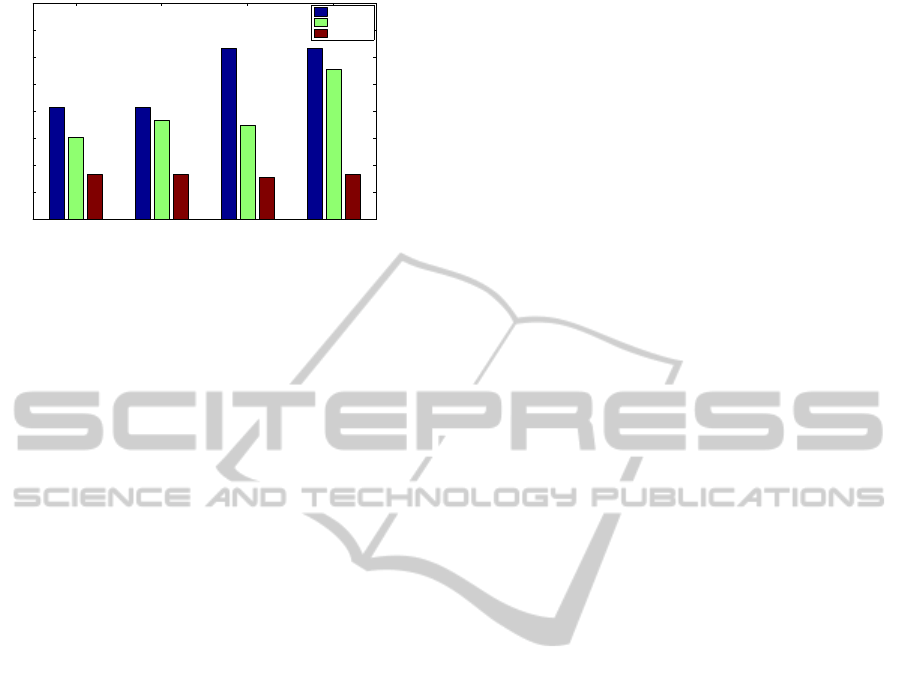
slow, with V2G slow, w/o V2G fast, with V2G fast, w/o V2G
0
20
40
60
80
100
120
140
160
community peak demand (kW)
dumb
distributed
centralized
26.6
59.7
11.8
59.7
44.9
75.1
12
74.2
Figure 6: Comparison of the charging strategies based on
the peak demand of the community (target battery level is
90% of the battery capacity).
strategy yields a reduction in the peak load by around
14% in all the four scenarios, which averageto 14.4%.
On the other hand, the centralized charging strategy
reduces the peak by as large as 65%, with average im-
provement of 38.1%.
Figure 6 depicts the relative performance of the
charging strategies when the target battery level at the
start of each commute is 90% of the battery capac-
ity. In this scenario, the distributed charging strategy
achieves an average peak reduction of 23.9%, where
its largest reduction is as high as 44.9%. Whereas
the centralized charging strategy obtains 67.2%, with
75.1% largest improvement.
All the simulation results reveal that the dumb
charging strategy results in large peak demands which
arise from the simultaneous charging of EVs right af-
ter they arrive at home. In the contrary, the central-
ized charging strategy has effectively revealed the po-
tential of the synergy between the EVs and the lo-
cal production to reduce the peak load by exploiting
the flexibility of the EVs. However, the centralized
charging strategy represents a situation where all the
households are cooperative to reduce the peak load
and all the EVs are managed by a centralized unit,
which might contradict the tendency of the house-
holds to autonomously manage their own EVs. On
the other hand, the distributed charging strategy de-
livers 11% to 44.9% reduction in peak demand over
the dumb charging strategy while respecting the au-
tonomy of the households to manage their own EVs.
The strength of the distributed strategy lies in the dy-
namic pricing model that is used as incentive to co-
ordinate the autonomous households that tend to self-
ishly minimize their cost. These improvements are
vital because they reduce the load on the transformer
connecting the energy community with the remain-
ing power grid. The reduction in the peak values has
the advantage of increasing the lifetime of the trans-
former as well as minimizing the need for installing
large capacity transformer.
6 CONCLUSIONS
In an energy community composed of prosumer
households that own EV, the aggregate load profile
might become highly volatile due to the intermittence
of the distributed generations as well the load of the
EVs, which could lead to a large peak load that might
exceed the capacity of the transformer. We have pro-
posed a centralized and a distributed EV charging
strategies that try to minimize the peak load in such
scenario by exploiting the synergy between the lo-
cal distributed generations and the flexibility of the
EVs. Our centralized charging strategy schedules the
(dis)charging of the EVs at a centralized unit assum-
ing that all the prosumers in the energy community
cooperate with each other to minimize the peak de-
mand. Our distributed charging strategy, however,
assumes that the prosumer households autonomously
manage their EVs and tend to selfishly minimize their
cost. Our distributed strategy coordinates the house-
holds to minimize the peak demand by providing in-
centives based on our novel dynamic pricing model.
In addition to presenting a detailed model of the sys-
tem taking into consideration the local constraints, we
have derived the driving behaviors of the EV users
from a realistic mobility data. Based on our simula-
tion results, we have shown that our centralized and
distributed charging strategies can reduce the peak by
as large as 75.1% and 44.9%, respectively, compared
to a reference dumb charging strategy. Therefore, our
proposed strategies can help to reduce the load on the
transformer connecting the energy community to the
rest of the grid, and also minimizes the need for up-
grading the capacity of the transformer.
REFERENCES
Fluhr, J., Ahlert, K., and Weinhardt, C. (2010). A stochastic
model for simulating the availability of electric vehi-
cles for services to the power grid. In 43rd HICSS,
2010, pages 1–10. IEEE.
Houwing, M., Negenborn, R., and De Schutter, B. (2011).
Demand response with micro-chp systems. Proceed-
ings of the IEEE, 99(1):200–213.
Ipakchi, A. and Albuyeh, F. (2009). Grid of the future.
Power and Energy Magazine, IEEE, 7(2):52–62.
Knapen, L., Kochan, B., Bellemans, T., Janssens, D., and
Wets, G. (2011). Activity based models for coun-
trywide electric vehicle power demand calculation.
SGMS.
Kok, J., Warmer, C., and Kamphuis, I. (2005). Power-
matcher: multiagent control in the electricity infras-
tructure. In Proceedings of the fourth international
joint conference on Autonomous agents and multia-
gent systems, pages 75–82. ACM.
SMARTINTEGRATIONOFELECTRICVEHICLESINANENERGYCOMMUNITY
31