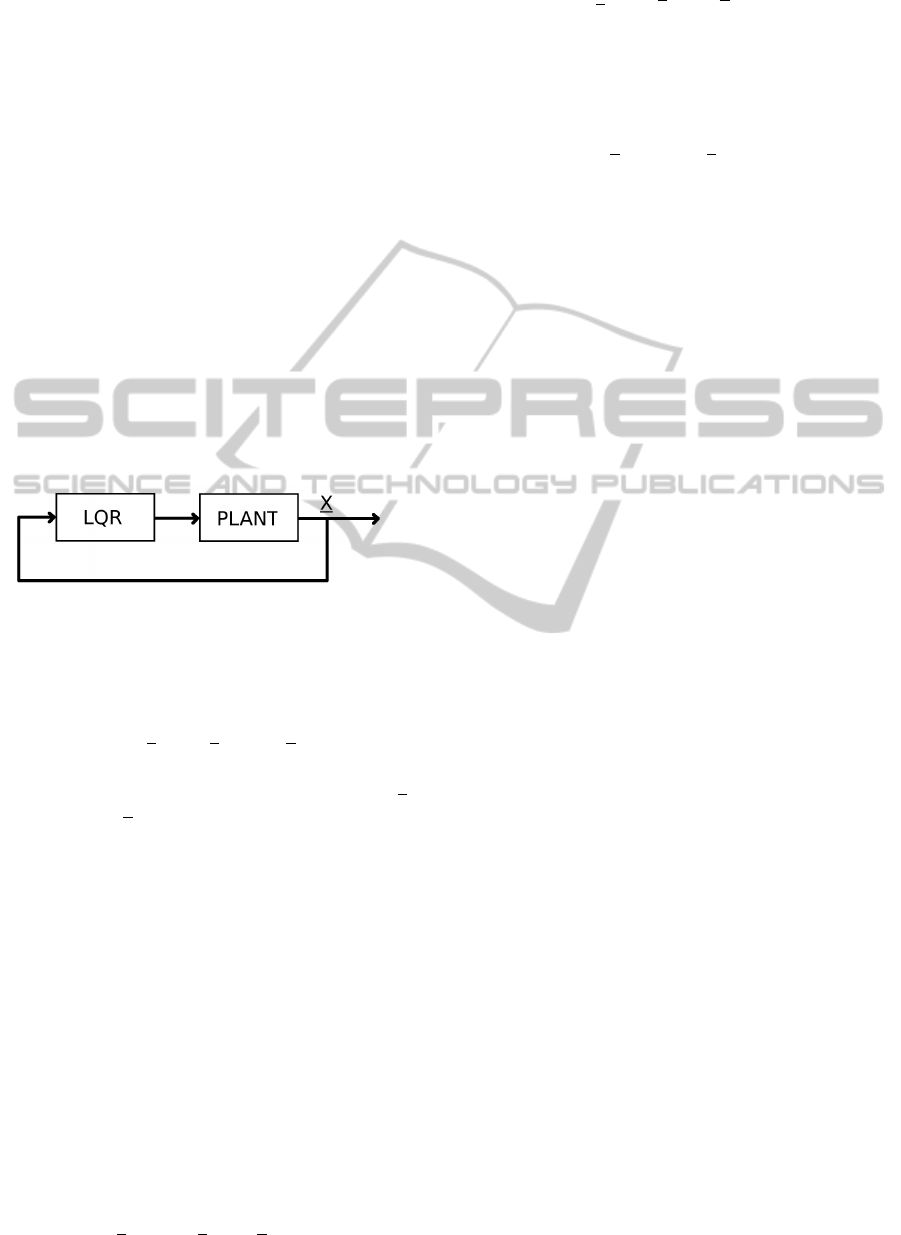
At this point very important is to set proper values for
the forgetting factor λ
0
and λ
0
. For maximum value
of 1, whole algorithm will be not sensitive for pa-
rameters change. On the other hand, small values for
λ’s can make RLS method internally unstable, which
could be observed as a oscillation in parameters.
5 LQR REGULATOR
The control system uses a linear quadratic regulator
LQR. It includes changing in time model which is lin-
earized in every point of trajectory. This solution is
suitable for stabilizing non-linear systems and it has
adaptive nature.
The LQR regulator can be applied to non-linear
system which is linerized along the trajectory. Then
the system matrices change in time.
The control aim is to make every state variable as
a desired value which is zero. The feedback uses the
whole state vector. Regulation system is in Fig. 3.
Figure 3: The LQR regulation system.
The control law is based on the optimal criterion
given by equation:
J =
n
∑
i=0
"
x
T
i
Q
LQR
x
i
+ R
LQR
u
2
i
#
, (26)
where Q
LQR
= Q
T
LQR
, J is quadratic cost function, x
i
is a state vector, u
i
is a control vector, n is a number
of iteration in regulator calculation process, Q
LQR
is a
state weight matrix and R
LQR
is weight of control sig-
nal. The aim is to minimize that quadratic criterion.
R
LQR
and Q
LQR
have constant values ((Wang et al.,
2010), (Zhang and Wang, 2010)):
R
LQR
= 100, (27)
Q
LQR
=
1 0 0
0 1 0
0 0 1
. (28)
This paper shows discrete time form of LQR equa-
tions with finite horizon which made it easer to imple-
ment it in the real application. In (Zabczyk, 1991) it
is shown how difficult solving continuous time LQR
equations is. For discrete problem it is needed to have
discrete state space model:
x
k+1
= A
d
x
k
+ B
d
u
k
, (29)
y
k
= C
d
x
k
+ D
d
u
k
, (30)
where A
d
, B
d
, C
d
and D
d
are discrete model matrices.
The author of (Sauer, 2011) describes how to obtain
them having linear model matrices. Here was used
approximate iterative method.
The control law is given by:
u
k+1
= −K
k
x
k
, (31)
where K
k
is a gain vector which equals to:
K
k
= (R
LQR
+ B
T
d
P
k
B
d
)
−1
B
T
P
k
A
d
. (32)
The P
k
is only unknown value which is the solution of
an iterative backward discrete time Riccati equation
((Yishao, 1996)):
P
k−1
= Q
LQR
+A
T
d
(P
k
−P
k
B
d
(R+B
T
d
P
k
B
d
)
−1
B
T
d
P
k
)A
d
,
(33)
with initial value P
n
= Q
LQR
. Sometimes it is
called ILQR (Iterative Linear Quadratic Regulator)
like in (In-Won et al., 2010) and (Li and Todorov,
2004).
6 RESULTS
In order to check every mentioned algorithms a proto-
type of an IWP was built. Using the computer simu-
lation acceptable mechanical parameters were found.
As a measurement unit, inertial sensor ADIS16355
from Analog Devices Company was used. It has high
precision tri-axis gyroscope and accelerometer. The
second important thing was the implemented – high
torque, DC current motor. It was direct drive for the
flywheel. Direct drive generates much less vibrations
than any mechanical transmissions. The last essential
thing was well-balanced flywheel. Every calculations
were made by using a personal computer.
Sensor fusion was the first thing, that authors had
to prepare and then check. In Fig. 4 comparison of
results of the Kalman Filter fusion is shown.
As it can be seen, accelerometer generates signal
with additional noise which is (i.a.) dynamic accel-
eration. In normal operation, it is impossible to esti-
mate declination from the pivot point by using only
accelerometer. By using fusion with gyroscope good
estimation can be obtained. In this example, authors
used proper settings for the fusion algorithm which
is: small variance (Q=0.0001) for gyroscope signal
and large variance (R=0.1) for accelerometer signal.
Right after fusion, algorithm of Extended Kalman
Filter performs his action. One of the estimates is
shown in Fig. 5. It is easy to see that Extended
Kalman Filter gives the opportunity to make estimates
robust for the noise, even from optical encoder. The
ControlandModelParametersIdentificationofInertiaWheelPendulum
577