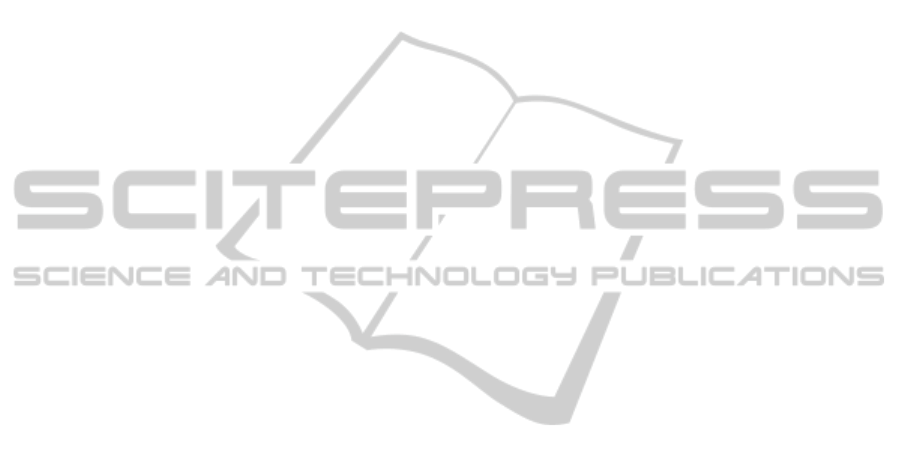
From the agreement figures shown in the Ta-
ble 1 we can conclude that agreement between human
raters is significantly higher than that of automated
techniques with expert annotation despite operating
on the same kind of input data (PSG).
In this paper we compare performances of the
RHA and Facial PSG approaches on the task of auto-
mated epoch bases sleep state annotation. This paper
is organized as follows. Section 2 describes the exper-
imental setup. In Section 3 we present the signal pro-
cessing and machine learning methods that were used
in this study. The results are discussed in Section 4.
Finally Section 5 summarizes the main conclusions of
this work and proposes future research directions.
2 EXPERIMENT DESCRIPTION
This study considers two data sets: First and Second,
acquired in two separate studies. The First dataset in-
cludes respiratory effort, cardiac, and acti-graphy sig-
nals. The RHA approach was tested on the First data
set.
The Second dataset was used for validation of the
Facial PSG approach which had as a major objective
to show that sleep state estimation can be performed
with electrodes applied on a single side of the face
(for which we used EOG-Left placed above the eye
and mastoid reference). Further details on the datasets
are provided in the next sections.
2.1 FirstDataset
The First data set contains overnight PSG recordings
of six young healthy volunteers (mean age 27 y.o., 4
males and 2 females). In a screening phase, selec-
tion of participants was based on absence of subjec-
tive sleep complaints and regular sleep/wake patterns.
Participants entered the sleep laboratory at 21.00h
and were prepared for PSG measurements. Lights
were turned off at around 23.00h. The waking up sig-
nal was given around 7 o’clock.
Sleep recordings and analysis Polysomnographic
sleep recordings were obtained during all sleep
episodes with a digital recorder, and included EEG
(F3/A2, F4/A1, C3/A2, C4/A1, O1/A2, O2/A1)
sampled at 100Hz, electrooculogram (EOG) sam-
pled at 100Hz, electrocardiogram (ECG) sampled
at 500Hz and chin electromyogram (EMG) sam-
pled at 200Hz. Respiratory effort was measured
with chest and abdominal respiratory effort belts at
10Hz. Obtained PSG recording were scored into
sleep stages using 30s epochs according to standard
criteria (Rechtschaffen and A.Kales, 1968) by the
Alice Philips Resperonics system and further proof
checked by human experts.
2.2 SecondDataset
The Second data set contains overnight PSG record-
ings of six young healthy volunteers (mean age 27, 4
males and 2 females). They signed a consent form. In
a screening phase, selection of participants was based
on absence of subjective sleep complaints and regu-
larity of sleep/wake patterns. Screening was based
on two questionnaires: the Sleep Disorders Question-
naire (SDQ) (Douglass et al., 1994) and the Pittsburgh
Sleep Quality Index (PSQI) (Buysse et al., 1989). All
selected participants scored within the normal range
of the PSQI. Moreover, the participant should not
score higher than the cutoff scores on the subscales
narcolepsy, apnea, restless legs, and psychiatry of the
SDQ (Douglass et al., 1994).
Participants entered the sleep laboratory at 21.00h
and were prepared for Polysomnographic measure-
ments. Lights were turned off at around 0.00h. The
waking up signal was given around 7 o’clock.
Polysomnographic sleep recordings were ob-
tained during all sleep episodes with a digital
recorder (Vitaport-3, TEMEC Instruments, Kerkrade,
Netherlands), and included EEG (F3/A2, F4/A1,
C3/A2, C4/A1, O1/A2, O2/A1) obtained with the
Sleep BraiNet system (Jordan NeuroScience, San
Bernardino, CA), electrooculogram (EOG), electro-
cardiogram (ECG) and chin electromyogram (EMG).
Respiratory effort was measured with Pro-Tech chest
and abdominal respiratory effort belts (Pittsburgh,
USA). The signals were recorded at a sampling fre-
quency of 256Hz. Obtained PSG recording were
scored by the Siesta Group’s software system - ”Som-
nolyzer” and further proof checked by Siesta’s ex-
perts.
3 METHODS
3.1 Feature Extraction
In the following two subsections we describe the
data preprocessing and feature extraction methodol-
ogy used for both the RHA and the Facial PSG ap-
proaches.
3.1.1 RHA Features
The raw respiration signal was first lowpass fil-
tered (cut-off 0.5Hz) and then analyzed for individ-
ual breaths. Based on a localized min/max filter, lo-
HEALTHINF 2012 - International Conference on Health Informatics
488