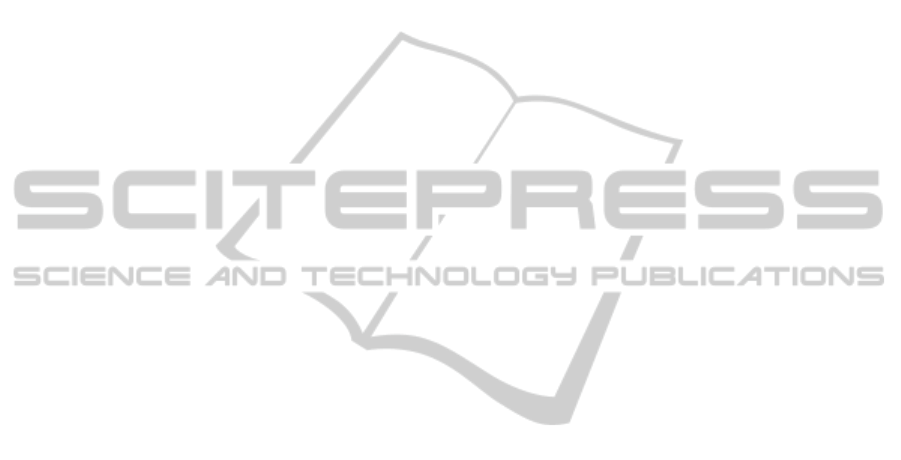
assume that both upper legs are horizontal, the sitting activity on the exercise bike is
not identified correctly. The identification of the cycling activity for user 03 shows
that further features may be needed to identify the difference between walking and
cycling accurately.
For user 04 results show good identification rates. Although sitting and lying are
confused 100% of the time, the trunk orientation of the user would give a potentially
perfect feature to correctly identify sitting. The identification of static versus dynamic
activities is 100% for this participant and the correct identification of walking and
cycling 98% and 85% respectively. Similar to user 03, the correct identification of
cycling is still rather low whereas the correct identification of walking is similar at
98%, which suggests that the algorithms could be improved by fine-tuning the identi-
fication of cycling. As data analysis of these trials is ongoing, more extensive results,
also including the other activities performed during the trial, will be published in a
subsequent paper.
4 Conclusions
This paper outlines the use of mobile phones in the treatment of depression as a
means of communication with the user, presentation of the treatment modules, sensor
data gatherer and physical activity monitor in its own right. The focus of this paper
was on the latter and two physical activity monitoring trials performed as part of the
ICT4Depression project are described. The inherent challenge in measuring physical
activity with a sensor whose orientation is not known, is addressed through a data
transformation from device-fixed coordinates to world-fixed coordinates through the
use of an approximated gravity vector. The world-fixed acceleration signal is then
used to apply thresholding based algorithms to the identification of various activities.
Preliminary results show that the phone can lead to a reasonable estimate of user
activity although it is also clear that further work is needed to obtain accuracies simi-
lar to these obtained with traditional sensors.
References
1. Andrews et al: Utilising survey data to inform public policy: comparison of the cost-
effectiveness of treatment of ten mental disorders. British Journal of Psychiatry, (2004)
184, 526-33
2. Ströhle, A.: Physical activity, exercise, depression and anxiety disorders, J Neural Transm,
(2009) Vol. 116, pp. 777–784.
3. Blumenthal J. A., Babyak M. A., Moore K. A. et al.: Effects of exercise training on patients
with major depression. Arch Int Med (1999) 159:2349–2356.
4. Maed et al.: Exercise for depression, Cochrane Database of Systematic Reviews ,(2008),
Issue 4. Art. No.: CD004366. DOI:10.1002/14651858.CD004366.pub3.
5. Manohar, C., Mc Crady, S., Fujiki, Y., Pavlidis, I. and Levine, J.: Laboratory evaluation of
the accuracy of a triaxial accelerometer embedded into a cell phone platform for measuring
physical activity. 2010 Experimental Biology meeting (2010)
6. Bieber, G., Voskamp, J., and Urban, B.: Activity Recognition for Everyday Life on Mobile
Phones. UAHCI '09 Proceedings of the 5th International Conference on Universal Access
112