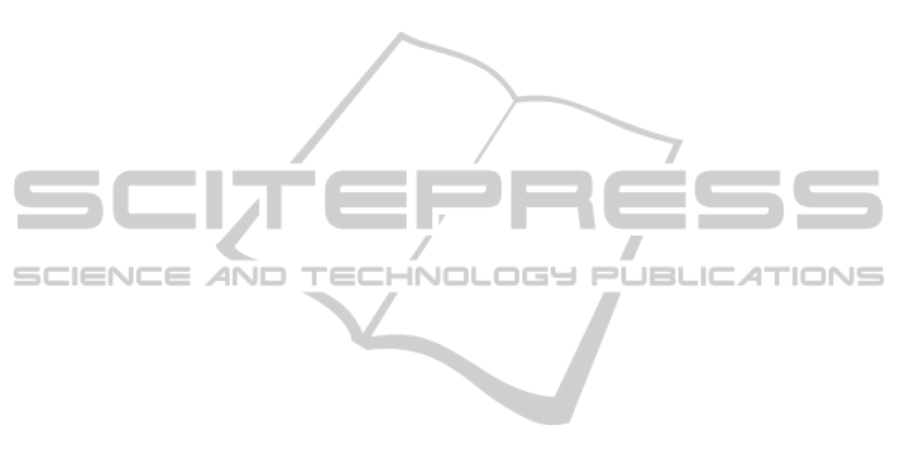
role in establishing effective cooperation among dis-
tributed Internet application participants.
In this paper we presented a game theoretic trust-
based recommendation system. Our approach is
based on the adoption of trust information in recom-
mendation system in order to improve the quality of
suggestions, thus identifying the best strategy for the
game model proposed. To sum up, the main con-
tributions of this paper are: (i) the representation of
e-Commerce interactions by means of a game the-
ory model, (ii) the proposal of the best strategy by
means of the adoption of trust information, and (iii)
the modeling of a trust-aware recommendation sys-
tem by means of an integration between similarity,
which is evaluated according the customer profile of
interest, and the proposed trust metrics.
In future, we aim at investigating two main direc-
tions. The first is to extend the approach presented
here in order to find the experimentalevidencethat the
proposed model can provide benefits (e.g., increased
profits for merchants or increase customer surplus) in
a large real dataset of Poste Italiane. Furthermore,
user information regarding age, sex, occupation, and
geographical information with the provided feedback
related to some products are available so that exper-
imenting deeper the different trust model could con-
firm the benefits of the proposed approach.
The second direction will examine how to find
out multi-player scenario, indeed in e-Commerce do-
main online shopping is becoming more and more
widespread and represents an everyday activity for
many. In this actual scenario, we will analyze the pro-
posed game model when it is executed with different
players, several customers and several merchants at
the same time. Moreover, we will highlight new aris-
ing issues and investigate the best strategy.
REFERENCES
Adomavicius, G. and Tuzhilin, A. (2005). Toward the
next generation of recommender systems: a survey of
the state-of-the-art and possible extensions. Knowl-
edge and Data Engineering, IEEE Transactions on,
17(6):734–749.
Birtolo, C., Ronca, D., Armenise, R., and Ascione, M.
(2011). Personalized suggestions by means of col-
laborative filtering: A comparison of two different
model-based techniques. In NaBIC, pages 444–450.
IEEE.
Goldberg, D., Nichols, D., Oki, B. M., and Terry, D. (1992).
Using collaborative filtering to weave an information
tapestry. Commun. ACM, 35:61–70.
Herlocker, J. L., Konstan, J. A., Terveen, L. G., and Riedl,
J. T. (2004). Evaluating collaborative filtering recom-
mender systems. ACM Trans. Inf. Syst., 22:5–53.
Huang, C. and Yin, J. (2010). Effective association clus-
ters filtering to cold-start recommendations. In Fuzzy
Systems and Knowledge Discovery (FSKD), 2010 Sev-
enth Int. Conf. on, volume 5, pages 2461–2464.
Huberman, B. A., Adar, E., and Fine, L. R. (2005). Valuat-
ing privacy. IEEE Security and Privacy, 3:22–25.
Jeong, B., Lee, J., and Cho, H. (2010). Improving memory-
based collaborative filtering via similarity updating
and prediction modulation. Information Sciences,
180(5):602 – 612.
Lim, H. C., Stocker, R., and Larkin, H. (2008). Ethical trust
and social moral norms simulation: A bio-inspired
agent-based modelling approach. Web Intelligence
and Intelligent Agent Technology, IEEE/WIC/ACM In-
ternational Conference on, 2:245–251.
Liu, B. and Yuan, Z. (2010). Incorporating social networks
and user opinions for collaborative recommendation:
local trust network based method. In Proc. of the
Workshop on Context-Aware Movie Recommendation,
CAMRa10, pages 53–56, New York, NY, USA. ACM.
Liu, L. and Shi, W. (2010). Trust and reputation manage-
ment. Internet Computing, IEEE, 14(5):10 –13.
Massa, P. and Avesani, P. (2007). Trust-aware recommender
systems. In Proceedings of the 2007 ACM conference
on Recommender systems, RecSys ’07, pages 17–24,
New York, NY, USA. ACM.
Mui, L., Mohtashemi, M., and Halberstadt, A. (2002). A
computational model of trust and reputation. In Sys-
tem Sciences, 2002. HICSS. Proceedings of the 35th
Annual Hawaii International Conference on, pages
2431 – 2439.
Oza, N. V. (2006). Game theory perspectives on client:
vendor relationships in offshore software outsourc-
ing. In Proceedings of the 2006 international work-
shop on Economics driven software engineering re-
search, EDSER ’06, pages 49–54, New York, NY,
USA. ACM.
Resnick, P. and Zeckhauser, R. (2002). Trust among
strangers in Internet transactions: Empirical analysis
of eBay’s reputation system. In Baye, M. R., edi-
tor, The Economics of the Internet and E-Commerce,
volume 11 of Advances in Applied Microeconomics,
pages 127–157. Elsevier Science.
Shiva, S., Roy, S., and Dasgupta, D. (2010). Game theory
for cyber security. In Proceedings of the Sixth Annual
Workshop on Cyber Security and Information Intelli-
gence Research, CSIIRW ’10, pages 34:1–34:4, New
York, NY, USA. ACM.
Zarghami, A., Fazeli, S., Dokoohaki, N., and Matskin,
M. (2009). Social trust-aware recommendation sys-
tem: A t-index approach. In Web Intelligence and
Intelligent Agent Technologies, 2009. WI-IAT ’09.
IEEE/WIC/ACM International Joint Conferences on,
volume 3, pages 85 –90.
WEBIST2012-8thInternationalConferenceonWebInformationSystemsandTechnologies
582