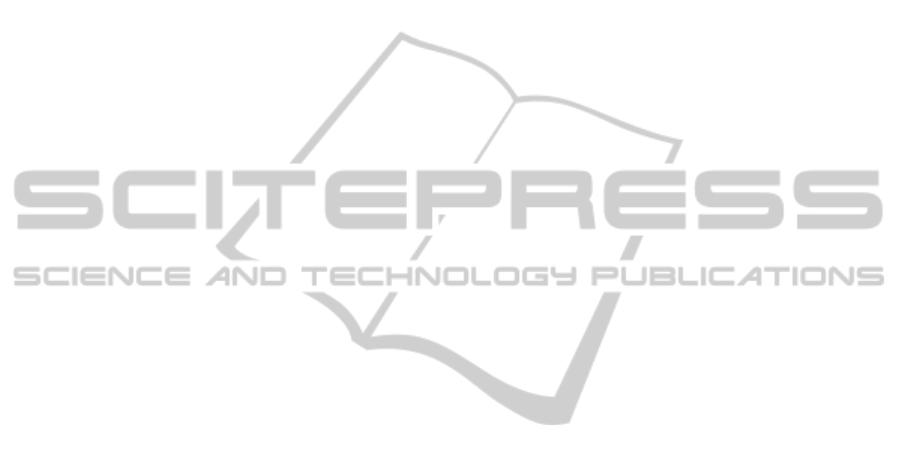
G.: Ubiquitous health in practice: the interreality paradigm. Stud Health Technol Inform
163 (2011) 185-191
3. Riva, G., Raspelli, S., Pallavicini, F., Grassi, A., Algeri, D., Wiederhold, B. K., Gaggioli,
A.: Interreality in the management of psychological stress: a clinical scenario. Stud Health
Technol Inform 154 (2010) 20-25
4. Stone, A. A., Smyth, J. M., Kaell, A., Hurewitz, A.: Structured writing about stressful
events: Exploring potential psychological mediators of positive health effects. Health
Psychology 19 (2000) 619-624
5. Grassi, A., Pallavicini, F., Raspelli, S., Serino, S., Spagnoli, G., Cipresso, P., Villamira, M.,
Gaggioli, A., Riva, G.: Using video clips to induce negative emotional responses. Journal of
CyberTherapy and Rehabilitation 4 (2011) 285-287
6. Ebner-Priemer, U. W., Trull, T. J.: Ambulatory Assessment An Innovative and Promising
Approach for Clinical Psychology. European Psychologist 14 (2009) 109-119
7. Fahrenberg, J., Brugner, G., Foerster, F., Kappler, C.: Ambulatory assessment of diurnal
changes with a hand-held computer: Mood, attention and morningness-eveningness.
Personality and Individual Differences 26 (1999) 641-656
8. Fahrenberg, J., Myrtek, M., Pawlik, K., Perrez, M.: Ambulatory assessment-monitoring
behavior in daily life settings - A Behavioral-Scientific challenge for psychology. European
Journal of Psychological Assessment 23 (2007) 206-213
9. Fahrenberg, J., Myrtek, M.: Progress in ambulatory assessment: Computer-assisted
psychological and psychophysiological methods in monitoring and field studies. Hogrefe &
Huber, Seattle (2001)
10. Stone, A. A., Shiffman, S.: Ecological momentary assessment in behavioral medicine.
Annals of Behavioral Medicine 16 (1994) 199–202
11. Csikszentmihalyi, M., Larson, R.: Validity and Reliability of the Experience-Sampling
Method. Journal of Nervous and Mental Disease 175 (1987) 526-536
12. Stone, A. A., Shiffman, S., Atienza, A. A., Nebeling, L.: The science of real-time data
capture: Self-reports in health research. Oxford University Press., New York, NY (2007)
13. Kahneman, D., Krueger, A. B., Schkade, D. A., Schwarz, N., Stone, A. A.: A survey
method for characterizing daily life experience: the day reconstruction method. Science 306
(2004) 1776-1780
14. Barrett, L. F., Barrett, D. J.: An introduction to computerized experience sampling in
psychology. Social Science Computer Review 19 (2001) 175-185
15. Houtveen, J. H., de Geus, E. J. C.: Noninvasive Psychophysiological Ambulatory
Recordings Study Design and Data Analysis Strategies. European Psychologist 14 (2009)
132-141
16. Intille, S.S.: A new research challenge: Persuasive technology to motivate healthy aging.
Ieee Transactions on Information Technology in Biomedicine 8 (2004) 235-237
17. Repetto, C., Gaggioli, A., Pallavicini, F., Cipresso, P., Raspelli, S., Riva, G.: Virtual reality
and mobile phones in the treatment of generalized anxiety disorders: a phase-2 clinical trial.
Personal and Ubiquitous Computing (in press)
18. Intille, S. S.: Technological innovations enabling automatic, context-sensitive ecological
momentary assessment. In: Stone, A.A., Shiffman, S., Atienza, A.A., Nebeling, L. (eds.):
The science of real-time data capture: Self-reports in health research. Oxford University
Press, New York, NY (2007) 308–337
19. Gaggioli, A., Pioggia, G., Tartarisco, G., Baldus, G., Corda, D., Cipresso, P., Riva, G.: A
Mobile Data Collection Platform for Mental Health Research. Personal and Ubiquitous
Computing (in press)
20. Jacobs, N., Myin-Germeys, I., Derom, C., Delespaul, P., van Os, J., Nicolson, N. A.: A
momentary assessment study of the relationship between affective and adrenocortical stress
responses in daily life. Biological Psychology 74 (2007) 60-66
21. Magagnin, V., Mauri, M., Cipresso, P., Mainardi, L., Brown, E. N., Cerutti, S., Villamira,
M., Barbieri, R.: Heart rate variability and respiratory sinus arrhythmia assessment of
33