morgan
Kaufmann.
Wr´oblewski, J. (1998). Genetic algorithms in decomposi-
tion and classification problem. In Polkowski, L. and
Skowron, A., editors, Rough Sets in Knowledge Dis-
covery 2, volume 2, pages 471–487. Physica-Verlag,
Heidelberg, Germany.
A RULE-BASED CLASSIFICATION OF LARYNGOPATHIES BASED ON SPECTRUM DISTURBANCE ANALYSIS
- An Exemplary Study
461