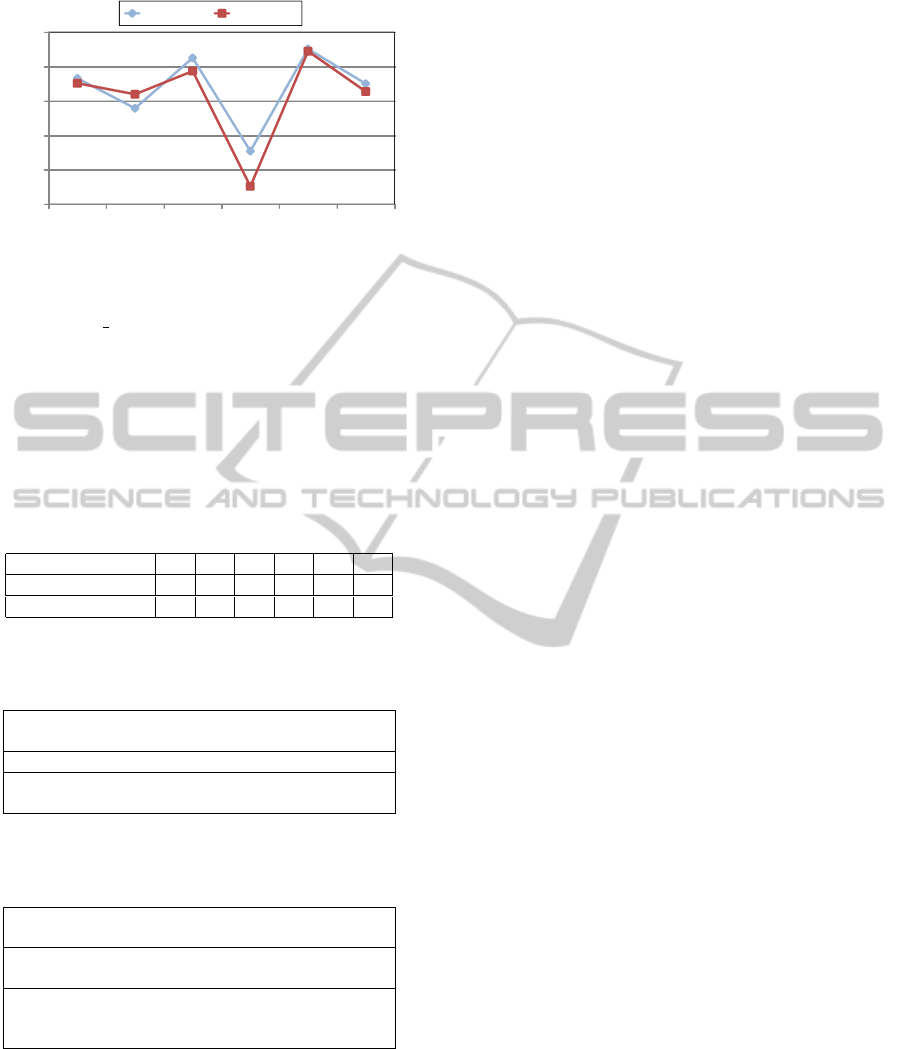
40
50
60
70
80
90
1 2 3 4 5 6
F measure(%)
Data set ``A'' Data set ``B''
Figure 7: Result of classification experiment for 2 category
classification sample patterns.
t f -id f and co t f-id f. So, the sentences with clear
contents can be classified accurately. On the other
hand, in categories which is confused contents such
as Category 4, the system can not identify words that
characterize the category, and the words appear in
other categories. Thus it is difficult to classify in these
categories.
Table 7: The number of correctly categorized opinions for
each category.
Category Number 1 2 3 4 5 6
Data set “A” 89 53 46 39 25 22
Data set “B” 89 60 36 43 21 30
Table 8: Examples of category 4 “Dangerousness and how
to deal with information society”.
I would like to learn how to deal with overflooding
information.
But such information is not always right.
But I do not know whether it is good to depend on
information in the web.
Table 9: Example of category 5 “Electronic tag technol-
ogy”.
And it is nice to know electronic tag is used in book
stores’ security system.
I understood that electronic tags are used every-
where.
I think there will be no more cash registers in the
future because electronic tags are used for in all
goods.
5 CONCLUSIONS
This paper addressed the classification method of
open-ended questionnaire using category-based dic-
tionary from category classification samples. Our
proposed method uses typical words involvement de-
gree which is an index that measures the number of
typical words and co-occurrence patterns that charac-
terize a category. By applying our proposed method
to questionnaires about a university lecture, 71% of
these questionnaires are classified accurately. As a
result of experiments, the clearer the contents are, the
more accurate the proposed method can classify the
questionnaires.
REFERENCES
Atkinson, M. and Van der Goot, E. (2009). Near real time
information mining in multilingual news. In Proceed-
ings of the 18th international conference on World
wide web, WWW ’09, pages 1153–1154, New York,
NY, USA. ACM.
Berry, M. (2003). Survey of Text Mining : Clustering, Clas-
sification, and Retrieval. Springer.
Chim, H. and Deng, X. (2008). Efficient phrase-based doc-
ument similarity for clustering. IEEE Transactions on
Knowledge and Data Engineering, 20:1217–1229.
Matsuo, Y. and Ishizuka, M. (2004). Keyword extraction
from a single document using word co-occurrence sta-
tistical information. International Journal on Artifi-
cial Intelligence Tools, 13(1):157–169.
Ramos, J. (2002). Using TF-IDF to Determine Word Rele-
vance in Document Queries. Technical report, Depart-
ment of Computer Science, Rutgers University, 23515
BPO Way, Piscataway, NJ, 08855e.
Salton, G. and Buckley, C. (1988). Term-weighting ap-
proaches in automatic text retrieval. Information pro-
cessing and management, 24(5):513–523.
Trieschnigg, D., Pezik, P., Lee, V., de Jong, F., Kraaij,
W., and Rebholz-Schuhmann, D. (2009). MeSH Up:
effective MeSH text classification for improved doc-
ument retrieval. Bioinformatics (Oxford, England),
25(11):1412–1418.
Tseng, Y.-H., Lin, C.-J., and Lin, Y.-I. (2007). Text mining
techniques for patent analysis. Inf. Process. Manage.,
43:1216–1247.
Zhang, D. and Lee, W. S. (2003). Question classification
using support vector machines. In Proceedings of the
26th annual international ACM SIGIR conference on
Research and development in informaion retrieval, SI-
GIR ’03, pages 26–32, New York, NY, USA. ACM.
ICEIS2012-14thInternationalConferenceonEnterpriseInformationSystems
198