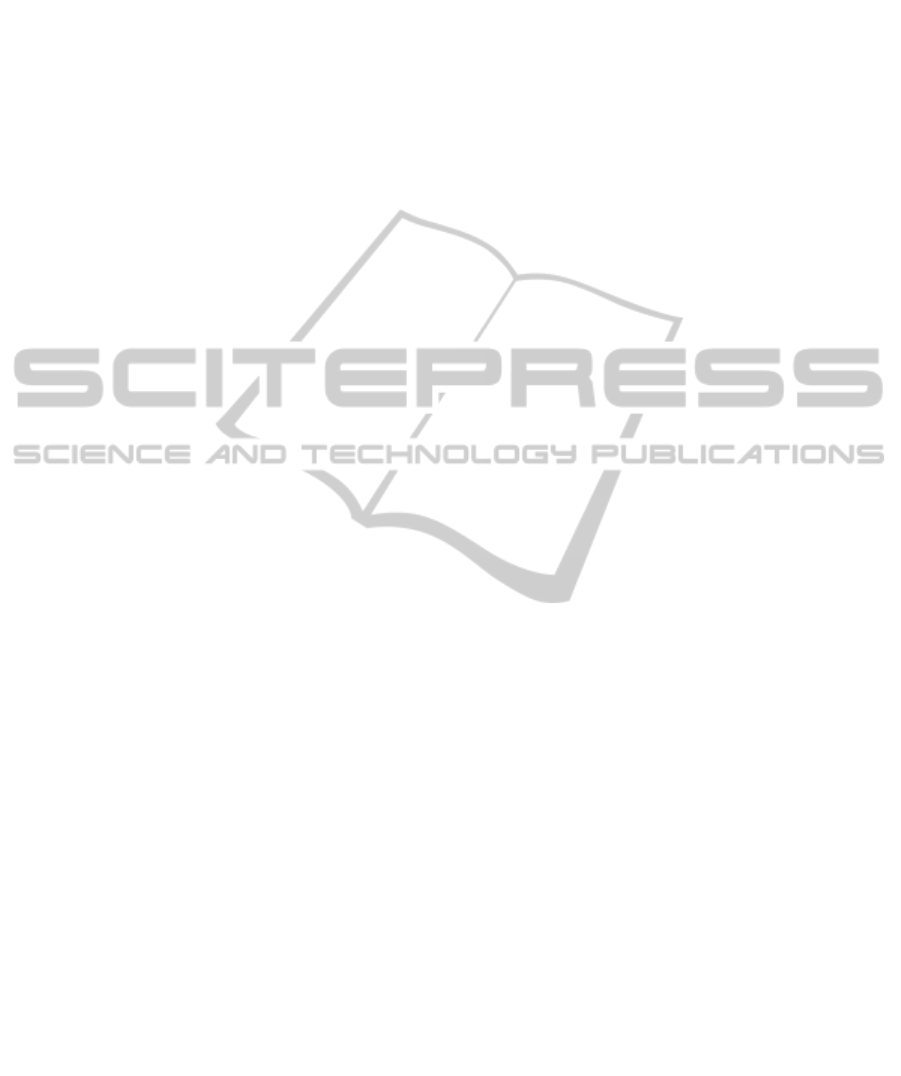
classification accuracy that is obtained when using
only reactive power as a feature. The results for just
the real power step change are better, with an overall
classification rate that is above 90%. And the
explanation for these results lie in the fact that using
just one metric does not work properly for
appliances with similar power consumption.
Nevertheless, the combination of real and reactive
power yields very good results, 100% for this set of
appliances, which is in accordance to results in
previous research.
As for the polynomial coefficients the
classification accuracy is very high using any of the
features, and the first thing that this shows is that
this solution can overcome the difficulty of
separating appliances with close consumption levels.
6 CONCLUSIONS
In this paper a low cost framework for non-intrusive
home energy monitoring and research was
presented. The final system is a very cost-effective,
(less than 300 Euros), energy monitor with some
load disaggregating capabilities and at the same
time, provides a very flexible research platform for
non-intrusive load monitoring.
Despite the promising results of the implemented
algorithms there is still a lot of room for
improvement and the flexibility of this framework
will allow the testing of different algorithms with
bigger sets of appliances, and, if possible, in
different houses.
NILM offers a big field of research, for example,
its concepts and techniques can be used in a lower
scale to create a smart power strip that would be able
to detect and turn-off appliances that are found to be
in stand-by mode. Also, it cannot be forgotten that
NILM can be easily exported to other domains,
opening the possibility of creating lower-cost sensor
networks. The ability to sense a whole house
together with the possibility of inferring human
activity will open various windows of research
opportunities. For example home automation
ambient intelligence and smart-grids are just three
fields that can greatly benefit from NILM.
Finally, it is also believed that there is still a lack
of services that use this technology making it
appealing not only for the consumers but also for the
electric companies and appliance manufacturers.
Which also opens a window of opportunity in the
area of service design, where researchers can aim at
creating innovative services on top of low-cost
technologies.
REFERENCES
Attari, S. Z. et al., 2010. Public perceptions of energy
consumption and savings. Proceedings of the National
Academy of Sciences of the United States of America,
107(37), p.16054-16059.
Bergés, M. et al., 2009. Learning Systems for Electric
Consumption of Buildings. Computing in Civil
Engineering, p.1-1.
Bergés, M., Soibelman, L. & Matthews, H. S., 2010.
Leveraging data from environmental sensors to
enhance electrical load disaggregation algorithms. In
proceedings of the International Conference
Computing Civil and Building Engineering.
Chisik, Y., 2011. An Image of Electricity : Towards an
Understanding of How People Perceive
Electricity. Ifip International Federation For
Information Processing, p.100-117.
Froehlich, J., Findlater, L. & Landay, J., 2010. The design
of eco-feedback technology. Proceedings of the 28th
international conference on Human factors in
computing systems CHI 10, 3, p.1999.
Gupta, S., Reynolds, M. S. & Patel, S. N., 2010.
ElectriSense: single-point sensing using EMI for
electrical event detection and classification in the
home. Computer Engineering, p.139-148.
Hart, G. W.,1992. Nonintrusive appliance load
monitoring. Proceedings of the IEEE, 80(12), 1870-
1891
Hohpe, G. and Woolf, B., 2003. Enterprise Integration
Patterns: Designing, Building and Deploying
Messaging Solutions. Addisson-Wesley Professional
Laughman, C. et al., 2003. Power signature analysis.
Computer, 1(2), p.56-63.
Luo, D., Norford, L. K. & Shaw, S. R., 2002. High
Performance Commercial Building Systems
Monitoring HVAC Equipment Electrical Loads from a
Centralized United Technologies Corporation.
ASHRAE Transactions, 108(1), p.841-857.
Parker, D. et al., 2006. How Much Energy Are We Using?
Potential of Residential Energy Demand Feedback
Devices. Solar Energy, p.1665-06.
Patel, S. N. et al., 2007. At the Flick of a Switch:
Detecting and Classifying Unique Electrical Events on
the Residential Power Line. In J. Krumm et al.,
eds.UbiComp 07 Proceedings of the 9th international
conference on Ubiquitous computing. Springer-
Verlag, pp. 271-288.
Peschiera, G., Taylor, J. E. & Siegel, J. A., 2010.
Response–relapse patterns of building occupant
electricity consumption following exposure to
personal, contextualized and occupant peer network
utilization data. Energy and Buildings, 42(8), p.1329-
1336.
SMARTGREENS2012-1stInternationalConferenceonSmartGridsandGreenITSystems
196