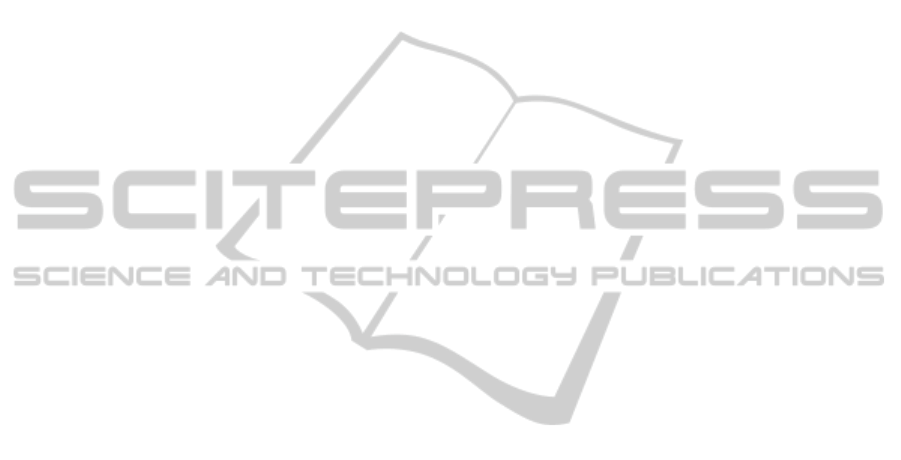
6 CONCLUSIONS
This paper has suggested new IFT algorithms meant
for parameter tuning of PI controllers dedicated to
the level control of the first two tanks in vertical
three-tank systems. A complete data-based
experiment-based approach is proposed with this
regard.
The experimental results show that the six steps
of our IFT algorithms ensure the performance
improvement of a representative nonlinear MIMO
benchmark process. An improved model reference
tracking is observed after few iterations of IFT
algorithms. The control system structure presented
in this paper does not employ an adaptive model
reference approach.
The results concerning the control system
behaviour with respect to modifications of
disturbance inputs have not been presented. The
integral components of PI controllers ensure the
disturbance rejection.
One limitation of this data-based technique is the
need for initial PI stabilizing controllers tuned by a
model-based approach represented, for example, by
the MO method. The integral components of PI
controllers cope with the cross-couplings specific to
MIMO systems. However, different organizations of
the experiments specific to MIMO systems can be
applied in this context in order to ensure further
control system performance improvement (Sjöberg
et al., 2003; Huusom et al., 2009; Rădac et al., 2009;
McDaid et al., 2010; Precup et al., 2010).
Future research will be focused on other data-
based tuning techniques applied to nonlinear MIMO
systems and on comparison of the performance of
similar tuning techniques. Special gradient
experiments for MIMO systems should be
constructed with this regard in order to fit the normal
operating regimes of control systems.
ACKNOWLEDGEMENTS
This work was supported by a grant of the Romanian
National Authority for Scientific Research, CNCS –
UEFISCDI, project number PN-II-ID-PCE-2011-3-
0109, and by a grant of the NSERC of Canada.
REFERENCES
Ahmed, S., Petrov, M., Ichtev, A., 2010. Fuzzy model-
based predictive control applied to multivariable level
control of multi tank system. In Proceedings of 5
th
IEEE International Conference Intelligent Systems (IS
2010). London, UK, 456-461.
Antić, D., Nikolić, S., Milojković, M., Danković, N.,
Jovanović, Z., Perić, S., 2011. Sensitivity analysis of
imperfect systems using almost orthogonal filters.
Acta Polytechnica Hungarica. 8, 79-94.
Åström, K. J., Hägglund, T., 1995. PID Controllers
Theory: Design and Tuning. Research Triangle Park,
NC: Instrument Society of America.
Bazanella, A. S., Gevers, M., Miskovic, L., Anderson, B.
D. O., 2008. Iterative minimization of H
2
control
performance criteria. Automatica. 10, 2549-2559.
Bigher, B.-A., 2011. Iterative feedback tuning-based
control structures. Applications to a multi-tank system
laboratory equipment. B.Sc. thesis, Faculty of
Automation and Computers, “Politehnica” University
of Timisoara, Timisoara, Romania.
Blažič, S., Škrjanc, I., Gerkšič, S., Dolanc, G., Strmčnik,
S., Hadjiski, M. B., Stathaki, A., 2009. Online fuzzy
identification for an intelligent controller based on a
simple platform. Engineering Applications of Artificial
Intelligence. 22, 628-638.
Ferreira, P. M., Ruano, A. E., 2009. On-line sliding-
window methods for process model adaptation. IEEE
Transactions on Instrumentation and Measurement.
58, 3012-3020.
Giua, A., Seatzu, C., 2008. Modeling and supervisory
control of railway networks using Petri nets. IEEE
Transactions on Automation Science and Engineering.
6, 431-445.
Hjalmarsson, H., 1998. Control of nonlinear systems using
iterative feedback tuning. In Proceedings of 1998
American Control Conference (1998 ACC).
Philadelphia, PA, USA. 4, 2083-2087.
Hjalmarsson, H., 2002. Iterative feedback tuning - an
overview. International Journal of Adaptive Control
and Signal Processing. 16, 373-395.
Hjalmarsson, H., Gevers, M., Gunnarsson, S., Lequin, O.,
1998. Iterative feedback tuning: theory and Applica-
tions. IEEE Control Systems Magazine. 18, 26-41.
Hjalmarsson, H., Gunnarsson, S., Gevers, M., 1994. A
convergent iterative restricted complexity control
design scheme. In Proceedings of 33
rd
IEEE
Conference on Decision and Control. Lake Buena
Vista, FL, USA, 1735-1740.
Huusom, J. K., Poulsen, N. K., Jørgensen, S. B., 2009.
Improving convergence of iterative feedback tuning.
Journal of Process Control. 19, 570-578.
Iglesias, J. A., Angelov, P., Ledezma, A., Sanchis, A.,
2010. Evolving classification of agents’ behaviors: a
general approach. Evolving Systems. 1, 161-171.
Inteco, 2007. Multitank System, User’s Manual. Krakow,
Poland: Inteco Ltd.
Johanyák, Z. C., 2010. Student evaluation based on fuzzy
rule interpolation. International Journal of Artificial
Intelligence. 5, 37-55.
Kasabov, N., Hamed, H. N. A., 2011. Quantum-inspired
particle swarm optimisation for integrated feature and
parameter optimisation of evolving spiking neural
networks. International Journal of Artificial
ICINCO2012-9thInternationalConferenceonInformaticsinControl,AutomationandRobotics
38