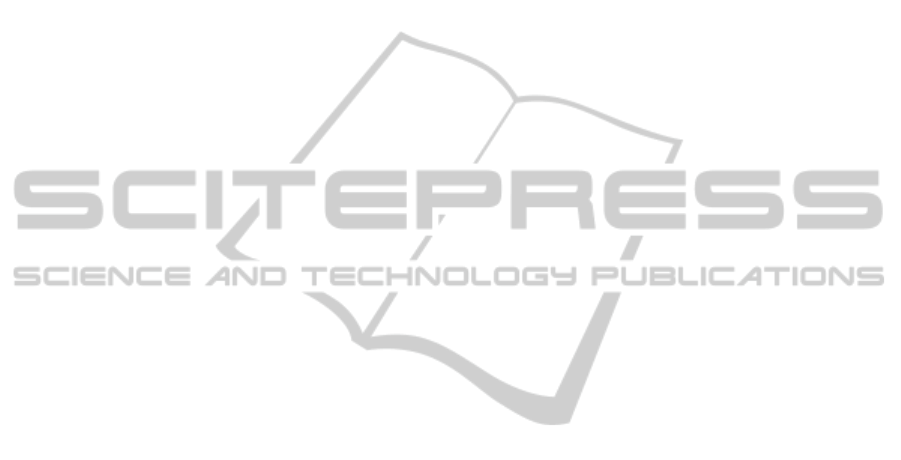
accurately detect learning style and increase learn-
ing performance when suitable teaching methods for
learning style are employed. Results of that study will
hopefully contribute to the quest of filling in the gap
of reliable empirical analysis in the area.
REFERENCES
Ballone, L. M. and Czerniak, C. M. (2001). Teachers’ be-
liefs about accommodating students’ learning styles in
science classes. Electronic Journal of Science Educa-
tion, 6:1–43.
Bousbia, N., Balla, A., and Rebai, I. (2009). Measuring
the learners’ learning style based on tracks analysis in
web based learning. In IEEE Symposium on Comput-
ers and Communications (ISCC’09), pages 98–103.
Cafferty, E. I. (1980). An analysis of student performance
based upon the degree of match between the educa-
tional cognitive style of the teacher and the educa-
tional cognitive style of the students. PhD thesis, The
University of Nebraska-Lincoln.
Chamberlin, M. T. (2011). The potential of prospective
teachers experiencing differentiated instruction in a
mathematics course. International Electronic Journal
of Mathematics Education, 6(3):134–156.
Coffield, F., Moseley, D., Hall, E., and Ecclestone, K.
(2004). Learning styles and pedagogy in post 16 learn-
ing: A systematic and critical review. Technical re-
port, Learning and Skills Research Centre / University
of Newcastle upon Tyne.
Dunn, R. and Griggs, S. A. (2003). Synthesis of the dunn
and dunn learning-style model research: Who, what,
when, where, and so what? Technical report, St.
Johns Universitys Center for the Study of Learning
and Teaching Styles.
Felder, R. M. and Silverman, L. K. (1988). Learning and
teaching styles in engineering education. Journal of
Engineering Education, 78(7):674–681.
Ford, N. and Chen, S. Y. (2001). Matching/mismatching
revisited: an empirical study of learning and teach-
ing styles. British Journal of Educational Technology,
32(1):5–22.
Graf, S. (2007). Adaptivity in Learning Management Sys-
tems Focussing on Learning Styles. PhD thesis, Vi-
enna University of Technology Faculty of Informatics.
Graf, S. and Kinshuk (2006). An approach for detect-
ing learning styles in learning management systems.
In International Conference on Advanced Learning
Technologies, pages 161–163. IEEE Computer Sci-
ence.
Gregorc, A. F. (1985). Style Delineator: a Self-Assessment
Instrument for Adults. Gregorc Associates Inc.
Kolb, A. Y. and Kolb, D. A. (2005). The kolb learning style
inventory - version 3.1. Technical report, Hay Group.
Kopsovich, R. D. (2001). A Study Of Correlations Between
Learning Styles Of Students And Their Mathematics
Scores On The Texas Assessment of Academic Skills
Test. PhD thesis, University of North Texas.
Manochehr, N. N. (2006). The influence of learning styles
on learners in e-learning environments: An empirical
study. Computers in Higher Education Economics Re-
view, 18(1):10–14.
Melis, E., Andres, E., Bdenbender, J., Frischauf, A.,
Goguadze, G., Libbrecht, P., Pollet, M., and Ullrich,
C. (2001). Activemath: A generic and adaptive web-
based learning environment. International Journal of
Artificial Intelligence in Education, 12:385–407.
Moallem, M. (2007). Accommodating individual differ-
ences in the design of online learning environments:
A comparative study. Journal of Research on Tech-
nology in Education, 40(2):217–245.
Papanikolaou, K. A. and Grigoriadou, M. (2004). Ac-
commodating learning style characteristics in adap-
tive educational hypermedia systems. In Individual
Differences in Adaptive Hypermedia Workshop at the
Third International Conference on Adaptive Hyper-
media and Adaptive Web based systems.
Papanikolaou, K. A., Grigoriadou, M., Kornilakis, H., and
Magoulas, G. D. (2003). Personalizing the interac-
tion in a web-based educational hypermedia system:
the case of inspire. User Modeling and User-Adapted
Interaction, 13(3):213–267.
Riding, R. and Agrell, T. (1997). The effect of cognitive
style and cognitive skills on school subject perfor-
mance. Educational Studies, 23(2):311–323.
Riding, R. and Grimley, M. (1999). Cognitive style and
learning from multimedia materials in 11-year chil-
dren. British Journal of Educational Technology,
30(1):43–59.
Riding, R. J. and Rayner, S. (1998). Cognitive styles and
learning strategies: understanding style differences in
learning behaviour. David Fulton Publishers.
Ritter, S., Anderson, J. R., Koedinger, K. R., and Corbett,
A. (2007). Cognitive tutor: Applied research in math-
ematics education. Psychonomic Bulletin and Review,
14(2):249–255.
Ross, J. and Schulz, R. (1999). Can computer-aided in-
struction accommodate all learners equally? British
Journal of Educational Technology, 30(1):5–24.
Sarrafzadeh, A., Alexander, S., Dadgostar, F., Fan, C., and
Bigdeli, A. (2008). “how do you know that i don’t un-
derstand?” a look at the future of intelligent tutoring
systems. Computers in Human Behavior, 24(4):1342–
1363.
Shrestha, C. H., Gipps, C., Edirisingha, P., and Linsey, T.
(2007). Learning and e-learning in he: the relationship
between student learning style and vle use. Research
Papers in Education, 22(4):443–464.
Soloman, B. and Felder, R. (2001). Index of learning
style questionnaire. Retrieved on 21.11.2011 from
http://www.engr.ncsu.edu/learningstyle/ilsweb.html.
Vermunt, J. D. (1998). The regulation of constructive learn-
ing processes. British Journal of Educational Psy-
chology, 68(2):149–171.
Zin, N. A. M. (2009). A-maths multimedia courseware for
effective mathematic learning: Matching instructions
to student’s learning style. Journal of Applied Sci-
ences, 9(8):1510–1516.
CSEDU2012-4thInternationalConferenceonComputerSupportedEducation
322