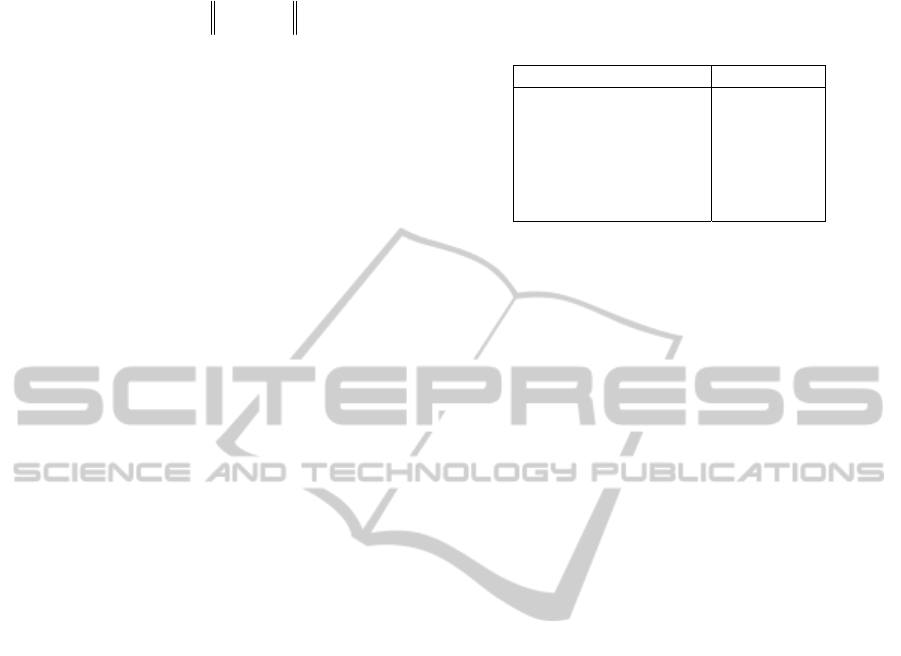
achieves the minimum distance, i.e.,
test
minarg
*
*
x
b
bbb
j
i
j
i
j
i
The proposed classification then assigns the
unknown
test
x to the reference action
*
i . In order to
rule out a novel action (i.e., an action irrelevant to
any of the reference actions of interest), a simple
distance threshold can be applied to exclude the
unrecognizable action.
3 EXPERIMENTAL RESULTS
This section evaluates the effectiveness of the
proposed action recognition scheme with the Public
Weizmann action dataset (Gorelick et al., 2007). In
the Weizmann dataset, we compare the experimental
results of the proposed method and several existing
methods with the Weizmann dataset. The Weizmann
dataset contains 90 low-resolution (180
144) video
sequences that show 9 different people, each
performing 10 natural actions of Run, Walk, Skip,
Jumping-jack, Jump forward on two legs, Jump in
place on two legs, Gallop sideways, Wave two
hands, wave one hand, and Bend. All the
backgrounds are stationary. We use 9 training
samples for each individual reference action,
wherein each EMHI training sample is obtained
from the last frame of each sequence. In the training
stage, a total of 90 (10 actions
9 people) video
representation sequences are collected as the training
data matrix.
The proposed method gave the same detection
results as those of the state-of-the-art methods (e.g.,
volume-based method [27], spatiotemporal methods
(Gorelick et al., 2007); (Hsiao et al., 2008), model-
based method [29] and optical flow-based method
[30]), as seen in Table 1. The existing methods
generally use shapes or silhouettes for the
representation, highly rely on accurate segmentation
of foreground shapes, and require high computation
complexity. They may fail to recognize the actions
of interest on a disturbed background.
The proposed algorithms were implemented
using the C++ language on a Core2 Duo, 2.53GHz
personal computer. The test images in the
experiments were
150200 pixels wide with 8-bit
gray levels. The total computation time from
foreground segmentation and spatiotemporal
representation to ICA feature extraction and distance
measure for an input image was only 0.015 seconds.
It achieved a mean of 67 fps (frames per second) for
real-time action recognition.
Table 1: Performance comparison of different methods on
the Weizmann dataset.
Methods Accuracy
Grundmann et al. 2008 94.6 %
Hsiao et al. 2008 96.7 %
Wang and Suter 2007 97.8 %
Fathi and Mori 2008 100 %
Gorelick et al. 2007 100 %
Our proposed method 100 %
4 CONCLUSIONS
The proposed ICA-based feature extraction and
classification method has been well applied to the
global spatiotemporal representation of EMHI for
recognizing activities that can be observed from a
global, macro viewpoint. It is worth further
investigation to extend the ICA-based scheme for
recognizing subtle activities with micro-observation
representation that can be observed only from a local
viewpoint of detailed body movements of individual
foreground objects.
REFERENCES
Gorelick, L., Blank, M., Shechtman, E., Irani, M., Basri,
R., 2007. Actions as Space-Time Shapes. IEEE Trans.
Pattern Analysis and Machine Intelligence, vol. 29, no.
12, pp. 2247-2253.
Hyvarinen, A., and Oja, E., 1997. A fast fixed-point
algorithm for independent component analysis. Neural
Computation, vol. 9, pp. 1483-1492.
Hurri, J., Gavert, H., Sarela, J., and Hyvarinen, A., 2004.
FastICA Package. Online Available:
http://www.cis.hut.fi/projects/ica/fastica/.
Grundmann, M., Meier, F., and Essa, I., 2008. 3D shape
context and distance transform for action recognition.
In: Proc. IEEE Conference on Computer Vision and
Pattern Recognition.
Hsiao, P. C., Chen, C. S., and Chang, L. W., 2008. Human
action recognition using temporal-state shape contexts.
In: Proc. IEEE Conference on Computer Vision and
Pattern Recognition.
Wang, L. and Suter, D., 2007. Recognition human
activities from silhouettes: Motion subspace and
factorial discriminative graphical model. In: Proc.
IEEE Conference on Computer Vision and Pattern
Recognition.
Fathi, A. and Mori, G., 2008. Action Recognition by
Learning Mid-level Motion Features. In: Proc. IEEE
Conference on Computer Vision and Pattern
Recognition.
GRAPP 2012 - International Conference on Computer Graphics Theory and Applications
522