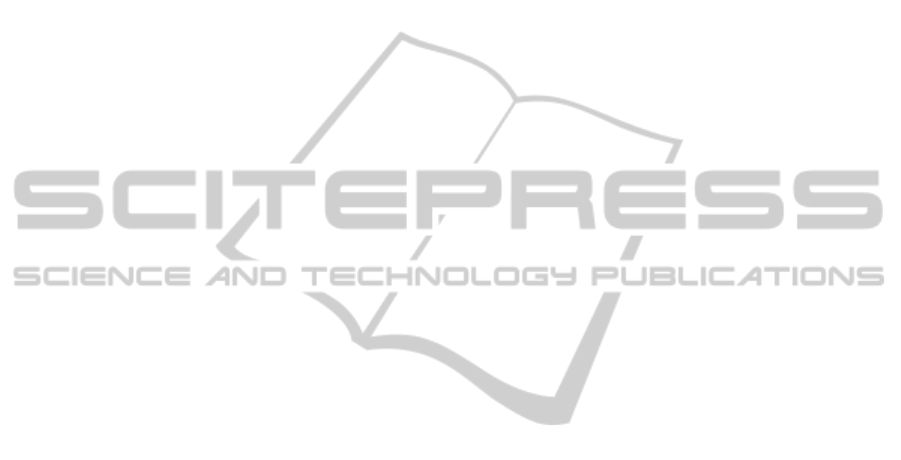
in (Tsai et al., 2007). Respect to the proposed cloud-
based architecture, these SOA-based solutions cover
only a particular aspect (i.e., data quality assessment
(Zhou et al., 2009) and data provenance (Tsai et al.,
2007)) of a data quality improvement process.
A very limited number of papers (Faruquie et al.,
2010; Dani et al., 2010) proposes a cloud-based solu-
tion to perform data quality improvementactivities. A
cloud infrastructure to offer virtualized data cleansing
that can be accessed as a transient service is presented
in (Faruquie et al., 2010). Setting up data cleansing
as a transient service gives rise to several challenges
such as (i) defining a dynamic infrastructure for the
cleansing on demand based on customer requirements
and (ii) defining data transfer and access that meet re-
quired service level agreements in terms of data pri-
vacy, security, network bandwidth and throughput.
Moreover, as further discussed in (Dani et al., 2010),
offering data cleansing as a service is a challenge be-
cause of the need to customize the rules to be applied
for different datasets. The Ripple Down Rules (RDR)
framework is proposed in (Dani et al., 2010) to lower
the manual effort required in rewriting the rules from
one source to another. The solutions in (Faruquie
et al., 2010; Dani et al., 2010) face challenges similar
to the ones tackled in this paper but they focused only
on data cleansing and not to a complete data quality
improvement process. Moreover, the contract-based
service selection is not addressed.
6 CONCLUSIONS AND FUTURE
WORKS
Cloud computing models offer powerful solutions to
reduce costs when performing data quality improve-
ments by using software and data offered as services
on-demand. However, since data quality improve-
ments potentially require the sharing of business criti-
cal data, these services should act in compliance with
predefined contracts. This paper has extended pre-
vious works on the definition of methods and tech-
niques for the specification, selection and evaluation
of service and data contracts. Moreover, this paper
has proposed an extension for the DataFlux dfPower
Studio architecture that supports contract-based ser-
vice selection for data quality improvement activities
in the cloud. Experimental activities on the PATSTAT
database have demonstrated the feasibility of the pro-
posed solutions.
Future works deal with some open issues concern-
ing data transfer and resource allocation for data pro-
cessing services over the cloud.
ACKNOWLEDGEMENTS
This work is supported by the SAS Institute srl (Grant
Carlo Grandi). The author wants to thank Andrea
Scrivanti for his precious contribution in this work.
REFERENCES
Batini, C. and Scannapieco, M. (2006). Data Quality: Con-
cepts, Methodologies and Techniques (Data-Centric
Systems and Applications). Springer-Verlag.
Coen-Porisini, A., Colombo, P., and Sicari, S. (2010). Deal-
ing with anonymity in wireless sensor networks. In
Proc. of SAC 2010, pages 2216–2223. ACM.
Comerio, M., De Paoli, F., and Palmonari, M. (2009a). Ef-
fective and flexible nfp-based ranking of web services.
In Proc. of ICSOC/ServiceWave 2009, pages 546–560.
Comerio, M., Truong, H.-L., Batini, C., and Dustdar, S.
(2010). Service-oriented data quality engineering and
data publishing in the cloud. In Proc. of SOCA 2010,
pages 1–6.
Comerio, M., Truong, H.-L., De Paoli, F., and Dustdar, S.
(2009b). Evaluating contract compatibility for service
composition in the seco2 framework. In Proc. of IC-
SOC/ServiceWave 2009, pages 221–236.
Dani, M. N., Faruquie, T. A., Garg, R., Kothari, G., Mo-
hania, M. K., Prasad, K. H., Subramaniam, L. V.,
and Swamy, V. N. (2010). A knowledge acquisition
method for improving data quality in services engage-
ments. In Proc. of SCC 2010, pages 346–353.
De Paoli, F., Palmonari, M., Comerio, M., and Maurino, A.
(2008). A Meta-Model for Non-Functional Property
Descriptions of Web Services. In Proc. of ICWS 2008,
pages 393–400.
Faruquie, T. A., Prasad, K. H., Subramaniam, L. V., Mo-
hania, M. K., Venkatachaliah, G., Kulkarni, S., and
Basu, P. (2010). Data cleansing as a transient service.
In Proc. of ICDE 2010, pages 1025–1036.
Li, J., Stephenson, B., and Singhal, S. (2009). A policy
framework for data management in services market-
places. In Proc. of ARES 2009, pages 560–565.
Machanavajjhala, A., Kifer, D., Gehrke, J., and Venkitasub-
ramaniam, M. (2007). L-diversity: Privacy beyond
k-anonymity. ACM Trans. Knowl. Discov. Data, 1.
Truong, H.-L., Gangadharan, G., Comerio, M., Dustdar, S.,
and De Paoli, F. (2011). On analyzing and developing
data contracts in cloud-based data marketplaces. In
Proc. of APSCC 2011, pages 174–181.
Tsai, W.-T., Wei, X., Zhang, D., Paul, R., Chen, Y., and
Chung, J.-Y. (2007). A new soa data-provenance
framework. In Proc. of ISADS 2007, pages 105–112.
Viega, J. (2009). Cloud computing and the common man.
Computer, 42:106–108.
Zhou, Y., Hanß, S., Cornils, M., Hahn, C., Niepage, S., and
Schrader, T. (2009). A soa-based data quality assess-
ment framework in a medical science center. In Proc.
of ICIQ 2009, pages 149–160.
ACLOUD-BASEDSOLUTIONFORDATAQUALITYIMPROVEMENT
227