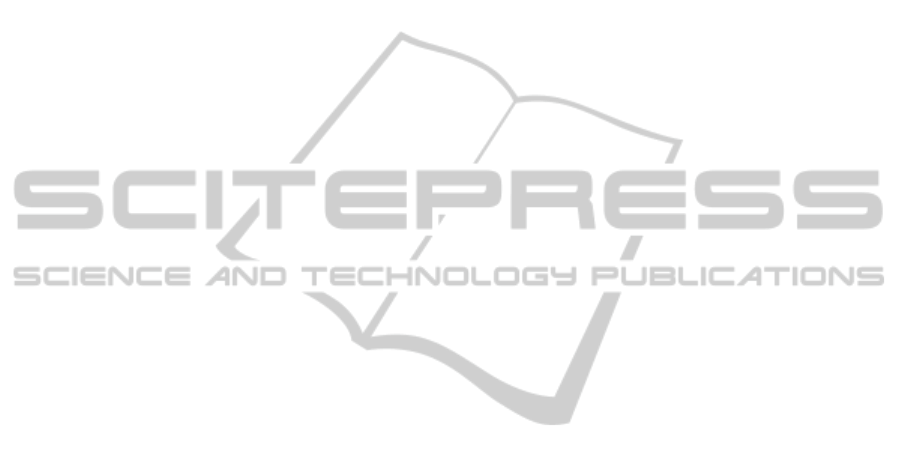
and successful 3D MULE student engagement, we
must identify and implement policies for student
self-regulation and 3D MUVE system management.
Thus, we validate our research hypotheses on
considering self-regulation and environment
management of 3D MUVE as a prime factor for 3D
MULE management policy considerations.
The environment management indicated the
highest positive impact on increasing the student
engagement. Although, students entertain
themselves by various environment engagements
they also felt the difficulty of task coordination and
unsupportive avatar behaviour during learning
engagements. For example, lecture displays reset
whenever an avatar hits the play button, disturbing
the other viewers. Also, if a student’s simulation
arrangement is too close to another’s setup,
simulation interferences were observed. These can
be easily solved if we implement suitable
environment management policy considerations.
Considering the student opinions and our
observations, we suggest the importance of cohesive
learning activities with 3D MULE user engagement
aspects through constructive alignment (Biggs,
1996). This would enable students to spend their
time highly engaged with the 3D MULE, while
achieving their learning goals comfortably.
Careful analyses and tests were employed to
minimise the impact of the following limitations.
Due to the nature of the research, the study sample
was limited to a particular set of students. These
students have provided their feedback and answers
based on their experiences, which we validated
through observation. Therefore, we consider the data
received as accurate and conclusive though the study
had a relatively small sample, due to resource
constraints. The questions used were appropriately
designed, although they have yet to be examined for
psychometric measures. It is a challenge to find a
widely accepted standard set of psychometric
measures in particular for 3D MULE, as the field of
study is still growing. We would welcome the
researchers to consider this aspect in future research.
6 CONCLUSIONS
3D MULE provide a great potential for engaging
students in innovative, immersive learning
environments. Use of policy considerations for 3D
MULE learning management facilitates the students
and teachers in many ways. As we have
comprehensively shown, students would have
benefited by having a supportive and managed 3D
learning environment, while allowing teachers to
focus more on the educational value of learning
tasks than worrying about the challenges they face
with 3D MULE. Policy based management of 3D
MULE is essential as the situational approaches for
utilizing 3D MUVE for learning would not
otherwise be sustainable. We have presented the two
important aspects identified for policy
considerations through empirical evidence, which
were also validated by previous research. With that
we look forward to facilitating the policy
consideration development through user guidance.
REFERENCES
Allison, C., Miller, A., et al. (2008), Exploratory Learning
for Computer Networking, ICWL, 331-342
Allison C., Miller A., et al., (2010), Educationally
Enhanced Virtual Worlds, 40
th
ASEE/IEEE Frontiers
in Education (FIE), IEEE Press, T4F-1–T4F-6
Allison, C., Miller A., et al., (2011), The Third Dimension
in Open Learning, 41
st
ASEE/IEEE Frontiers in
Education, IEEE Press, T2E-1 – T2E-6
Biggs, J. B. (1996), Enhancing teaching through
constructive alignment, Higher Education, 32(3):347-
364
Costello, A. B. & Osborne, J. W., (2005), Best practices in
exploratory factor analysis: four recommendations for
getting the most from your analysis, Practical
Assessment, Research & Evaluation, 10(7):1-9
Dalgarno, B., et al., (2009), Effectiveness of a Virtual
Laboratory as a preparatory resource for Distance
Education chemistry students, Computers &
Education, 53(3):863-865,
Field A., (2006), Discovering Statistics using SPSS, 2nd
Ed., SAGE, London
Getchell, K., et al. (2010), Games Methodologies and
Immersive Environments for Virtual Fieldwork, IEEE
Transactions on Learning Technologies, 3(4), 281-293
Girvan, C. & Savage, T. (2010), Identifying an appropriate
pedagogy for virtual worlds: A Communal
Constructivism case study, Comp. & Edu., 55,342-349
Kolb, D. A., Boyatzis, R. E. and Mainemelis, C., (2001),
Experiential Learning Theory: Previous Research and
New Directions, J. Sternberg and L. F. Zhang, (Eds):
In Perspectives on Thinking, Learning and Cognitive
Styles, Lawrence Erlbaum: Mahwah, 227
Linden Labs (2003), Second Life, http://www.secondlife.
com
McCaffery J., et al., (2011), Extending the use of virtual
worlds as an educational platform - Network Island:
An advanced learning environment for teaching
Internet routing algorithms, 3rd CSEDU, INSTICC, 1
Perera, I., Allison, C., McCaffery J., and Miller A. (2011),
Towards Effective Blended Learning with 3D MUVE
- An Analysis of Use Case Implementations for 3D
MUVE Learning, 3rd CSEDU2011, 2, 46-55.
CSEDU2012-4thInternationalConferenceonComputerSupportedEducation
198