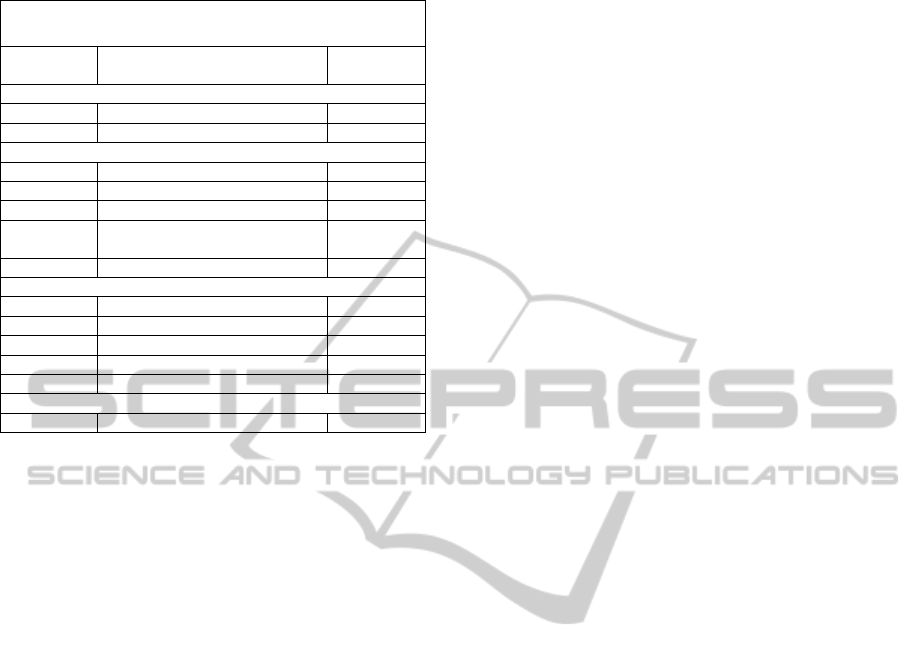
Table 2: Non-SITS input parameters.
Non SITS parameters
In. No. Input Name Input
range
Treatment
26 I.V. Trombolysis Yes/No
27 Stroke care unit Yes/No
Stroke Type
28 Large-artery atherosclerosis Yes/No
29 Cardioembolism Yes/No
30 Small-vessel occlusion Yes/No
31 Stroke of other determined
etiology
Yes/No
32 Haemorrhalgic stroke Yes/No
Laboratory indicators
33 CRP Number
34 Platelets Number
35 Leukocytes Number
36 Fibrinogen Number
37 Vitamin D Number
Other possible laboratory indicators (in the future)
38,39,40,41 IL 6, SAA, NSE, PARK 7 Number
6 CONCLUSIONS
In this paper we have discussed a design of our new
biomedical model for the stroke outcome prediction
which is based on artificial neural network
architecture. First important part of the model
creation was the selection of input and output
parameters. This task was done with the help of
neurological experts from the University Hospital in
Plzen. Than the architecture of an ANN was
designed and also briefly referred in this paper. Now
the model is prepared for training patient´s data set.
The ANN training and optimization of the model are
our main research tasks to the future. The work
presented in this paper is supported by The Czech
Science Foundation project 106/09/0740 dealing
with brain perfusion modelling.
REFERENCES
Adams H. P. Jr., Bendixen B. H., Kappelle L. J., Biller J.,
Love B. B., Gordon D. L., Marsh E. E., 3
rd
, 1993.
Classification of subtype of acute ischemic stroke:
definitions for use in a multicenter clinical trial.
TOAST. Trial of Org 10172 in Acute Stroke
Treatment. Stroke 1993;24:35– 41.
Celler, B. G., Chazal, P., 1998. Low computational cost
classifiers for ECG diagnosis using neural networks.
Proceedings of the International Conference of
Engineering in Medicine & Biology Society (EMBC
1998), pp. 1337–1340.
Elmer Andres Fernández, Rodolfo Valtuille, Jesus
Rodriguez Presedo, and Peter Willshaw (2005)
Comparison of Standard and Artificial Neural Network
Estimators of Hemodialysis Adequacy. International
Center for Artificial Organs and Transplantation.
29(2):159–165, Blackwell Publishing, Inc.
Goldstein L. B., Samsa G. P. Reliability of the National
Institutes of Health Stroke Scale: extensit to non-
neurologists in the context of a clinical trial. Stroke
1997;28:307–10.
Hacke W., Kaste M., Bluhmki E., Brozman M., Da´ valos
A., Guidetti D. et al. Thrombolysis with alteplase 3 to
4.5 hours after acute ischemic stroke. N Engl J Med
2008;359:1317–29.
Ham, P. M. and Han, S., "Classification of cardiac
arrhythmias using fuzzy artmap" IEEE Transactions
on Biomedical Engineering, 43(4): 425–430 (1996).
Lloyd-Jones D., Adams R., Carnethon M., De Simone G.,
Ferguson T. B., Flegal K., et al. Heart disease and
stroke statistics—2009 update: a report from the
American Heart Association Statistics Committee and
Stroke Statistics Subcommittee. Circulation
2009;119:e21–181.
Modai, I., Israel, A., Mendel, S., Hines, E.L. and
Weizman, R., "Neural network based on adaptive
resonance theory as compared to experts in suggesting
treatment for schizophrenic and unipolar depressed in
patients," Journal of Medical Systems, 20(6): 403–412
(1996).
Morgenstern L. B., Lisabeth L. D., Mecozzi A. C., Smith
M. A., Longwell P. J., McFarling D. A., Risser J. M.
A population-based study of acute stroke and TIA
diagnosis. Neurology 2004;62:895–900.
Nazeran, H., Behbehani, K., 2001. Neural networks in
processing and analysis of biomedical signals. In M.
Akay (Ed.), Nonlinear biomedical signal processing:
Fuzzy logic, neural networks and new algorithms, pp.
69–97.
Papaloukas, C.,Fotiadis, D. I.,Likas, A., and Michalis, L.
K., 2002. An ischemia detection method based on
artificial neural networks. Artificial Intelligence in
Medicine, 24, 167– 178.
Shanthi, D., Sahoo, G. and Saravanan, N., 2010. Designing
an Artificial Neural Network Model for the Prediction
of Thrombo-embolic Stroke, International Journals of
Biometric and Bioinformatics (IJBB)
, Volume (3)
SITS International, https://sitsinternational.org
HEALTHINF 2012 - International Conference on Health Informatics
470