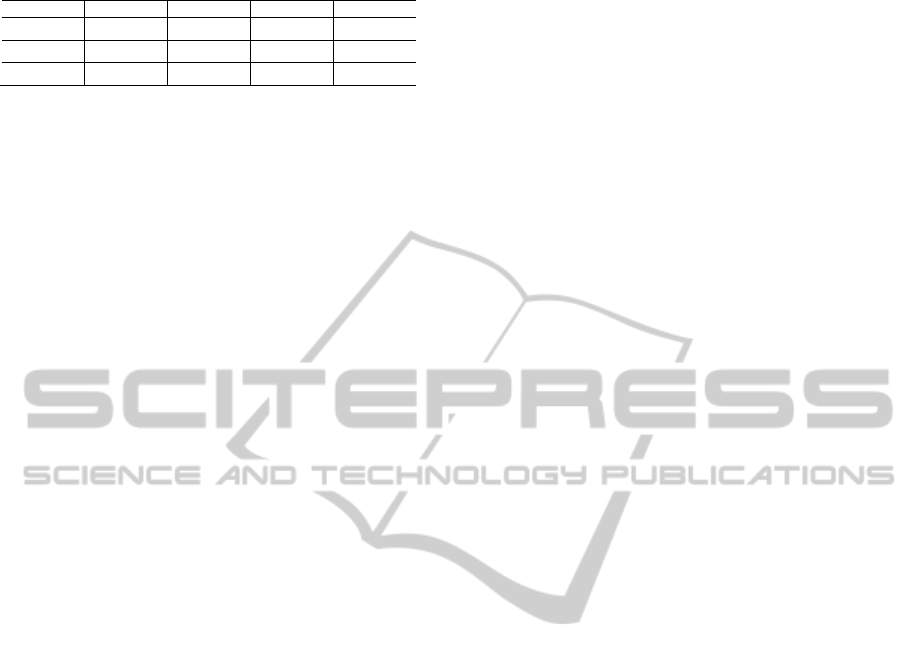
Table 8: Result of emotion classification by SVM.
boredom pain surprise total
boredom
100.0 0.0 0.0
100.0
pain
0.0 100.0 0.0
100.0
surprise 0.0 0.0 100.0 100.0
4 CONCLUSIONS
This study was to classify three different emotional
states (boredom, pain, and surprise) by machine
learning algorithms using physiological features.
Our results showed that physiological responses of
three emotions were differed and SVM were the best
algorithm for classification of three emotions. This
result could help emotion recognition studies lead to
better chance to recognize human emotions by using
physiological signals. Also, it can be useful in
profiling various emotion-specific physiological
responses or establishing the basis for emotion
recognition system in human-computer interaction.
However, this result was the classification
accuracy using only training set which didn’t divide
training and test sets. An average accuracy of
classification is necessary for repeated sub-sampling
validation using training and test sets as the choice
of training and test sets can affect the results.
Therefore, we will perform the average classification
in further analysis. Also, although it is known that
physiological signals offer a great potential for the
recognition of emotions in computer systems, in
order to fully exploit the advantages of physiological
measures, standardization needs to be established on
the emotional model, stimulus used for the
identification of physiological patterns,
physiological measures, parameters for analysis, and
model for pattern recognition and classification
(Arroyo-Palacios & Romano, 2008).
ACKNOWLEDGEMENTS
This research was supported by the Converging
Research Center Program funded by the Ministry of
Education, Science and Technology (No. 2011K000
655 and 2011K000658).
REFERENCES
Wagner, J., Kim, J., Andre, E., 2005. From physiological
signals to emotions: Implementing and comparing
selected methods for feature extraction and
classification, IEEE International Conference on
Multimedia and Expo, Amsterdam, pp. 940-943.
Drummond, P. D., Quah, S. H., 2001. The effect of
expressing anger on cardiovascular reactivity and
facial blood flow in Chinese and Caucasians,
Psychophysiology, vol. 38, pp. 190-196.
Ax, A. R., 1953. The physiological differentiation between
fear and anger in humans, Psychosomatic Medicine,
vol. 15, pp. 147-150.
Boiten, F. A., 1996. Autonomic response patterns during
voluntary facial action, Psychophysiology, vol. 33, pp.
123-131.
Kanade, T. C., Tian, Y., 2000. Comprehensive database
for facial expression analysis, Proceeding of the 4th
IEEE International Conference on Automatic Face
and Gesture Recognition, pp. 46-53.
Palomba, D., Sarlo, M., Angrilli, A., Mini, A., 2000.
Cardiac responses associated with affective processing
of unpleasant film stimulus, International Journal of
Psychophysiology, vol. 36, pp. 45-57.
de Melo, C. M., Kenny, P. G., Gratch, J., 2010. Influence
of autonomic signals on perception of emotions in
embodied agents, Applied Artificial Intelligence, vol. 6,
pp. 494-509.
Flor, H., Knost, B., Birbaumer, N., 2002. The role of
operant conditioning in chronic pain: an experimental
investigation pain, Pain, vol. 95(1-2), pp. 111-118.
Jolliffe, C. D., Nicholas, M. K., 2004. Verbally reinforcing
pain reports: an experimental test of the operant model
of chronic pain, Pain, vol. 107(1-2), pp. 167–175.
Collet, C., Vernet-Maury, E., Delhomme, G., Dittmar, A.,
1997. Autonomic nervous system response patterns
specificity to basic emotions, Journal of the
Autonomic Nervous System, vol. 62, pp. 45-57.
Nasoz, F., Alvarez, K., Lisetti, C. L., Finkelstein, N., 2004.
Emotion recognition from physiological signals using
wireless sensors for presence technologies, Cognition,
Technology and Work, vol. 6, pp. 4-14.
Verhoef, T., Lisetti, C., Barreto, A., Ortega, F., Zant, T.,
Cnossen, F., 2009. Bio-sensing for emotional
characterization without word labels, Human-
Computer Interaction: Ambient, ubiquitous and
intelligent interaction, 13th International Conference,
San Diego, CA, USA, pp. 693-702.
Arroyo-Palacios, J., Romano, D. M., 2008. Towards a
standardization in the use of physiological signals for
affective recognition systems, Proceedings of
Measuring Behavior 2008.
THREE DIFFERENTIAL EMOTION CLASSIFICATION BY MACHINE LEARNING ALGORITHMS USING
PHYSIOLOGICAL SIGNALS - Discriminantion of Emotions by Machine Learning Algorithms
531