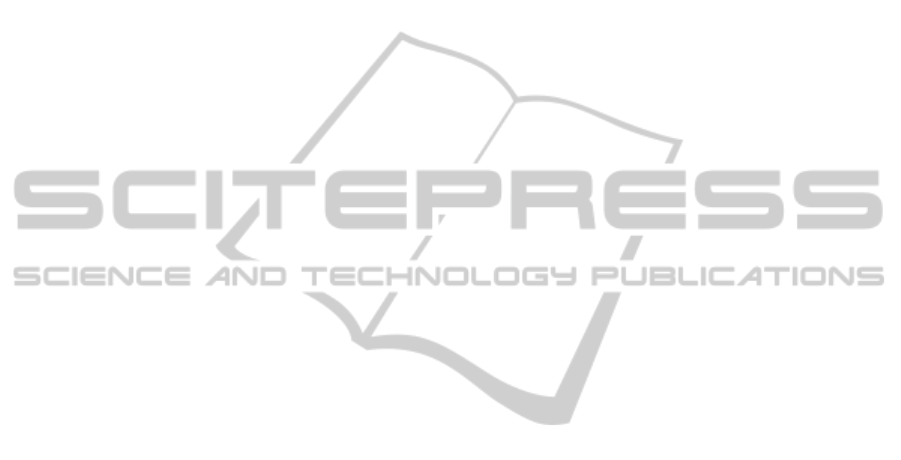
ognition rates above 70% are achieved when classifying positive and negative emo-
tions using LOOCV estimates with a k-NN Classifier. For future work, classification
based on criterions such as the emotion valence and arousal will be used; other emo-
tion elicitation techniques such as pictures, sounds, games can also be inserted and
tested in the developed Web application; acquiring new electrophysiological data and
extend our current database is also a future goal.
Acknowledgements
This work was partially supported by the National Strategic Reference Framework
(NSRF-QREN) programme under contract no. 3475 (Affective Mouse), and partially
developed under the grant SFRH/BD/65248/2009 from Fundação para a Ciência e
Tecnologia (FCT), whose support the authors gratefully acknowledge.
References
1. Filipe Canento. Affective mouse. Master’s thesis, IST-UTL, 2011.
2. Filipe Canento, Ana Fred, Hugo Silva, Hugo Gamboa, and André Lourenço. Multimodal
biosignal sensor data handling for emotion recognition. In Proceedings of the IEEE Sensors
Conference, 2011.
3. Hugo Gamboa. Multi-Modal Behavioural Biometrics Based on HCI and Electrophysiology.
PhD thesis, IST-UTL, 2006.
4. André Lourenço, Hugo Silva, and Ana Fred. Unveiling the biometric potential of Finger-
Based ECG signals. Computational Intelligence and Neuroscience, 2011.
5. A. Fred, H. Gamboa, and H. Silva, “Himotion project,” tech. rep., Universidade Técnica de
Lisboa, Instituto Superior Técnico, 2007.
6. PLUX, “PLUX Website”, www.plux.info, March 2011.
7. R. Picard, Affective Computing. MIT press, 1997.
8. J. Larsen, G. Berntson, K. Poehlmann, T. Ito, and J. Cacioppo, “The psychophysiology of
emotion,” in The handbook of emotions, pp. 180–195, Guilford, 2008.
9. M. Whang and J. Lim, “A physiological approach to affective computing,” in Affective
computing: Focus on Emotion Expression, Synthesis and Recognition (J. Or, ed.), pp. 309–
318, In-Tech Education and Publishing, 2008.
10. L Shen, M.Wang, and R. Shen, “Affective e-learning: Using “emotional” data to improve
learning in pervasive learning environment,” Educational Technology & Society, 2009.
11. Haag, S. Goronzy, P. Schaich, and J. Williams, “Emotion recognition using bio-sensors:
First steps towards an automatic system,” in Affective Dialogue Systems: Lecture Notes in
Computer Science, pp. 36–48, Ed. Springer Berlin, 2004.
12. E. Leon, G. Clarke, V. Callaghan, and F. Sepulveda, “A user-independent real-time emo-
tion recognition system for software agents in domestic environments,” Engineering Appli-
cations of Artificial Intelligence, 2006.
13. K. Kim, S. Bang, and S. Kim, “Emotion recognition system using short-term monitoring of
physiological signals,” Medical & Biological Engineering & Computing, 2004.
14. F. Hönig, A. Batliner, and E. Nöth, “Real-time recognition of the affective user state with
physiological signals,” in Proceedings of the Doctoral Consortium of the 2nd International
Conference on Affective Computing and Intelligent Interaction, pp. 1–8, 2006.
66