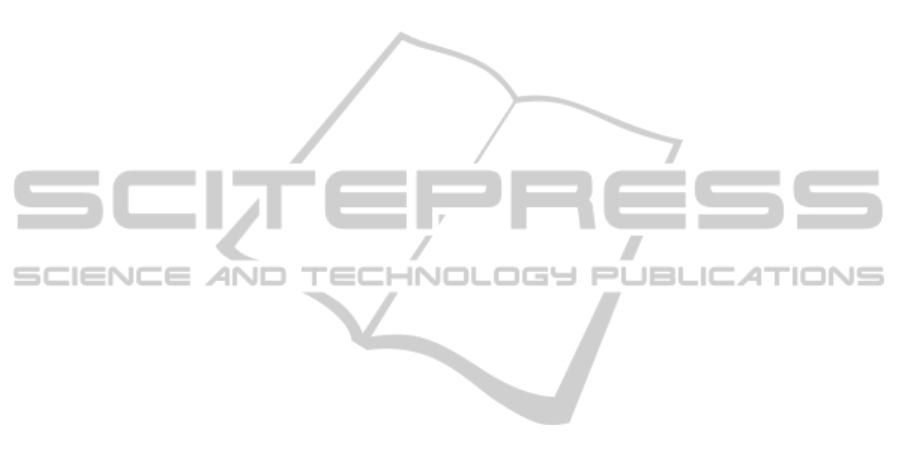
private property, direct interventions on equipments
may often be impossible.
In order to cope with the challenges future electricity
grids face, the smart grid vision proposes to couple
the traditional electricity network with an
infor-mation network allowing a “smart”
management of the electrical load (Schiller &
Fassmann, 2010).
In a micro smart grid, each home participates to the
network by selling its production or adapting its
consumption according to the load. The system that
manages the district grid gives live information on
the load that determines current purchase and sale
prices, according to customer contracts. Domestic
productions are connected to consumptions,
allowing a fine management of supply and demand,
practically use by use. Thus, grid management is not
only about limiting consumptions and maximising
productions, but also scheduling these streams
throughout the day, while taking usages into
consideration.
The vision of micro smart grids requires
sophisticated management capabilities, in particular
with respect to systems integration. Therefore this is
a promising use case for autonomic computing to
illustrate its capabilities while addressing concrete
issues of the domain. The rest of this paper presents
scenarios that detail how autonomic approaches
could be a solution to micro smart grid management,
with analysis of the technical requirements possible
autonomic solutions should fulfil.
3 “HEATING SYSTEM”
SCENARIO
Taking advantage of renovation work, Mark has
invested in an autonomic underfloor heating. Thanks
to a set of sensors and actuators, the system can
monitor temperature in the room and receive
instructions. A hot water tank allows accumulating
and returning heat; this is done according to
electricity rates, to Mark's orders, to the weather, to
the actual temperatures in the rooms and to whether
or not someone is present in the apartment. Mark
has also activated a geo-location functionality:
aware of Mark's position, the system can reduce
temperature while he is out and ensures everything
returns to normal when he comes back.
Mark may monitor his system live state: electrical
consumption, desired temperatures compared to
actual temperatures, money saved thanks to the
water tank stock. The initial investment was
substantial but Mark sees a difference on his bill and
can be confident he will get return on investment. In
addition to ecological considerations on energy
consumption reduction, the regulator adapts to the
occupants' desires via simple instructions. Reading
his system history, Mark has realised that some
occasional adjustments he did by hand – for
instance, in his bathroom – have been learnt by the
autonomic system that anticipates his morning
shower since then.
Mark's inquiring mind has led him to play with his
regulator's most advanced functionalities. He has
been offered a choice of management profiles,
favouring comfort, energy savings or bill reduction.
After some experimentations, Mark eventually
decided to follow an highly economical policy that
reduced again his electricity bill. And when guests
are expected, the system can automatically restore
more suitable comfort conditions.
Analysis: This scenario raises the following issues:
conflicts between objectives and an
unpredictable context;
necessity to anticipate the context, in
particular, to develop learning features.
The equipment this scenario describes follows two
contradictory objectives: “maintain temperature”
versus “limit electrical consumption”, in a dynamic,
unpredictable context. This context must be
monitored by the system via temperature sensors,
measurements of the electricity consumption, grid
load sensors, hot water stock probes, possibly
presence sensors and geo-location system. Since
measurements are imprecise by nature, the system
must take possible errors (unidentified moves in a
room, GPS failure, etc.) into account.
In addition to adapting to the context (temperature,
domestic load) the autonomic system must be able to
forecast it in order to achieve efficient management
of its stock. In the scenario, the system must
anticipate Mark's return home after work; it reloads
its stock during the day, according to a consumption
forecast for the night. This forecast may imply mid
to long-term learning capabilities, in order to
evaluate a standard night profile, possible variations
of Mark's consumption, etc.
Unexpected events (unforeseen load peak, unusual
order from the user, heat wave, cold wave, etc.) can
challenge previous forecasts and threaten the
system's functioning. However, it must be able to
anticipate such variations and allow room for
manoeuvre in order to face the unexpected with
appropriate reactions. Sophisticated objectives can
help the system solve the “margin of error versus
optimisation” dilemma.
SMARTGREENS2012-1stInternationalConferenceonSmartGridsandGreenITSystems
138