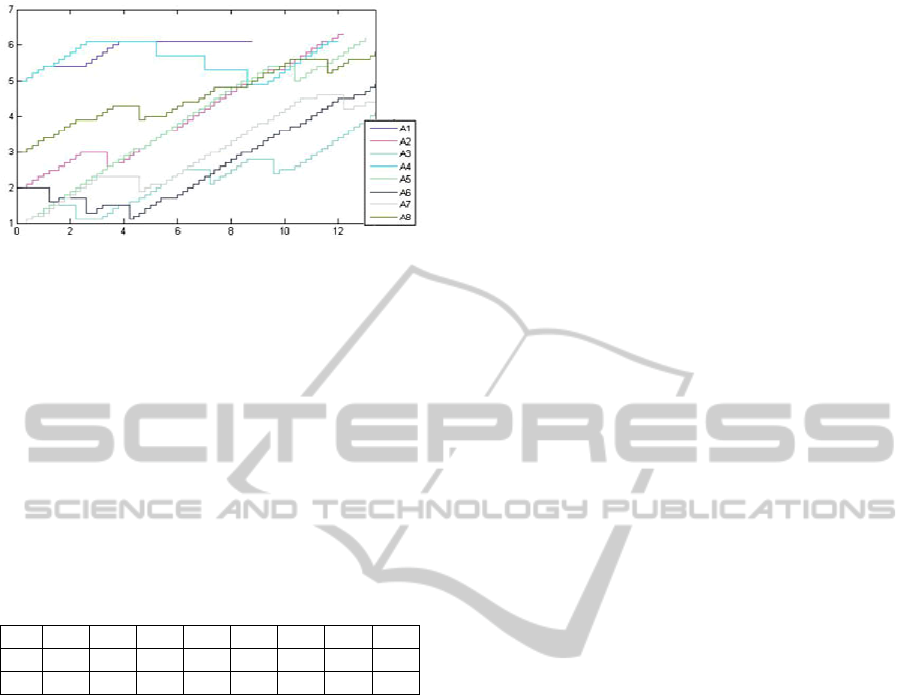
Figure 2: Q&A model of Collaborative Learning.
slight improvement can be observed in the
established period of time, whilst in the second one,
peer-to-peer interaction appears to be the best
method to boost competence.
Even in the worst case scenario, a week without
any activity, the overall results at the end of a 12
weeks semester were encouraging.
Table 1 presents an example of the increase in
competences (points gained), during 12 weeks, for
both of the above models, one subject, one set of
simulations.
Table 1: Competences gained during 12 weeks in the
classical (C), and in the collaborative learning (CL) model.
A1 A2 A3 A4 A5 A6 A7 A8
C 0,8 0,5 1.6 0,7 0,8 0,7 0,7 0,8
CL 1,1 4,3 3,1 1,1 5,0 3,5 3,6 5,1
3 FINAL COMMENTS
In recent years studies have offered constant proof
of the impact collaborative learning has on the way
students accumulate knowledge. Peer-to-peer
learning appears to succeed where classic tutor input
fails or faces resistance. The Q&A model proposed
in this paper seeks to increase knowledge by
emphasizing the importance of questions and
answers among peers in online social networks, a
process sustained and enhanced by tutors.
Simulations compared two models: the classic
model, where students ask and teachers answer, and
proposed one, where students ask and peers answer.
Simulations showed the increase in knowledge was
more significant in the latter case.
Expanding the network, allowing network
friends from different classes, and even from outside
school could only lead to an increasing Q&A
activity, to more questions and faster answers,
lowering thus the necessity for tutors to intervene, in
order to stimulate the answering process.
Furthermore agents will be used to speed up the
question answering, by automatically answering
questions they have answers for in a special Q&A
list (consisting of questions and answers approved or
given by tutors) and by suggesting contacts (other
agents) that could answer question from a certain
field (using the Learner Competence Vector).
ACKNOWLEDGEMENTS
This paper was supported by the project
"Development and support of multidisciplinary
postdoctoral programmes in major technical areas of
national strategy of Research - Development -
Innovation" 4D-POSTDOC, contract no. POSDRU/
89/1.5/S/52603, project co-funded by the European
Social Fund through Sectorial Operational Progra-
mme Human Resources Development 2007-2013,
and the Spanish MICINN contract IPT-430000-
2010-13 project Social powered Agents for Know-
ledge search Engines (SAKE).
REFERENCES
Allen, A. R., Boraks, N., 1978. Peer Tutoring - Putting it
to Test, Reading Teacher, vol. 32, no. 3, pp. 274-278.
Bloom, B. S., Krathwohl, D. R., 1956. Taxonomy of
Educational Objectives: The Classification of
Educational Goals. Handbook I: Cognitive Domain,
Longmans.
Chi, E. H., 2009. Information seeking can be social,
Computer, vol. 42, pp. 42–46.
Glasser, W., 1986. Control Theory in the Classroom,
Perennial Library, New York.
Morris, M. R., Teevan, J., Panovich, K., 2010. What do
people ask their social networks, and why?: a survey
of status message Q&Abehavior, 28th Intl.Conf.
Human factors in Comp. Sys
Mitra, S., 2003. Minimally invasive education: a progress
report on the “hole-in-the-wall” experiments, British J.
of Educational Tech., vol. 34, no. 3, pp. 367-371.
Mitra, S., Dangwal, M., 2010. Limits to self-organising
systems of learning — the Kalikuppam experiment,
British J. of Educational Technology, vol. 41, no. 5,
pp. 672-688.
Moreno, A., de la Rosa, J. L, Szymanski, B. K., Barcenas,
J. M., 2009. Reward System for Completing FAQs,
Artificial Int. Research and Dev., Vol. 202, pp. 361-
370
Pous, C., de la Rosa, J. L., Vehi, J., Melendez, J.,
Colomer, J., 1997. Anàlisi de Plans d'Estudi amb
Enginyeria de Sistemes, Innovació en educació,
pp.149-182
Trias, A., de la Rosa, J. L, Galitsky, B., Dobrocsi, G.,
2010. 'Automation of social networks with QA agents,
Proc. of the 9
th
International Conference on
CSEDU2012-4thInternationalConferenceonComputerSupportedEducation
334