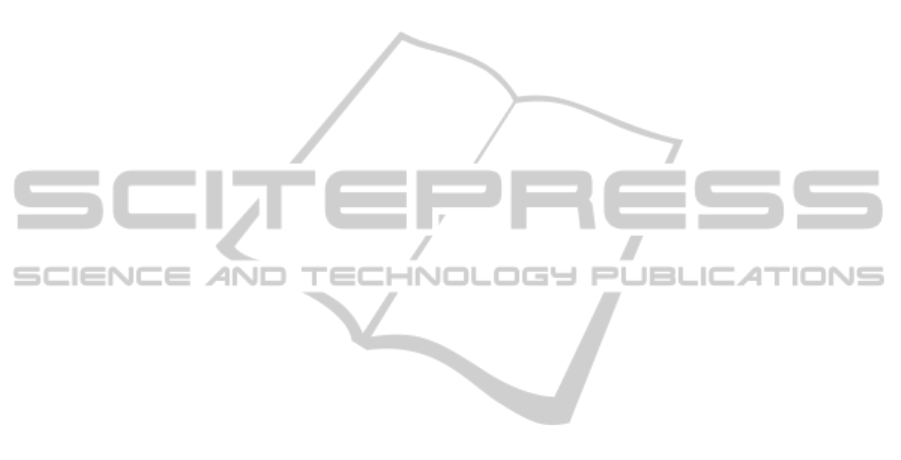
the system parametrization. The robustness of the
generative model to initialization, and its ability to
learn the model parameters for textured/untextured,
indoor/outdoor environments have been demonstrated
through experimental analysis and from the many
hours VISAR01 has been allowed to roam out into
the faculty corridors, avoiding both static and dy-
namic objects.
Future work will see the inclusion of structure-
from-motion depth estimation to allow the robot to
transition from one type of surface to another auto-
matically, and new exploration behaviours based on
the probability of traversability, rather than simple bi-
nary classification. This means that instead of merely
moving towards an obstacle-free path determined by
a hard decision (Santosh et al., 2008), the robot may
decide to take the path that has the highest probability
of being traversable.
ACKNOWLEDGEMENTS
The research work disclosed in this publication is par-
tially funded by the Strategic Educational Pathways
Scholarship (Malta). The scholarship is part-financed
by the European Union - European Social Fund (ESF)
under the Operational Programme II - Cohesion Pol-
icy 2007-2013, Empowering People for More Jobs
and a Better Quality of Life.
REFERENCES
Al-Athari, F. (2008). Estimation of the mean of truncated
exponential distribution. Journal of Mathematics and
Statistics, 4(4):284–288.
Dalal, N. and Triggs, B. (2005). Histograms of oriented
gradients for human detection. In IEEE Conf. on Com-
puter Vision and Pattern Recognition, pages 886–893.
Davidson, J. and Hutchinson, S. (2003). Recognition of
traversable areas for mobile robotic navigation in out-
door environments. In IEEE/RSJ Int. Conf. on Intelli-
gent Robots and Systems, pages 297–304.
DeSouza, G. and Kak, A. (2002). Vision for mobile robot
navigation: A survey. IEEE Trans. Pattern Analysis
and Machine Intelligence, 24(2):237–267.
Felzenszwalb, P. and Huttenlocher, D. (2004). Efficient
graph-based image segmentation. Int. Journal of
Computer Vision, 59(2):167–181.
Garthwaite, P., Jolliffe, I., and Jones, B. (2002). Statisti-
cal Inference. Oxford University Press, Inc., second
edition.
Hadsell, R., Sermanet, P., Ben, J., Erkan, A., Scoffier, M.,
Kavukcuoglu, K., Muller, U., and LeCun, Y. (2009).
Learning long-range vision for autonomous off-road
driving. Journal of Field Robotics, 26(2):120–144.
Hoiem, D., Efros, A., and Hebert, M. (2007). Recovering
surface layout from an image. Int. Journal of Com-
puter Vision, 75(1):151–172.
Katramados, I., Crumpler, S., and Breckon, T. (2009). Real-
time traversable surface detection by colour space fu-
sion and temporal analysis. In Int. Conf. Computer
Vision Systems, volume 5815, pages 265–274.
Kim, D., Oh, S., and Rehg, J. (2007). Traversability clas-
sification for UGV navigation: a comparison of patch
and superpixel representations. In IEEE/RSJ Int. Conf.
on Intelligent Robots and Systems, pages 3166–3173.
Kosaka, A. and Kak, A. (1992). Fast vision-guided mo-
bile robot navigation using model-based reasoning
and prediction of uncertainties. In IEEE/RSJ Int. Conf.
on Intelligent Robots and Systems, pages 2177–2186.
Lorigo, L., Brooks, R., and Grimson, W. (1997). Visually-
guided obstacle avoidance in unstructured environ-
ments. In IEEE/RSJ Int. Conf. on Intelligent Robots
and Systems, pages 373–379.
M
¨
aenp
¨
a
¨
a, T., Turtinen, M., and Pietik
¨
ainen, M. (2003).
Real-time surface inspection by texture. Real-Time
Imaging, 9(5):289–296.
Meng, M. and Kak, A. (1993). NEURO–NAV: A neural
network based architecture for vision-guided mobile
robot navigation. In IEEE Int. Conf. on Robotics and
Automation, pages 750–757.
Michels, J., Saxena, A., and Ng, A. (2005). High speed
obstacle avoidance using monocular vision and rein-
forcement learning. In Proceedings 22nd Int. Conf.
on Machine Learning, pages 593–600.
Mitchell, T. (1997). Machine Learning. The McGraw-Hill
Companies, Inc., first edition.
Murali, V. and Birchfield, S. (2008). Autonomous navi-
gation and mapping using monocular low-resolution
grayscale vision. In IEEE Workshop on Computer Vi-
sion and Pattern Recognition, pages 1–8.
Ohno, T., Ohya, A., and Yuta, S. (1996). Autonomous nav-
igation for mobile robots referring pre-recorded im-
age sequence. In IEEE/RSJ Int. Conf. on Intelligent
Robots and Systems, pages 672–679.
Prince, S. (2011). Computer vision models.
Roning, J., Taipale, T., and Pietikainen, M. (1990). A 3-d
scene interpreter for indoor navigation. In IEEE Int.
Workshop on Intelligent Robots and Systems, pages
695–701.
Santosh, D., Achar, S., and Jawahar, C. (2008). Au-
tonomous image-based exploration for mobile robot
navigation. In IEEE Int. Conf. on Robotics and Au-
tomation, pages 2717–2722.
Sofman, B., Lin, E., Bagnell, J., Cole, J., Vandapel, N.,
and Stentz, A. (2006). Improving robot navigation
through self-supervised online learning. Journal of
Field Robotics, 23(11-12):1059–1075.
Ulrich, I. and Nourbakhsh, I. (2000). Appearance-based ob-
stacle detection with monocular color vision. In AIII
Conf. on Artificial Intelligence, pages 866–871.
ICINCO2012-9thInternationalConferenceonInformaticsinControl,AutomationandRobotics
184