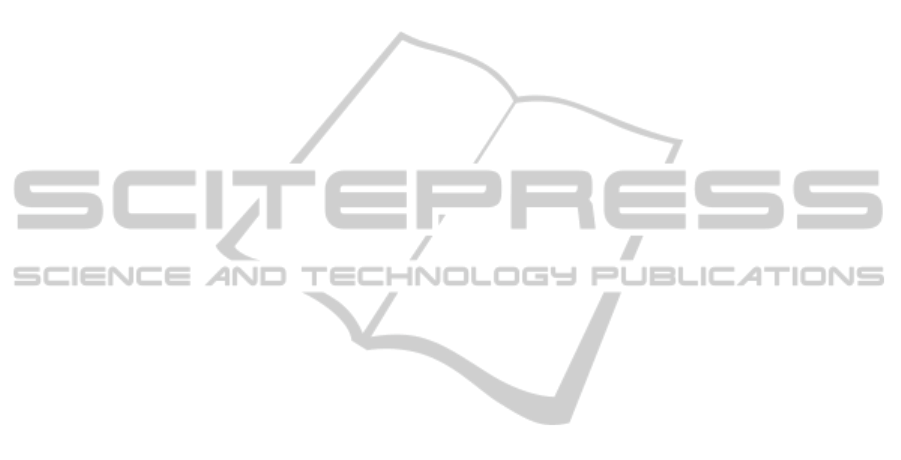
to be good tool, which allows for combining selected
methods (see e.g. (Mzyk, 2010b)), depending on
specificity of the particular task. Moreover, pure
nonparametric estimates are the only possible
choice, when the prior knowledge of the system is
poor or uncertain.
Nonparametric approach offers simple
algorithms, which are asymptotically free of
approximation error, i.e. they converge to the true
system characteristics. However, the purely
nonparametric methods are not commonly exploited
in practice for the following reasons: (i) they depend
on various tuning parameters and functions; in
particular, proper selection of kernel and the
bandwidth parameter or orthonormal basis and the
scale factor are critical for the obtained results, (ii)
the prior knowledge of subsystems is completely
neglected; the estimates are based on measurements
only, and the resulting model may be not satisfactory
when the number of measurements is small, and (iii)
bulk number of estimates must be computed when
the model complexity grows large.
REFERENCES
Bai, E. W., Reyland, J., 2008. Towards identification of
Wiener systems with the least amount of a priori
information on the nonlinearity. Automatica. Vol. 44,
No. 4, pp. 910-919.
Bai, E. W., 2003. Frequency domain identification of
Wiener models. Automatica. Vol. 39, No. 9, pp. 1521-
-1530.
Bershad, N. J., Celka, P., Vesin, J. M, 2000. Analysis of
stochastic gradient tracking of time-varying
polynomial Wiener systems. IEEE Transactions on
Signal Processing. Vol. 48, No. 6, pp. 1676-1686.
Billings, S. A., Fakhouri, S. Y., 1977. Identification of
nonlinear systems using the Wiener model.
Automatica. Vol. 13, No. 17, pp. 502-504.
Boutayeb, M., Darouach, M., 1995. Recursive
identification method for MISO Wiener-Hammerstein
Model. IEEE Transactions on Automatic Control. Vol.
40, No. 2, pp. 287-291.
Celka, P., Bershad, N. J., Vesin, J. M., 2001. Stochastic
gradient identification of polynomial Wiener systems:
analysis and application. IEEE Transactions on Signal
Processing. Vol. 49, No. 2, pp. 301-313.
Giannakis, G. B., Serpedin, E., 2001. A bibliography on
nonlinear system identification. Signal Processing.
Vol. 81, pp. 533-580.
Greblicki, W., 1992. Nonparametric identification of
Wiener systems. IEEE Transactions on Information
Theory. Vol. 38, pp. 1487-1493.
Greblicki, W., 1997. Nonparametric approach to Wiener
system identification. IEEE Transactions on Circuits
and Systems -- I: Fundamental Theory and
Applications. Vol. 44, No. 6, pp. 538-545.
Greblicki, W., 2010. Nonparametric input density-free
estimation of the nonlinearity in Wiener systems.
IEEE Transactions on Information Theory. Vol. 56,
No. 7, pp. 3575-3580.
Greblicki, W., Mzyk, G., 2009. Semiparametric approach
to Hammerstein system identification. Proceedings of
the 15th IFAC Symposium on System Identification,
pp. 1680-1685, Saint-Malo, France.
Greblicki, W., Pawlak, M, 2008. Nonparametric System
Identification, Cambridge University Press, 2008.
Hasiewicz, Z., 1987. Identification of a linear system
observed through zero-memory non-linearity.
International Journal of Systems Science. Vol. 18, pp.
1595-1607.
Hasiewicz, Z., Mzyk, G., 2004. Combined parametric-
nonparametric identification of Hammerstein systems.
IEEE Transactions on Automatic Control. Vol. 49, pp.
1370-1376.
Hasiewicz, Z., Mzyk, G., 2009. Hammerstein system
identification by non-parametric instrumental
variables. International Journal of Control. Vol. 82,
No. 3, pp. 440-455.
Hunter, I. W., Korenberg, M. J., 1986. The identification
of nonlinear biological systems: Wiener and
Hammerstein cascade models. Biological Cybernetics.
Vol. 55, pp. 135-144.
Lacy, S. L., Bernstein, D. S., 2003. Identification of FIR
Wiener systems with unknown, non-invertible,
polynomial non-linearities. International Journal of
Control
. Vol. 76, No. 15, pp. 1500-1507.
Mzyk, G., 2007. A censored sample mean approach to
nonparametric identification of nonlinearities in
Wiener systems. IEEE Transactions on Circuits and
Systems -- II: Express Briefs. Vol. 54, No. 10, pp. 897-
901.
Mzyk, G., 2009. Nonlinearity recovering in Hammerstein
system from short measurement sequence. IEEE
Signal Processing Letters. Vol. 16, No. 9, pp. 762-
765.
Mzyk, G., 2010. Parametric versus nonparametric
approach to Wiener systems identification. Lecture
Notes in Control and Information Sciences. Vol. 404,
Chapter 8.
Mzyk, G., 2010. Wiener-Hammerstein system
identification with non-gaussian input. IFAC
International Workshop on Adaptation and Learning
in Control and Signal Processing.
Nesic, D., Bastin, G., 1999. Stabilizability and dead-beat
controllers for two classes of Wiener-Hammerstein
models. IEEE Transactions on Automatic Control.
Vol. 44, No. 11, pp. 2068-2071.
Pawlak, M., Hasiewicz, Z., Wachel, P., 2007. On
nonparametric identification of Wiener systems. IEEE
Transactions on Signal Processing. Vol. 55, No. 2, pp.
482-492.
Vanbeylen, L., Pintelon, R., Schoukens, J., 2009. Blind
maximum-likelihood identification of Wiener systems.
ICINCO2012-9thInternationalConferenceonInformaticsinControl,AutomationandRobotics
444