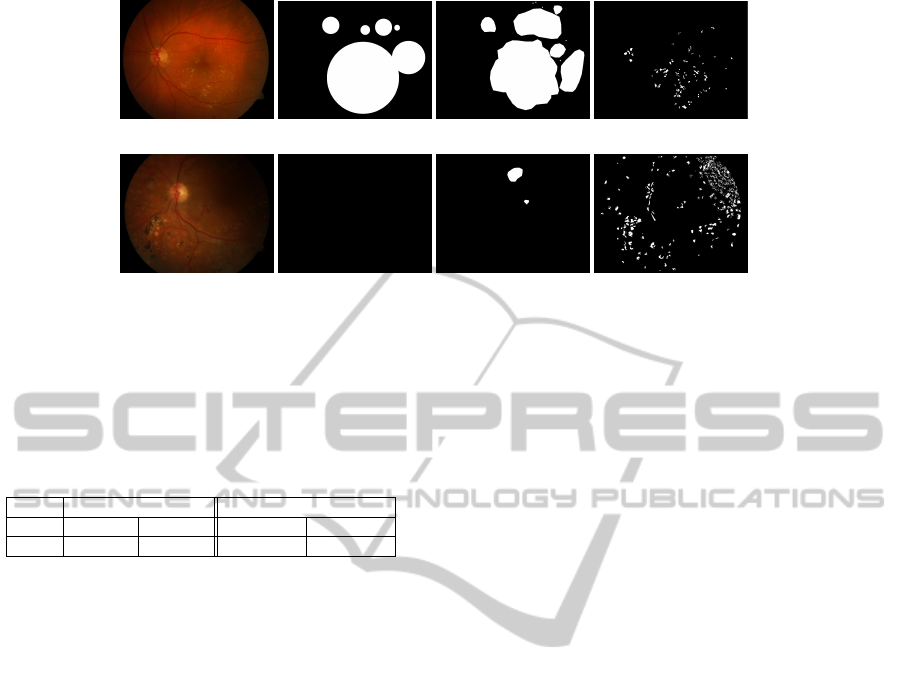
(a) (b) (c) (d)
(e) (f) (g) (h)
Figure 4: (a) and (e) Original images. (b) and (f) Hard exudates found by Expert 1. (c) and (g) Hard exudates found by Expert
2. (d) and (h) Hard exudates found by our method.
Table 1: Results for evaluation of automatic detection of
exudates and optic disc. The average area of exudates over-
lapped with the areas highlighted by the experts is pre-
sented. Sensitivity and specificity is shown for optic disc
detection.
Hard exudates Optic Disc
Expert 1 Expert 2 Sensitivity Specificity
Mean 60.75% 63.91% 72.12% 95.56%
negatives number of pixels respectively . In Table 1
results for sensitivity and specificity are shown for
25 images from IMAGERET (Kauppi et al., 2009)
database. In average, our methods detect the OD with
sensitivity of 72.12% and specificity of 95.56%.
Ideally, the evaluation of exudates should be done
similar to that of the optic disc, however the database
we used does not allow us to make that assessment.
It is not possible to compare our preliminary results
with other studies since most of these works used their
own databases which are not public. It is necessary
therefore the generation of a public database of pat-
terns marked by experts accurately for a reliable eval-
uation and comparison.
5 CONCLUSIONS
Methods for automatic detection of hard exudates
and optic disc in fundus images were presented.
These methods preserved computational simplicity,
still achieving good results even for images with a
wide range of lightening conditions. Differences in
the selection of exudates by the experts and meth-
ods here presented complicated the definition of good
evaluation techniques. Selection of the optic disc
by the experts was more specific, allowing a better
evaluation of concordance. From our preliminary re-
sults we concluded that a more pattern specific public
database make by experts is needed in order to have a
more reliable evaluation. Work is being done in this
issue.
REFERENCES
Fleming, A., Philip, S., Goatman, K., Olson, J., and Sharp,
P. (2006). Automated microaneurysm detection us-
ing local contrast normalization and local vessel de-
tection. IEEE Transactions on Medical Imaging,
25(9):1223–1232.
Foracchia, M., Grisan, E., and Ruggeri, A. (2004). Detec-
tion of optic disc in retinal images by means of a ge-
ometrical model of vessel. IEEE Trans. Med. Imag.,
23(10):1189–1195.
Kauppi, T., Kalesnykiene, V., Kamarainen, J., Lensu, L.,
and et. al. (2009). Imageret-database. www2.it.lut.fi/
project/imageret/.
Osareh, A., Mirmehdi, M., Thomas, B., and Markham, R.
(2001). Automatic recognition of exudative macu-
lopathy using fuzzy c-means clustering and neural net-
works. In Proc Medical Image Understanding and
Analysis, pages 49–52.
Otsu, N. (1979). A threshold selection method from gray-
level histograms. IEEE Trans. Syst., Man, Cybern.,
9:62–66.
Pereira Delgado, E. (2005). Nuevas perspectivas en oftal-
molog
´
ıa: Retinopat
´
ıa diab
´
etica. Glosa, Laboratorios
Esteve.
Phillips, R., Forrester, J., and Sharp, P. (1993). Auto-
mated detection and quantification of retinal exudates.
Graefe’s Archive for Clinical and Experimental Oph-
thalmology, 231(2):90–94.
Sinthanayothin, C., Boyce, J., Cook, H., and Williamson,
T. (1999). Automated localization of the optic disc,
fovea, and retinal blood vessels from digital colour
fundus images. British Journal of Ophthalmology,
83(8):902–910.
Wong, D. W. K., Liu, J., Lim, J. H., Jia, X., Yin, F., Li, H.,
and Wong, T. Y. (2008). Level-set based automatic
BIOSIGNALS 2012 - International Conference on Bio-inspired Systems and Signal Processing
546