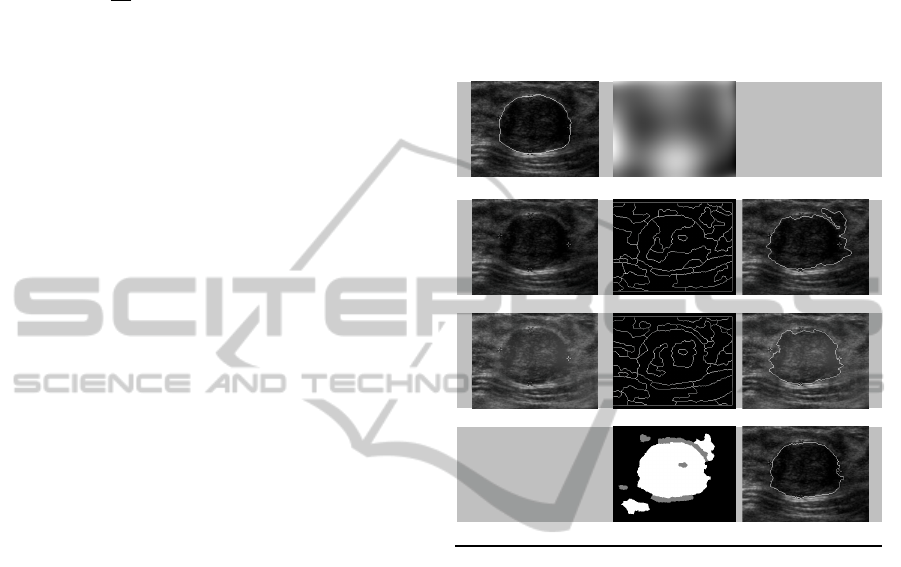
i
of pixel i, i , may be modeled as a polynomial
surface. To estimate the polynomial surface, a least
squared fitting is employed to minimize the cost
function:
i
ii
g
N
2
2
1
(11)
where
FR
if pixel i is in the foreground region
and
BR
if pixel i is in the background region.
Moreover,
),(
iii
yxf , where ),( yxf is a
polynomial function of order n. In this study, n is
set to 6.
3 RESULTS AND CONCLUSIONS
Forty-nine breast sonograms with intensity
inhomogeneity acquired from 1996 – 2004, each
from a different subject, were randomly selected
from the ultrasound image database in a teaching
hospital in Taiwan. The assessment is performed
based on the mean manually-delineated boundary of
each lesion in a breast sonogram. Each lesion was
demarcated by two graduate students and confirmed
by two experienced radiologists with 10- and 28-
year experience, respectively.
The mean distance between the lesion boundaries
attained by the proposed algorithm and the
corresponding manually delineated boundaries
defined by two radiologists is 1.571±0.513 pixels.
(Assessing Chan and Vese level set method for
intensity inhomogeneity-correction segmentation in
the same way, the mean distance error is
3.299±1.203 pixels, for the 49 images).
Figure 1 results of the proposed segmentation
approach and Chan and Vese level set method
applied on the intensity inhomogeneity image. Fig. 1
(a) shows the mean manually-delineated boundary
of the sonographic breast lesion given in Fig. 1 (c),
i.e., the original image.
Fig. 1(b) gives the intensity inhomogeneity field
and Fig. 1(f) shows the inhomogeneity-corrected
breast sonogram. The weak edge at the upper-right
portion of the lesion boundary becomes manifested
in the inhomogeneity-corrected breast sonogram.
The boundary derived by the Chan and Vese level
set method of the intensity inhomogeneity image and
intensity inhomogeneity-corrected image are shown
as Fig. 1 (e) and Fig. 1 (h), respectively. The Chan
and Vese level set method fails to capture the upper-
right portion of the lesion boundary which is a weak
edge caused by the intensity inhomogeneity. Fig. 1
(d) and Fig. 1 (g) show the cells (i.e., the prominent
components) derived by the FCC algorithm in the
intensity inhomogeneity image and intensity
inhomogeneity-corrected image, respectively. The
cell structures play the role of offering the feature
vectors to Gibbs-weighted K-means segmentation
algorithm, and the clustering result is shown as Fig.
1 (i). The boundary derived by Gibbs-weighted K-
means segmentation algorithm is shown as Fig. 1 (j).
(a) (b)
(c) (d) (e)
(f) (g) (h)
(i) (j)
Figure 1: The proposed algorithm segmentation results
compare with Chan and Vese level set method.
REFERENCES
Chan, T. F. and Vese, L. A., 2001. Active contours
without edges. IEEE Trans Image Processing 10(2).
Chen, C. M., Chou, Y. H. and Chen, C. S. K., 2005. Cell-
competition algorithm: a new segmentation algorithm
for multiple objects with irregular boundaries in
ultrasound images. Ultrasound in Medicine and
Biology 31(12).
Chen, D. R., Chang, R. F., Wu, W. J., Moon, M. K. and
Wu, W. L., 2003. 3-D breast ultrasound segmentation
using active contour model. Ultrasound in Medicine
and Biology 29(7).
Lee, C. Y., Chou, Y. H., Huang, C. S., Chang, Y. C., Tiu,
C. M., Chen, C. M., 2010. Intensity inhomogeneity
correction for the breast sonogram: Constrained fuzzy
cell-based bipartitioning and polynomial surface
modeling. Medical Physics 37(11).
Mohamed, R. M., El-Baz, A., and Farag A. A., 2004.
Image Modeling Using Gibbs-Markov Random Field
and Support Vector Machines Algorithm.
International Journal of Information Technology 1(14).
VISAPP 2012 - International Conference on Computer Vision Theory and Applications
384