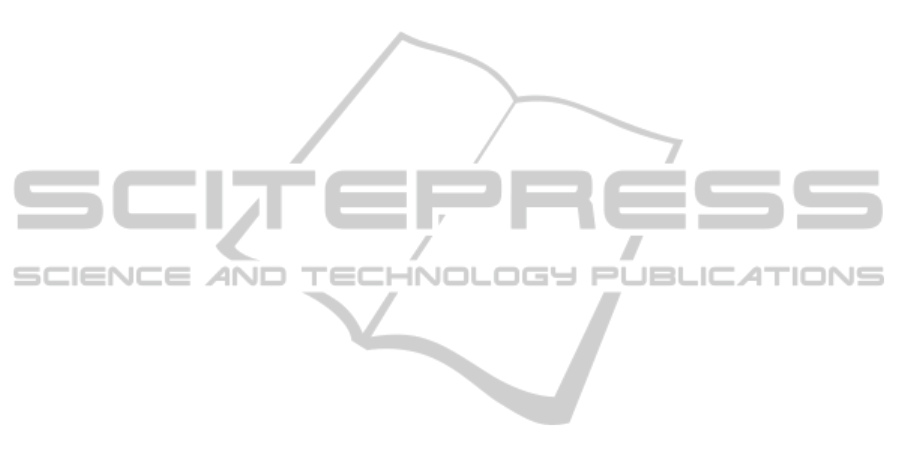
timates. The results also suggest that the coefficient
α is useful for altering initial scores. Despite of these
promising results, our study is not without shortcom-
ings, i.e., the small size of the ontology as well as the
small amount of scenarios tested so far. However, a
strong point is certainly the empirical assessment by
means of professors, lecturers and post-docs teaching
programming courses at university.
5 CONCLUSIONS
We proposed a novel algorithm to propagate expertise
scores in an ontology overlay model based on con-
strained spreading activation and relative depth scal-
ing. We compared the algorithm’s performance with
a baseline. 29 experts qualified calculated expertise
scores given various scenarios. Thereby, our algo-
rithm outperforms the baseline approach in half of
the test scenarios. For the remaining scenarios both
algorithms propagate almost equally. These results
suggests that the calculation of a learner’s expertise
utilizing constrained spreading activation and relative
depth scaling can lead to more accurate learner mod-
els. Future work may consider multi-inheritance of
topics as well as the integration of additional relation
types like the part-of relationship.
REFERENCES
Anderson, J. (1983). A spreading activation theory of mem-
ory. Journal of verbal learning and verbal behavior,
22(3):261–295.
Billig, A., Blomqvist, E., and Lin, F. (2010). Semantic
matching based on enterprise ontologies. On the Move
to Meaningful Internet Systems 2007: CoopIS, DOA,
ODBASE, GADA, and IS, pages 1161–1168.
Brusilovsky, P. and Mill´an, E. (2007). User models for
adaptive hypermedia and adaptive educational sys-
tems. The adaptive web, pages 3–53.
Bull, S. and Kay, J. (2010). Open learner models. Advances
in Intelligent Tutoring Systems, pages 301–322.
Cantador, I., Szomszor, M., Alani, H., Fern´andez, M., and
Castells, P. (2008). Enriching ontological user profiles
with tagging history for multi-domain recommenda-
tions. In 1st Int. Workshop on Collective Intelligence
and the Semantic Web (CISWeb 2008).
Carr, B. and Goldstein, I. (1977). Overlays: A theory
of modelling for computer aided instruction. Artifi-
cial Intelligence Memo 406, Massachusetts Institute
of Technology, Cambridge, Massachusetts.
Cohen, P. and Kjeldsen, R. (1987). Information re-
trieval by constrained spreading activation in seman-
tic networks. Information processing & management,
23(4):255–268.
Crestani, F. (1997). Application of spreading activation
techniques in information retrieval. Artificial Intelli-
gence Review, 11(6):453–482.
Kay, J. and Lum, A. (2005a). Exploiting readily available
web data for scrutable student models. In Proceedings
of the 2005 conference on Artificial Intelligence in Ed-
ucation: Supporting Learning through Intelligent and
Socially Informed Technology, pages 338–345. IOS
Press.
Kay, J. and Lum, A. (2005b). Ontology-based user mod-
elling for the semantic web. In Proceedings of the
Workshop on Personalisation on the Semantic Web:
Per-SWeb05, pages 15–23.
Liu, H. and Maes, P. (2005). Interestmap: Harvesting social
network profiles for recommendations. In Beyond Per-
sonalization - IUI 2005, San Diego, California, USA.
Liu, W., Weichselbraun, A., Scharl, A., and Chang, E.
(2005). Semi-automatic ontology extension using
spreading activation. Journal of Universal Knowledge
Management, 1:50–58.
Manouselis, N., Drachsler, H., Vuorikari, R., Hummel, H.,
and Koper, R. (2011). Recommender systems in tech-
nology enhanced learning. Recommender Systems
Handbook, pages 387–415.
Pirr`o, G. (2009). A semantic similarity metric combin-
ing features and intrinsic information content. Data
Knowl. Eng., 68(11):1289–1308.
Schickel-Zuber, V. and Faltings, B. (2007). Oss: a semantic
similarity function based on hierarchical ontologies.
In Proceedings of the 20th international joint confer-
ence on Artifical intelligence, pages 551–556.
Sieg, A., Mobasher, B., and Burke, R. (2007). Web search
personalization with ontological user profiles. In Pro-
ceedings of the sixteenth ACM conference on Con-
ference on information and knowledge management,
pages 525–534. ACM.
Sussna, M. (1993). Word sense disambiguation for free-
text indexing using a massive semantic network. In
Proceedings of the second international conference on
Information and knowledge management, pages 67–
74. ACM.
CSEDU2012-4thInternationalConferenceonComputerSupportedEducation
180