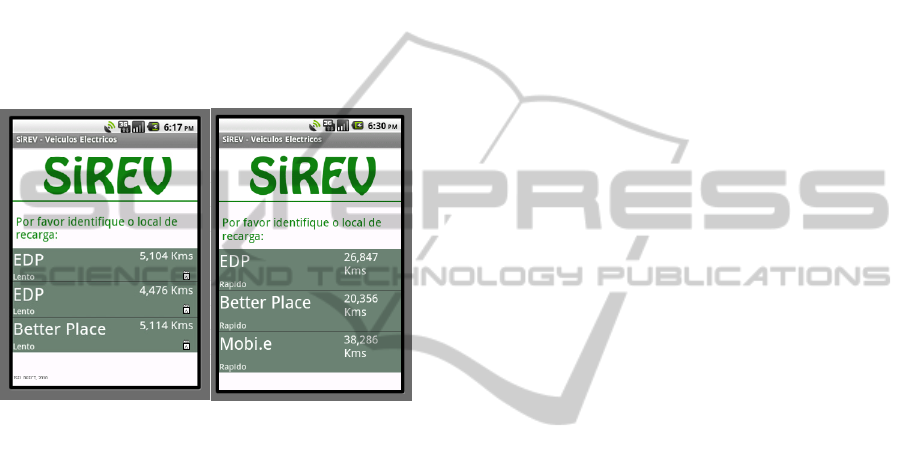
after the driver decides to return home. The vehicle's
range is 70%, and will not reach the destination.
After walking 50 km of new drivers are notified
that you have to carry the load of the vehicle. In this
situation the recommendation system will consider
the following context: (1) Mode of Operation:
leisure; (2) Time of day: Afternoon; and (3)
Destination: House.
By stating that the destination is home, the
recommendation system provides fast charging
points along the route (Figure 13-right), in this
context because the driver always chooses this type
of sites because they want to get home as soon as
possible.
Figure 13: Recommendations given by the Recomender
System, because SiREV detected that range is below the
configured limit of 30 km: On left - recommendation for
slow charging points; On right - recommendation for fast
charging points (in Portuguese language).
7 CONCLUSIONS
The main objective of this work is to help drivers in
the daily use of Electric Vehicles (EV), acting
proactively and increasing the attractiveness of EV.
However, EV technology is still under development,
and so, all available information systems are
necessary for a successful full integration of the
Electric Vehicles in the transportation system. In this
work it is proposed the integration of information
from various areas that can be important in the
successful implementation of a mobility model for
drivers of Electric Vehicles. It is also necessary to
ensure that the integration model is sufficiently
generic and flexible to be adapted to different
systems, having a low impact on the implementation
of a Recommender System. On the other hand, it is
easy to see that the technologies needed to develop a
mobile system, capable of receiving position and
geographic information, and able to establish
connection to external devices or to the Internet,
have a very advanced level of development. The
Android system allows a very simple and efficient
development of applications, with the advantage of
being totally free of cost, both with regard to the
licensing of their own API and developer tools,
which are all based on free software. The ease of
development, the integration with GPS, USB,
Bluetooth, SMS, and the test execution, makes the
implementation work very efficient.
The usage of the developed Recommender
System showed only part of all its potential for
application in the Electric Mobility context, It will
be part of future work to deepen the study of a more
complex model for the Recommender System, with
the optimization of the scenario in question,
demonstrating the validity and enforceability of this
project in a real use, with its added value, in an
attempt to identify functional requirements and
possible ways for their implementation.
ACKNOWLEDGEMENTS
This work is financed by FEDER Funds, through the
Operational Programme for Competitiveness Factors
– COMPETE, and by National Funds through FCT –
Foundation for Science and Technology of Portugal,
under the project PTDC/EEA-EEL/104569/2008 and
the project MIT-PT/EDAM-SMS/0030/2008.
REFERENCES
Ferreira, J. C., Monteiro V. and Afonso J., 2011. Mobile
Geographic Range Prediction for Electric Vehicles.
Proceedings of the 1st Conference in Electronics,
Telecommunications, and Computer Engineering, from
24th to the 25th November, 2011, at Instituto Superior
de Engenharia de Lisboa – ISEL, Lisbon- Portugal.
Gediminas A., (2005). Toward the Next Generation of
Recommender Systems: A Survey of the State-of-the-Art
and Possible Extensions, IEEE Transactions on
Knowledge and Data Engineering, Vol. 17, Nº. 6, June
2005
Tuzhilin A., Gediminas A., 2001. Multidimensional
Recommender Systems: A Data Warehousing
Approach, Springer Berlin/Heidelberg, 2001.
Pereira P., (2010). Sistema de Recomendação para
Condutores de Veículos Eléctricos. MSc dissertation -
ADEETC- ISEL, Lisbon.
Fontes T. F., (2008). Determinação do melhor caminho
em sistemas de transporte terrestre (BPath – Best
Path). Final Year Project at ADEETC – ISEL, Lisbon.
SMARTGREENS2012-1stInternationalConferenceonSmartGridsandGreenITSystems
58