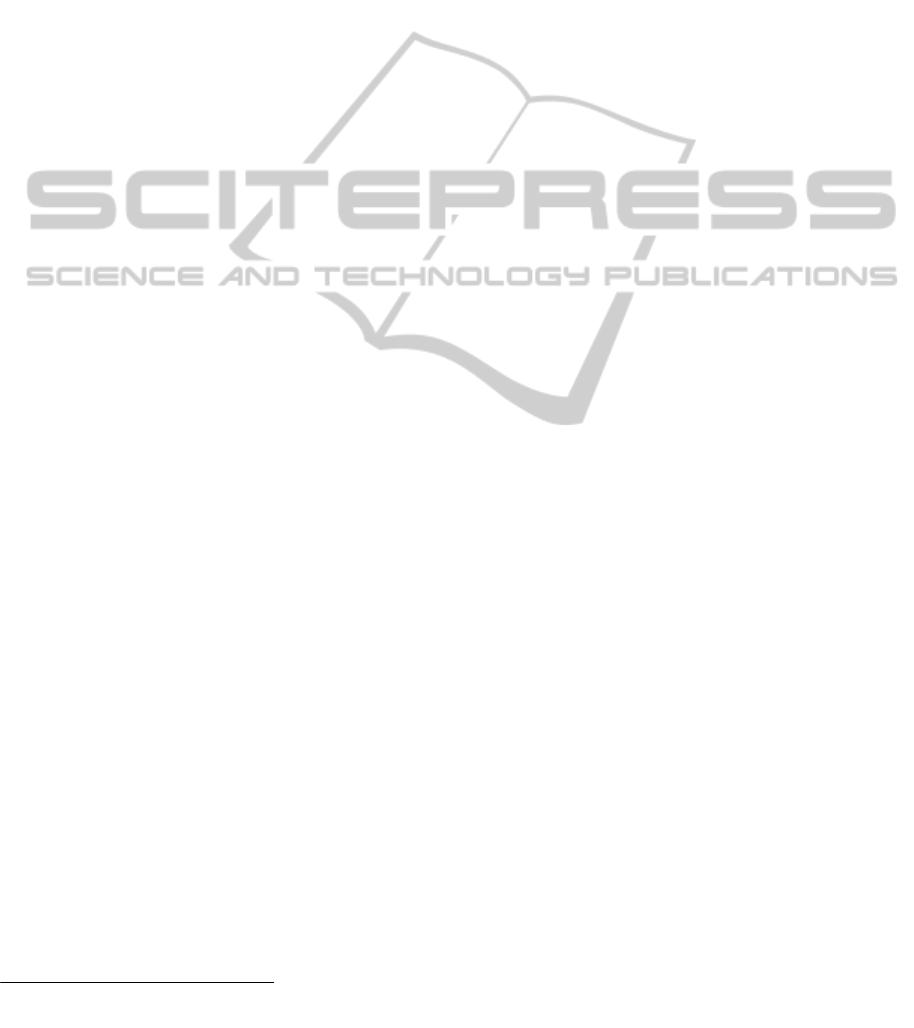
of hybridism, individualization and evolution. This
theoretical characterization constitutes the base for
an inferential model that automatically identifies
genres within web pages. Later, Santini (2007) pre-
sented a model based on a scheme performing zero-
to-multi genre assignments. Sharoff (2007) per-
formed experiments to find the best set of features
that delimit a typology useful for classifying web
pages according to their domain and genre. With
respect to Greek, Stamatatos et al. (2000) described
an approach that relies on classification schemes and
natural language processing modules for categoriz-
ing Greek texts according to their genre and author.
The application of their technique to various Greek
corpora proved the feasibility of their proposed
method.
3 METHODOLOGY
Our text genre detection approach operates upon
pre-determined classes of text types in which it
looks for features that signify the genre of the re-
spective texts. Before delving into the details of our
method, we should point out that we distinguish text
genre from text type in that the former represents the
way in which information is communicated via
texts, whereas the latter represents the type of infor-
mation texts convey. As an example consider the
current paper, whose style might be characterized as
technical or formal and whose type might be charac-
terized as scientific publication or research paper.
Based on the discrimination between style and
type, we propose a method that tries to identify the
genre (i.e. style) of texts that belong to particular
types. To obviate the need for pre-classifying texts
according to their type, we rely on an existing tex-
tual type classification scheme
1
that defines the fol-
lowing categories of text types: (i) narrative: an
account of events, (ii) expository: text that explains
something, (iii) procedural: text that gives instruc-
tions on how to do something and (iv) descriptive:
text that lists the characteristics of something. Each
of the above category types contains sub-types,
which share common elements in structure and con-
tent with their parental type and with each other, and
at the same time they deliver clues that are unique
and representative of their respective sub-type cate-
gory. As sub-type example categories consider the
following: (i) narrative texts may be sub-categorized
into novels, poetry or short stories, (ii) expository
texts may be sub-categorized into news articles,
travel books and periodicals, (iii) procedural texts
may be sub-categorized into various kinds of guide-
books, e.g. cooking books, manuals, installation
guides and (iv) descriptive texts may be sub-
categorized into encyclopedias, grammars and dic-
tionaries. Given the type and/or sub-type of a text,
our method tries to identify structural and contextual
elements that signify the text's style or else genre. To
ensure the accurate identification of genre, we relied
on a dataset of texts already classified into one of the
above types and after manually inspecting them we
extracted their genre-indicative elements. Manual
inspection of texts was performed by human expert
annotators, i.e. linguistics, to whom we distributed a
number of texts along with their respective type
categories and we asked them to indicate for every
text type the features (both contextual and structural
if feasible) that yield its style. Note that we did not
ask our study participants to directly indicate the
genre of every examined text type, but rather to in-
dicate the elements that shape the stylistic identity of
every examined text. This is because the quest in our
study is to determine the characteristics that distin-
guish the genre of text types from one another rather
than annotate texts with stylistic information. Based
on the above determination, one can employ super-
vised clustering methods for automatically grouping
texts in terms of their common underlying stylistic
elements. Turning back to the description of our
method, we should point out that due to space and
time constraints, we did not examine all the sub-
categories of every text type but rather we relied on
texts categorized under a specific (randomly se-
lected) type sub-category. Thus, for the narrative
category, we relied on texts belonging to the short
stories sub-category, for the expository category we
relied on texts belonging to the news articles sub-
category, for the procedural category we relied on
texts belonging to the cookbooks sub-category and
for the descriptive category we relied on texts be-
longing to the dictionaries sub-category.
The dataset used for each of the above catego-
ries, concerned online texts that we harvested from
suitable websites, i.e. sites containing (i) short sto-
ries, (ii) online newspapers, (iii) sites about recipes
and cooking and (iv) lexicographic sites. In the next
section, we report on the statistics of our collected
dataset. Now, we present the way in which human
annotators assessed our collected data sources in
order to identify their structural and contextual ele-
ments that signify their genre. To carry out our
study, we recruited 5 experienced linguists (3 fe-
male, 2 male), who volunteered to read the texts
collected for every type category and indicate the
1
http://www.sil.org/linguistics/glossaryoflinguisticterms/WhatIsATex
t.htm
WEBIST2012-8thInternationalConferenceonWebInformationSystemsandTechnologies
734