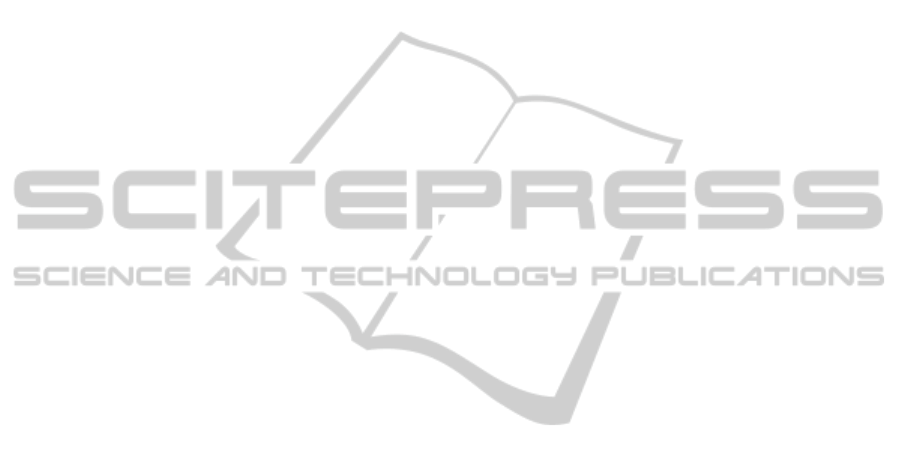
5 CONCLUSIONS
We have presented two visions of region growing.
The first one can easily deal with multidimensional
data in the feature space and specify locally adaptive
segmentation. The second one leverages the
powerful mathematical tools of variational
framework. One major advantage is to bring
convergence properties through the minimization of
the energy functional.
Various approaches derived from both
formalisms have been successfully applied to life
imaging, yielding quite satisfying results while
enabling simple initializations, intuitive interactions
and easy understanding of tuning parameters by
users. From our knowledge, these two formalisms
should encompass whatever region growing
approaches proposed in the literature.
ACKNOWLEDGEMENTS
The authors thank the ESRF ID19 group for help
during data acquisition and Pr. M Lafage-Proust
(Inserm U890, St Etienne, France) for providing the
bones samples.
REFERENCES
Adams R, Bischof L, 1994. Seeded region growing. IEEE
Transactions on Pattern Analysis and Machine
Intelligence,
16, 641-7.
Boykov Y Y, Jolly M P, 2001. Interactive graph cuts for
optimal boundary and region segmentation of objects
in N-D images. In:
8th IEEE International Conference
on Computer Vision, Vol I, Proceedings
, 105-12.
Chan T, Zhu W, 2005. Level set based shape prior
segmentation. In:
Proceedings - 2005 IEEE Computer
Society Conference on Computer Vision and Pattern
Recognition, CVPR 2005
, 1164-70.
Chan T F, Vese L A, 2001. Active contours without edges.
IEEE Transactions on Image Processing, 10, 266-77.
Chuang C H, Lie W N, 2001. Region growing based on
extended gradient vector flow field model for multiple
objects segmentation. In:
IEEE International
Conference on Image Processing
, 74-7.
Comaniciu D, Meer P, 2002. Mean shift: A robust
approach toward feature space analysis. IEEE
Transactions on Pattern Analysis and Machine
Intelligence,
24, 603-19.
Cremers D, Sochen N, Schnörr C, 2003. Towards
recognition-based variational segmentation using
shape priors and dynamic labeling.
Scale Space
Methods in Computer Vision,
2695/2003, 388-400.
Dehmeshki J, Ye X, Costello J, 2003. Shape based region
growing using derivatives of 3D medical images:
Application to semi-automated detection of pulmonary
nodules. In: IEEE International Conference on Image
Processing
, 1085-8.
Fan J, Yau D K Y, Elmagarmid A K, Aref W G, 2001.
Automatic image segmentation by integrating color-
edge extraction and seeded region growing.
IEEE
Transactions on Image Processing,
10, 1454-66.
Foulonneau A, Charbonnier P, Heitz F, 2006. Affine-
invariant geometric shape priors for region-based
active contours.
Pattern Analysis and Machine Intelli-
gence, IEEE Transactions on,
28, 1352-7.
Frangi A F, Niessen W J, Vincken K L, Viergever M A,
1998. Multiscale vessel enhancement filtering. In:
Medical Image Computing and Computer-Assisted In-
tervention - Miccai'98
, 130-7.
Freedman D, Zhang T, 2004. Active contours for tracking
distributions. IEEE Transactions on Image
Processing,
13, 518-26.
Gastaud M, Barlaud M, Aubert G, 2004. Combining shape
prior and statistical features for active contour
segmentation.
Circuits and Systems for Video
Technology, IEEE Transactions on,
14, 726-34.
Grenier T, Revol-Muller C, Costes N, Janier M, Gimenez
G, 2005. Automated seeds location for whole body
NaF PET segmentation.
IEEE Trans. Nuc. Sci., 52,
1401-5.
Grenier T, Revol-Muller C, Costes N, Janier M, Gimenez
G, 2007. 3D robust adaptive region growing for
segmenting [18F] fluoride ion PET images. In: IEEE
Nuclear Science Symposium Conference Record
,
2644-8.
Jehan-Besson S, Barland M, Aubert G, 2003. DREAM2S:
Deformable regions driven by an Eulerian accurate
minimization method for image and video
segmentation.
International Journal of Computer
Vision,
53, 45-70.
Kass M, Witkin A, Terzopoulos D, 1987. Snakes: active
contour models. In: Proceedings - First International
Conference on Computer Vision.
, 259-68.
Lin Z, Jin J, Talbot H, 2000. Unseeded region growing for
3D image segmentation. ACM International Conferen-
ce Proceeding Series, 2000.
Malladi R, Sethian J A, Vemuri B C, 1995. Shape
modeling with front propagation: a level set approach.
IEEE Transactions on Pattern Analysis and Machine
Intelligence,
17, 158-75.
Mehnert A, Jackway P, 1997. Improved seeded region
growing algorithm.
Pattern Recognition Letters, 18,
1065-71.
Olabarriaga S D, Smeulders A W M, 2001. Interaction in
the segmentation of medical images: A survey.
Medical Image Analysis, 5, 127-42.
Pacureanu A, Revol-Muller C, Rose J L, Sanchez-Ruiz M,
Peyrin F, 2010. A Vesselness-guided Variational Se-
gmentation of Cellular Networks from 3D Micro-CT.
In: IEEE International Symposium on Biomedical Ima-
ging: From Nano to Macro
, 912 - 5.
Paragios N, Deriche R, 2002. Geodesic active regions: A
new framework to deal with frame partition problems
in computer vision.
Journal of Visual Communication
VISAPP 2012 - International Conference on Computer Vision Theory and Applications
296