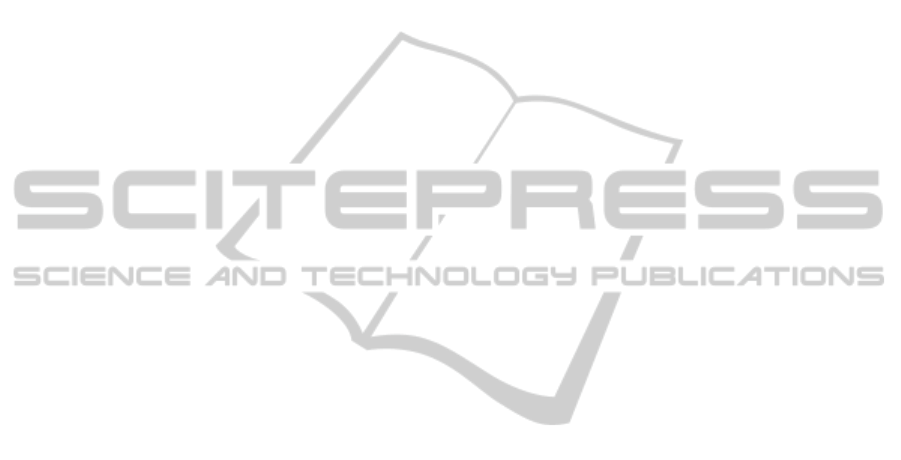
Comanici, G. and Precup, D. (2010). Optimal policy
switching algorithms for reinforcement learning. In
Proc. 9th International Conference on Autonomous
Agents and Multiagent Systems (AAMAS’10), pages
709–714.
Dimitrakiev, D., Nikolova, N., and Tenekedjiev, K. (2010).
Simulation and discrete event optimization for auto-
mated decisions for in-queue flights. Int. Journal of
Intelligent Systems, 25(28):460–487.
Drummond, C. (2002). Accelerating reinforcement learn-
ing by composing solutions of automatically identified
subtask. Journal of Artificial Intelligence Research,
16:59–104.
Enembreck, F., Avila, B. C., Scalabrini, E. E., and Barthes,
J. P. A. (2007). Learning drifting negotiations. Applied
Artificial Intelligence, 21:861–881.
Firby, R. J. (1989). Adaptive Execution in Complex Dy-
namic Worlds. PhD thesis, Yale University.
Galvn, I., Valls, J., Garca, M., and Isasi, P. (2011). A lazy
learning approach for building classification models.
Int. Journal of Intelligent Systems, 26(8):773–786.
Jordan, P. R., Schvartzman, L. J., and Wellman, M. P.
(2010). Strategy exploration in empirical games. In
Proc. 9th International Conference on Autonomous
Agents and Multiagent Systems (AAMAS’10), v. 1,
pages 1131–1138, Toronto, Canada.
Kittler, J., Hatef, M., Duin, R. P. W., and Matas, J. (1998).
On combining classifiers. IEEE Transactions on Pat-
tern Analysis and Machine Intelligence, 20(3):226–
239.
Le, T. and Cai, C. (2010). A new feature for approx-
imate dynamic programming traffic light controller.
In Proc. 2th International Workshop on Computa-
tional Transportation Science (IWCTS’10), pages 29–
34, San Jose, CA, U.S.A.
Mohammadian, M. (2006). Multi-agents systems for intel-
ligent control of traffic signals. In Proc. International
Conference on Computational Inteligence for Mod-
elling Control and Automation and Int. Conf. on Intel-
ligent Agents Web Technologies and Int. Commerce,
page 270, Sydney, Australia.
Pegoraro, R., Costa, A. H. R., and Ribeiro, C. H. C. (2001).
Experience generalization for multi-agent reinforce-
ment learning. In Proc. XXI International Conference
of the Chilean Computer Science Society, pages 233–
239, Punta Arenas, Chile.
Pelta, D., Cruz, C., and Gonzlez, J. (2009). A study on
diversity and cooperation in a multiagent strategy for
dynamic optimization problems. Int. Journal of Intel-
ligent Systems, 24(18):844–861.
Price, B. and Boutilier, C. (2003). Accelerating reinforce-
ment learning through implicit imitation. Journal of
Artificial Intelligence Research, 19:569–629.
Ribeiro, C. H. C. (1999). A tutorial on reinforcement learn-
ing techniques. In Proc. Int. Joint Conference on Neu-
ral Networks, pages 59–61, Washington, USA.
Ribeiro, R., Borges, A. P., and Enembreck, F. (2008). Inter-
action models for multiagent reinforcement learning.
In Proc. 2008 International Conferences on Compu-
tational Intelligence for Modelling, Control and Au-
tomation; Intelligent Agents, Web Technologies and
Internet Commerce; and Innovation in Software En-
gineering, pages 464–469, Vienna, Austria.
Ribeiro, R., Borges, A. P., Ronszcka, A. F., Scalabrin, E.,
Avila, B. C., and Enembreck, F. (2011). Combinando
modelos de interao para melhorar a coordenao em sis-
temas multiagente. Revista de Informtica Terica e
Aplicada, 18:133–157.
Ribeiro, R., Enembreck, F., and Koerich, A. L. (2006).
A hybrid learning strategy for discovery of policies
of action. In Proc. International Joint Conference
X Ibero-American Artificial Intelligence Conference
and XVIII Brazilian Artificial Intelligence Symposium,
pages 268–277, Ribeiro Preto, Brazil.
Sislak, D., Samek, J., and Pechoucek, M. (2008). Decentral-
ized algorithms for collision avoidance in airspace. In
Proc. 7th International Conference on AAMAS, pages
543–550, Estoril, Portugal.
Spaan, M. T. J. and Melo, F. S. (2008). Interaction-driven
markov games for decentralized multiagent planning
under uncertainty. In Proc. 7th International Confer-
ence on AAMAS, pages 525–532, Estoril, Portugal.
Strehl, A. L., Li, L., and Littman, M. L. (2009). Reinforce-
ment learning in finite mdps: Pac analysis. Journal of
Machine Learning Research (JMLR), 10:2413–2444.
Sutton, R. S. and Barto, A. G. (1998). Reinforcement Learn-
ing: An Introduction. MIT Press, Cambridge, MA.
Tesauro, G. (1995). Temporal difference learning and td-
gammon. Communications of the ACM, 38(3):58–68.
Watkins, C. J. C. H. and Dayan, P. (1992). Q-learning. Ma-
chine Learning, 8(3/4):279–292.
Zhang, C., Lesser, V., and Abdallah, S. (2010). Self-
organization for cordinating decentralized reinforce-
ment learning. In Proceedings of the 9th Interna-
tional Conference on Autonomous Agents and Multi-
agent Systems, AAMAS’10, pages 739–746. Interna-
tional Foundation for Autonomous Agents and Multi-
agent Systems.
ICEIS2012-14thInternationalConferenceonEnterpriseInformationSystems
238