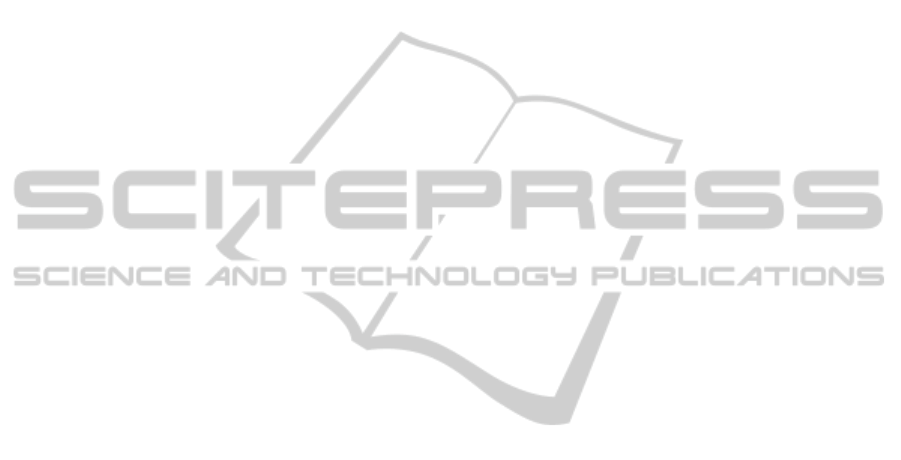
The contributions of this position paper will be
further developed. In fact, the application of the
proposed approach requires an extensive
experimentation in cooperation with a medical staff
and a sample group of patients suffering from HF.
Moreover, before the real deployment of such a
smartphone-based user activity recognition method,
the recognized movements’ set should be enriched
with other typical activities such as climbing/down
the stairs and indoor/outdoor cycling, treadmill.
2 RELATED WORK
In recent years a significant amount of work has
been proposed concerning context-aware services
relying on accelerometer data. Application fields are
diverse and include remote health-care (Ryder,
2009), social networking and Activity Recognition
(Miluzzo, 2008). Differently from the approach
proposed in this paper, some of the proposed
methods are designed to work with ad hoc sensors
worn by the users (Keally, 2011). Other methods are
developed for commoner devices such as
smartphones. For example, Nokia’s N95 is a popular
choice (e.g., (Miluzzo, 2008), (Wang, 2009) and
(Ryder, 2009)) have been implemented on it), but
newer devices have received some attention as well:
an iPhone version of (Miluzzo, 2008) has been
developed and (Ryder, 2009) has also been
implemented on Android smartphones. Other
methods stand in between, requiring both custom
hardware and off-the-shelf devices. For example, the
method described in (Keally, 2011) employs an
Android smartphone and ad-hoc wearable sensors.
A Paper Contribution. The proposed method is
implemented on an Android smartphone and it takes
into account the limitations of mobile devices. Even
though processing power and battery capacity are
improving, they still remain limited and valuable
and must not be employed excessively for
background added-value services giving priority to
voice calls. Other projects have also tackled such
problem: (Wang, 2009) proposes a system that
manages sensor duty cycles and reduces energy
consumption by shutting down unnecessary sensors.
The proposed method follows a similar approach,
but it also adds a window-based mechanism, which
represents the main contribution of the paper.
3 APPLICATIVE SCENARIO:
HEART FAILURE PATIENT
MONITORING
The Heart Failure (HF) is a chronic disease that
alternates intense and weak phases and requires
repeated and frequent hospital treatments. The use of
automatic instruments for a remote and ubiquitous
monitoring of biological parameters, relevant with
respect to the HF pathophysiology, offers new
perspectives to improve the patients’ life quality and
the efficacy of the applied clinical treatment. In more
details, HF is a disease represented by the limited
capacity of the heart to provide a sufficient blood
flow needed to meet all the body’s necessities. HF
usually causes a significant quantity of symptoms
such as shortness of breath, weight gain due to
excessive fluids, leg swelling, and exercise
intolerance. This illness condition can be diagnosed
with echocardiography and blood tests and the
consequent treatment commonly consists of
continuous lifestyle measures, drug therapy or, in
very critical cases, surgery.
Currently, in medical practice, well-known
patients’ management models are focused on
“manually handled” remote monitoring approaches:
nurses daily interrogate, through a phone call,
patients about their weight and the physical activity
they have done. The achieved results of this practice
show that this continuous remote monitoring
approach improves the quality of life of these
patients, prevents the progression to HF advanced
stages and reduces the use of hospitalization.
The presented smartphone-based AR method
associates modern context-aware capability of the
recent smartphone platforms, obtained by
implementing specific algorithmic solutions, with the
any-time and any-where communication capability
commonly offered by them. This joint usage will
allow reducing the patients’ involvement in the
monitoring process without impacting its
effectiveness. In particular, the method proposed is
going to be applied in an real experimental campaign,
in cooperation with a medical staff.
4 THE PROPOSED ACTIVITY
RECOGNITION METHOD
The proposed activity recognition method is designed
to distinguish four different user activities: Sitting,
Standing, Walking and Running.
The algorithm periodically collects raw signals
SMARTPHONE-BASED USER ACTIVITY RECOGNITION METHOD FOR HEALTH REMOTE MONITORING
APPLICATIONS
201