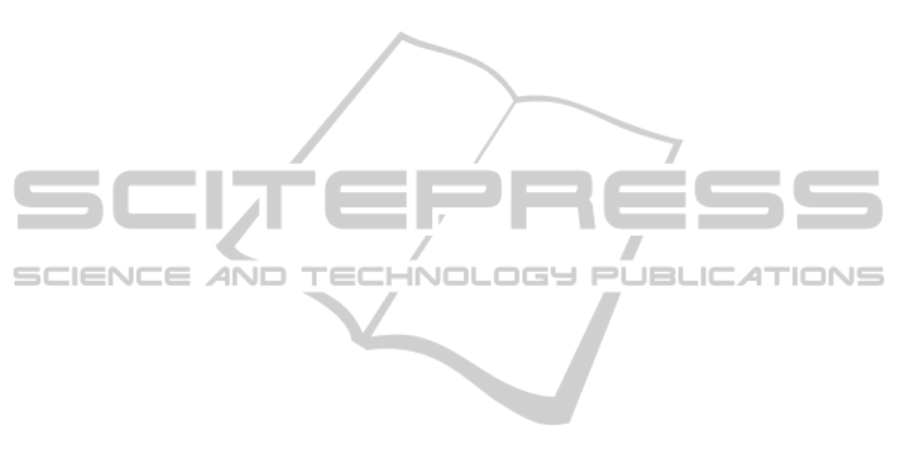
measure, and which experiments to conduct, in the
field of synthetic biology.
The other fundamental aspect of our approach is
the use of evolution to refine the designs arrived at
by humans or machines. The uncertainty inherent in
biological systems—whether arising from inherent
stochasticity or our lack of knowledge about the
structure and function of many biomolecules—
means that a completely rational design strategy in
synthetic biology, as espoused by hard-core
engineers, is simply not practical at this point in
time. By harnessing evolution to refine our design,
and then comparing the products of evolution with
our original designs, we have the potential to learn
not only how to better engineer the organisms in
which we are interested, but also how these
organisms work in the absence of engineering.
Molecular and systems biology form the basis for
synthetic biology; but synthetic biology also
promises to provide unique insights into the
fundamental workings of the cell.
A highly automated approach, incorporating
computational intelligence wherever possible, and
operating at the level of one or a few cells, appears
to us to offer the best prospects for designing,
implementing and testing large-scale novel genetic
systems, thus bridging the gap between design and
reality in synthetic biology. Although there are still
many technical hurdles to be overcome in the
construction of such a system, all of the individual
technologies are currently in place, and the
construction of such a synthetic biology factory is a
realistic goal in the near future.
REFERENCES
Aharoni, A., L. Gaiducov, et al. (2005). "The 'evolvability'
of promiscuous protein functions." Nature Genetics
37(1): 73 - 76.
Beal, J., T. Lu, et al. (2011). "Automatic compilation from
high-level biologically-oriented programming
language to genetic regulatory networks." PLoS ONE
6(8): e22490.
Box, G. E. P. (1957). "Evolutionary operation: A method
for increasing industrial productivity." Applied
Statistics 6(2): 81 - 101.
Brustad, E. M. and F. H. Arnold (2011). "Optimizing non-
natural protein function with directed evolution."
Current Opinion in Chemical Biology 15(2): 201 -
210.
Cello, J., A. V. Paul, et al. (2002). "Chemical synthesis of
poliovirus cDNA: Generation of infectious virus in the
absence of natural template." Science 297(5583): 1016
- 1018.
Chandran, D., F. T. Bergmann, et al. (2009) "TinkerCell:
modular CAD tool for synthetic biology." Journal of
Biological Engineering 3 DOI: doi:10.1186/1754-
1611-3-19.
Church, G. M. (2005). "From systems biology to synthetic
biology." Molecular Systems Biology 1: 0032.
Cohen, J. (2008). "The crucial role of CS in systems and
synthetic biology." Transactions of the ACM 51(5): 15
- 18.
Cooling, M. T., P. Hunter, et al. (2008). "Modelling
biological modularity with CellML." Systems Biology,
IET 2(2): 73-79.
Craddock, T., C. R. Harwood, et al. (2008). "e-Science:
Relieving bottlenecks in large-scale genomic
analyses." Nature Reviews Microbiology 6: 948 - 954.
Czar, M., Y. Cai, et al. (2009). "Writing DNA with
GenoCAD." Nucleic Acids Research 37(W40-7).
Felton, M. J. (2003). "Product review: Liquid handling:
Dispensing reliability." Analytical Chemistry 75(17):
397A - 399A.
Ferry, M. S., I. A. Razinkov, et al. (2011). "Microfluidics
for synthetic biology: From design to execution."
Methods in Enzymology 497: 295 - 372.
Galperin, M. Y. and G. R. Cochrane (2011). "The 2011
Nucleic Acids Research database issue and the online
molecular biology database collection." Nucleic Acids
Research 39(Suppl 1).
Gibson, D. G., J. I. Glass, et al. (2010). "Creation of a
bacterial cell controlled by a chemically synthesized
genome." Science 329(5987): 52 - 56.
Gilman, A. and J. Ross (1995). "Genetic-algorithm
selection of a regulatory structure that directs flux in a
simple metabolic model." Biophysical Journal 69(4):
1321 - 1333.
Gulati, S., V. Rouilly, et al. (2009). "Opportunities for
microfluidic technologies in synthetic biology."
Journal of the Royal Society Interface 6(Suppl_4):
S493-S506.
Hallinan, J., G. Misirli, et al. (2010). Evolutionary
computation for the design of a stochastic switch for
synthetic genetic circuits. 32nd Annual International
Conference of the IEEE Engineering in Medicine and
Biology Society (EMBC 2010), Buenos Aires,
Argentina.
Hallinan, J. and J. Wiles (2002). Evolutionary algorithms.
The Encyclopedia of Cognitive Sciences. L. Nadel.
New York, Palgrave Macmillan.
Hucka, M., A. Finney, et al. (2003). "The systems biology
markup language (SBML): A medium for
representation and exchange of biochemical network
models." Bioinformatics 19: 524-531.
Khalil, A. S. and J. J. Collins (2010). "Synthetic biology:
Applications come of age." Nature Reviews Genetics
11(5): 367 - 379.
King, R. D., J. Rowland, et al. (2009). "The Automation of
Science." Science 324(5923): 85-89.
King, R. D., K. E. Whelan, et al. (2004). "Functional
genomic hypothesis generation and experimentation
by a robot scientist." Nature 427(6971): 247-252.
BRIDGING THE GAP BETWEEN DESIGN AND REALITY - A Dual Evolutionary Strategy for the Design of Synthetic
Genetic Circuits
267