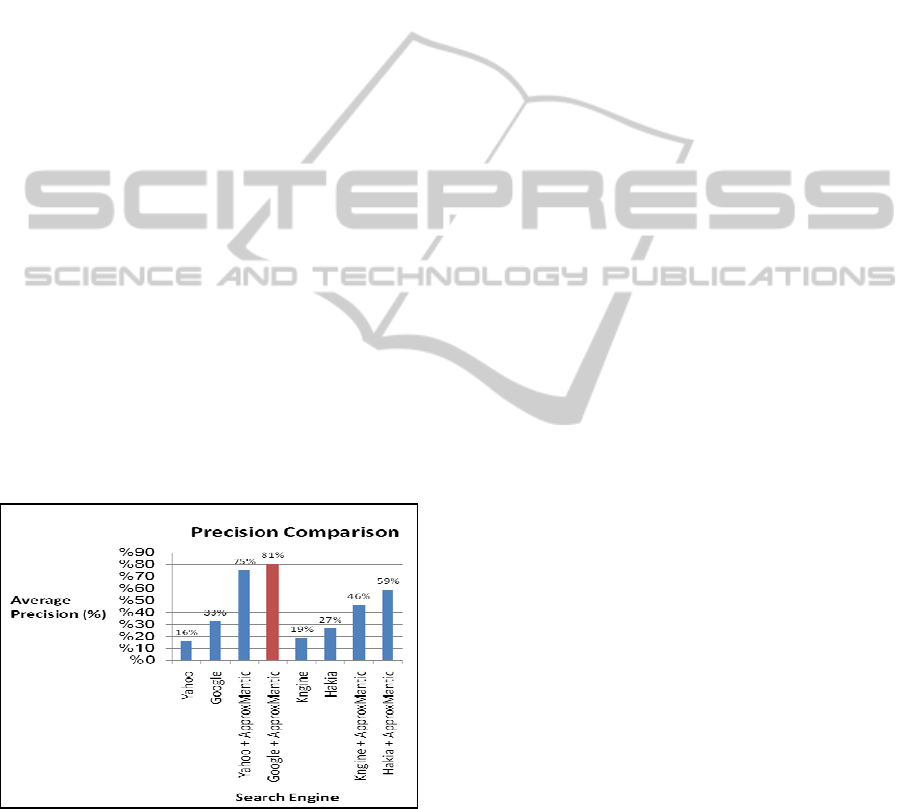
reformulations for each original query. Here are the
generated reformulated queries:
Reformulations (some):
Kindle “super deal” “Buy now”
Kindle “bargain” “add to cart”
Kindle “floor model” “Buy web only”
…etc.
Finally the reformulated queries are passed to a
search engine to retrieve results.
3 EXPERIMENTS
We assess the improvement in search results by
observing the number of URLs returned in the result
and the percentage of URLs in the top 20 URLs
returned that are relevant to the user's query (top 20
results' precision).
We ran several categories of experiments. We
investigated our solution with keyword-based search
engines, such as Google and Yahoo. We also
experimented against semantic-based search
engines, such as Kngine and Hakia (Manuja and
Garg, 2011).
The results obtained from our experiments show
that our solution enhances both the results retrieved
by the keyword-based search engines as well as by
the semantic-based search engines. The best result
achieved was when our solution was integrated with
Google search engine. Figure 5, shows summary of
top 20 result precision.
Figure 5: Summary of top 20 result precision.
4 CONCLUSIONS
In this paper we have introduced a novel technique
for approximating search engine user's intention that
relies on query reformulations. Our solution utilizes
a special domain specific ontology that models how
data on the internet is organized. Such ontology
enables encoding and processing of query
reformulation rules. Our experiments confirm that
the proposed solution is able to generate highly
relevant query transformations that deliver better
results than that obtained from traditional term-
based query engines and even that of selected
semantic query engines.
REFERENCES
Allon, O. (2009). Two new improvements to Google
results pages. Retrieved on December 7
th
, 2011. from
http://googleblog.blogspot.com/2009/03/two new-
improvements-to-google-results.html.
Awad, A. (2012). Approximating User’s Intention for
Search Engine Queries. M.Sc. Thesis. College of
Computing & I.T., Arab Academy for Science,
Technology & Maritime Transport, Alexandria, Egypt.
Maedche, A., Staab, S., Stojanovic, N., Studer, R., Sure,
Y. (2001). SEAL - a framework for developing
semantic web portals. 18th British National
Conference on DB, pages. 1–22.
Manuja, M. and Garg, D. (2011). Semantic Web Mining
of Un-structured Data: Challenges and Opportunities.
International Journal of Engineering, CSC Press,
Volume (5): Issue (3), pages 268-276.
Mostafa, L., Farouk, M., Fakhary, M. (2009). An
Automated Approach for Web Page Classification.
19th International Conference on Computer Theory
and Application, Alexandria, Egypt.
Salton, G., and Buckley, C. 1988. On the use of spreading
activation methods in automatic information. 11th
annual international ACM SIGIR conference on
Research and Development in Information Retrieval,
pages 147–160.
Shirazi, H. M., Shirazi, M. M. and Fardroo, N. (2009).
Discovering User Interest by Ontology-based User
Profile. Int. Journal of Intelligent Information
Technology application, Engineering Technology
Press. Volume 2 [1].
Surdeanu, M., Ciaramita, M. and Zaragoza, H. (2008).
Learning to Rank Answers on Large Online QA
Collections. ACL, OH, pages 719-727.
Verberne, S., Boves, L., Oostdijk, N. and Coppen, P.
(2010). What Is Not in the Bag of Words for Why-
QA? ACL, pages 719-727.
APPROXIMATINGUSER'SINTENTIONFORSEARCHENGINEQUERIES
425