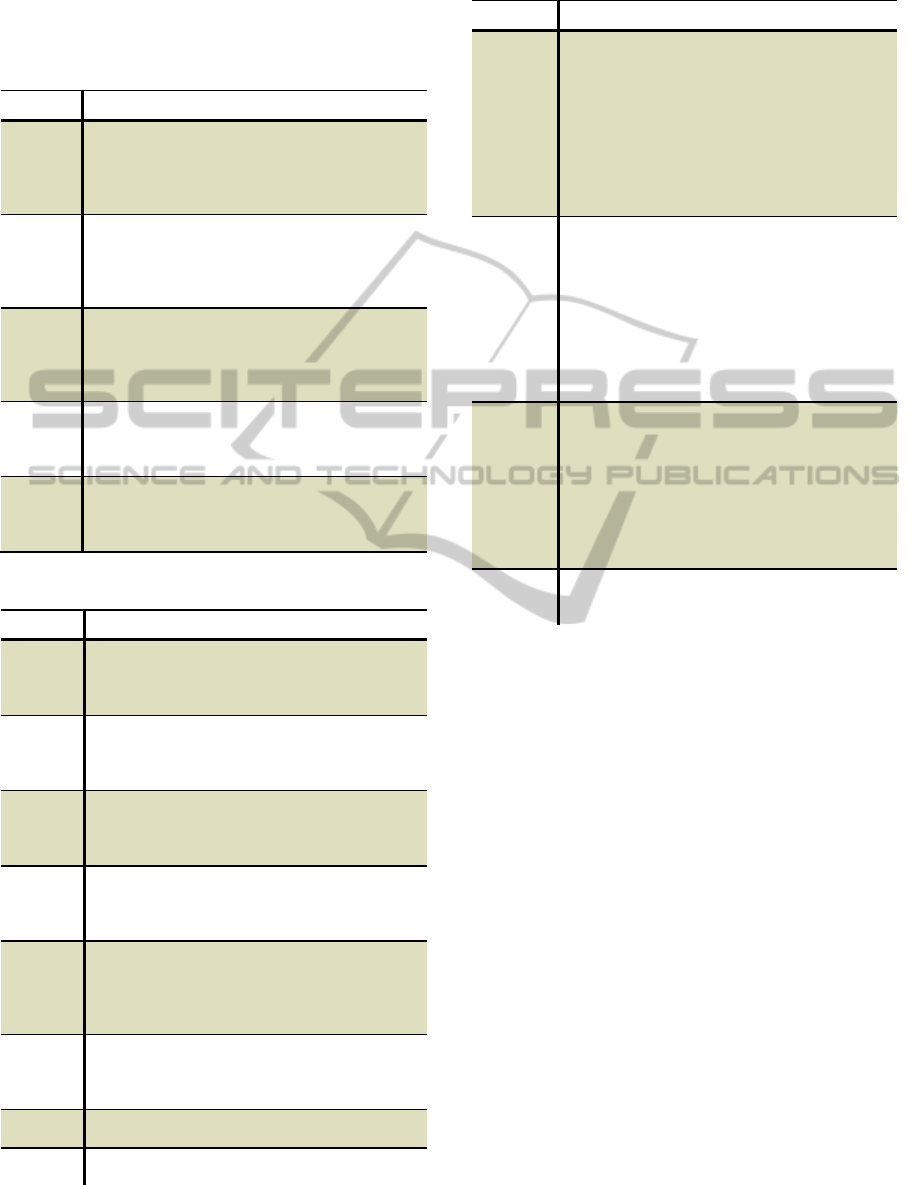
granules and concepts. To facilitate the analysis, we
highlighted the cells with the greatest similarity
measures.
Table 2: The 5 clusters/granules of corpus 1.
GRANULE
SUBJECT
KEYWORDS
1
genetic
algorithm /
optimization
exploration, performance,
fitness, operator, member,
algorithm, convergence,
solution, population, optimum,
crossover
2
neural networks
extension, input, example,
property, regression, analysis,
neuron, procedure, realization,
synthesis, vector, coefficient,
manner, applicability
3
data mining /
knowledge
management
user, technique, topic, storage,
knowledge, management,
capability, information,
methodology, data, business,
database
4
cognition /
logic
behavior, theory, life, paradigm,
language, computer, principle,
aspect, manipulation,
intelligence
5
cognition
protocol, difference, relations,
complexity, analysis, problem,
role, system, cognition, method,
application
Table 3: The 8 clusters/granules of corpus 2.
GRANULE
SUBJECT
KEYWORDS
01
semantic
evolution, entity, library,
management, language,
technology, ontology, domain,
description, semantics
02
latent
semantic
analysis
subspace, combination, detection,
decomposition, association,
retrieval, matrix, effectiveness,
vector, collection
03 clustering
example, prototype, constraint,
tendency, algorithm, objective,
possibility, principle, data,
problem,
04
information
retrieval
period, kind, property, relations,
decomposition, retrieval,
information, expansion, criterion,
construction
05
concept
extraction
extension, representation,
evaluation, concept, strategy,
selection, explanation, logic,
interpretation, identification, text,
baseline
06 ontology
mechanism, classifier, correlation,
thesaurus, creation, ontology,
context, integration, recognition,
source, module.
07
fuzzy
relations
membership, co-occurrence, set,
binary
08 topic models
probability, language, processing,
mixture, model, generator
Table 4: The 4 clusters/granules of corpus 2.
GRANULE
SUBJECT
KEYWORDS
01
semantic/
ontology
development, evolution, entity,
library, management, language,
version, technology, ontology,
methodology, domain,
description, semantics, input,
mechanism, classifier,
correlation, thesaurus, creation,
ontology, context, integration,
identification, recognition,
source, module.
02
latent semantic
analysis/
concept
extraction
item, user, basis, subspace,
combination, detection,
decomposition, association,
retrieval, matrix, effectiveness,
vector, collection, method,
extension, representation,
evaluation, concept, strategy,
selection, explanation, addition,
logic, interpretation,
identification, text, baseline
03
clustering/
information
retrieval
example, prototype, constraint,
tendency, algorithm, objective,
possibility, finding, principle,
data, problem, difficulty, period,
user, minimum, kind, property,
relations, decomposition,
retrieval, information,
expansion, criterion, method,
construction
04 topic models
probabilistic, language,
processing, mixture, model,
generative
7 RESULTS
Looking through Tables 1 and 3, the proposed
technique combines words significant enough to
present the topics in each corpus. In corpus 1, for
computational intelligence, 7 topics are easily
identified from the words associated with their
clusters/granules. In corpus 2, on text mining /
information retrieval, we achieved better results,
because the eight subjects that make up the corpus
are easily identified. The results presented in Tables
2 and 4 show that the technique performs well
against the ability of granule generalization
contained in the corpus.
With respect to corpus 1 which was tested, we
give special emphasis to the grouping of words that
describe the topics of genetic
algorithms/optimization and data mining/ knowledge
management. Such topics are strongly related. The
proposed technique shows consistency since it
captures these relationships by grouping the words
contained in their respective documents. LSA
identified 13 clusters of words for corpus 1 text and
10 clusters for corpus 2.
WEBIST2012-8thInternationalConferenceonWebInformationSystemsandTechnologies
686