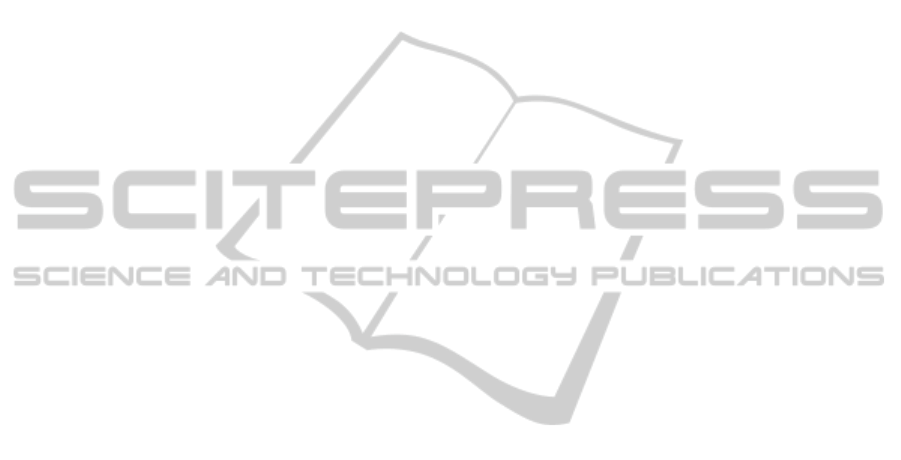
users that should be taken more into consideration
when predictions have to be suggested. Serendipity
and negative preferences are further factors that mu-
sic recommenders should include in their predictive
analysis (Iaquinta et al., 2008; Musto et al., 2011).
REFERENCES
Acampora, G., Gaeta, M., and Loia, V. (2010a). Exploring
e-learning knowledge through ontological memetic
agents. Comp. Intell. Mag., 5:66–77.
Acampora, G., Gaeta, M., Loia, V., and Vasilakos, A. V.
(2010b). Interoperable and adaptive fuzzy services for
ambient intelligence applications. ACM Trans. Auton.
Adapt. Syst., 5:8:1–8:26.
Biancalana, C., Flamini, A., Gasparetti, F., Micarelli, A.,
Millevolte, S., and Sansonetti, G. (2011a). Enhanc-
ing traditional local search recommendations with
context-awareness. In Konstan, J. A., Conejo, R.,
Marzo, J. L., and Oliver, N., editors, User Model-
ing, Adaption and Personalization - 19th International
Conference, UMAP 2011, Girona, Spain, July 11-15,
2011. Proceedings, volume 6787 of Lecture Notes in
Computer Science, pages 335–340. Springer.
Biancalana, C., Gasparetti, F., Micarelli, A., Miola, A., and
Sansonetti, G. (2011b). Context-aware movie recom-
mendation based on signal processing and machine
learning. In Proceedings of the 2nd Challenge on
Context-Aware Movie Recommendation, CAMRa ’11,
pages 5–10, New York, NY, USA. ACM.
Breese, J. S., Heckerman, D., and Kadie, C. M. (1998). Em-
pirical analysis of predictive algorithms for collabora-
tive filtering. In Cooper, G. F. and Moral, S., editors,
Proceedings of the 14
th
Conference on Uncertainty in
Artificial Intelligence, pages 43–52.
Celma, . (2010). Music Recommendation and Discovery -
The Long Tail, Long Fail, and Long Play in the Digital
Music Space. Springer.
Gabor, D. (1946). Theory of communication. J. Inst. Elect.
Eng., 93:429–457.
Gaeta, A., Gaeta, M., and Ritrovato, P. (2009). A grid based
software architecture for delivery of adaptive and per-
sonalised learning experiences. Personal Ubiquitous
Comput., 13:207–217.
Gasparetti, F. and Micarelli, A. (2007). Personalized search
based on a memory retrieval theory. International
Journal of Pattern Recognition and Artificial Intel-
ligence (IJPRAI): Special Issue on Personalization
Techniques for Recommender Systems and Intelligent
User Interfaces, 21(2):207–224.
Groh, G. and Ehmig, C. (2007). Recommendations in taste
related domains: collaborative filtering vs. social fil-
tering. In GROUP ’07: Proceedings of the 2007 inter-
national ACM conference on Supporting group work,
pages 127–136, New York, NY, USA. ACM.
Iaquinta, L., de Gemmis, M., Lops, P., Semeraro, G., Fi-
lannino, M., and Molino, P. (2008). Introducing
serendipity in a content-based recommender system.
In Xhafa, F., Herrera, F., Abraham, A., K¨oppen, M.,
and Ben´ıtez, J. M., editors, International Conference
on Hybrid Intelligent Systems (HIS 2008, pages 168–
173. IEEE Computer Society.
Micarelli, A., Gasparetti, F., and Biancalana, C. (2006).
Intelligent search on the internet. In Stock, O. and
Schaerf, M., editors, Reasoning, Action and Inter-
action in AI Theories and Systems, volume 4155 of
Lecture Notes in Computer Science, pages 247–264.
Springer.
Micarelli, A., Sciarrone, F., and Gasparetti, F. (2009). A
case-based approach to adaptive hypermedia naviga-
tion. IJWLTT, 4(1):35–53.
Musto, C., Semeraro, G., Lops, P., and de Gemmis, M.
(2011). Random indexing and negative user prefer-
ences for enhancing content-based recommender sys-
tems. In Huemer, C. and Setzer, T., editors, E-
Commerce and Web Technologies, volume 85 of Lec-
ture Notes in Business Information Processing, pages
270–281. Springer.
Resnick, P., Iacovou, N., Suchak, M., Bergstrom, P., and
Riedl, J. (1994). Grouplens: an open architecture
for collaborative filtering of netnews. In Proceedings
of the 1994 ACM conference on Computer supported
cooperative work, CSCW ’94, pages 175–186, New
York, NY, USA. ACM.
Schafer, J. B., Frankowski, D., Herlocker, J., and Sen, S.
(2007). Collaborative filtering recommender systems.
In Brusilovsky, P., Kobsa, A., and Nejdl, W., edi-
tors, The Adaptive Web: Methods and Strategies of
Web Personalization, volume 4321 of Lecture Notes
in Computer Science, pages 291–324. Springer Berlin,
Heidelberg, Berlin, Heidelberg, and New York.
Semeraro, G., Lops, P., Basile, P., and de Gemmis, M.
(2009). Knowledge infusion into content-based rec-
ommender systems. In Bergman, L. D., Tuzhilin, A.,
Burke, R. D., Felfernig, A., and Schmidt-Thieme, L.,
editors, Proceedings of the 2009 ACM Conference on
Recommender Systems, RecSys 2009, pages 301–304.
ACM.
Shardanand, U. and Maes, P. (1995). Social information fil-
tering: algorithms for automating word of mouth. In
Proceedings of the SIGCHI conference on Human fac-
tors in computing systems, CHI ’95, pages 210–217,
New York, NY, USA. ACM Press/Addison-Wesley
Publishing Co.
WEBIST2012-8thInternationalConferenceonWebInformationSystemsandTechnologies
402