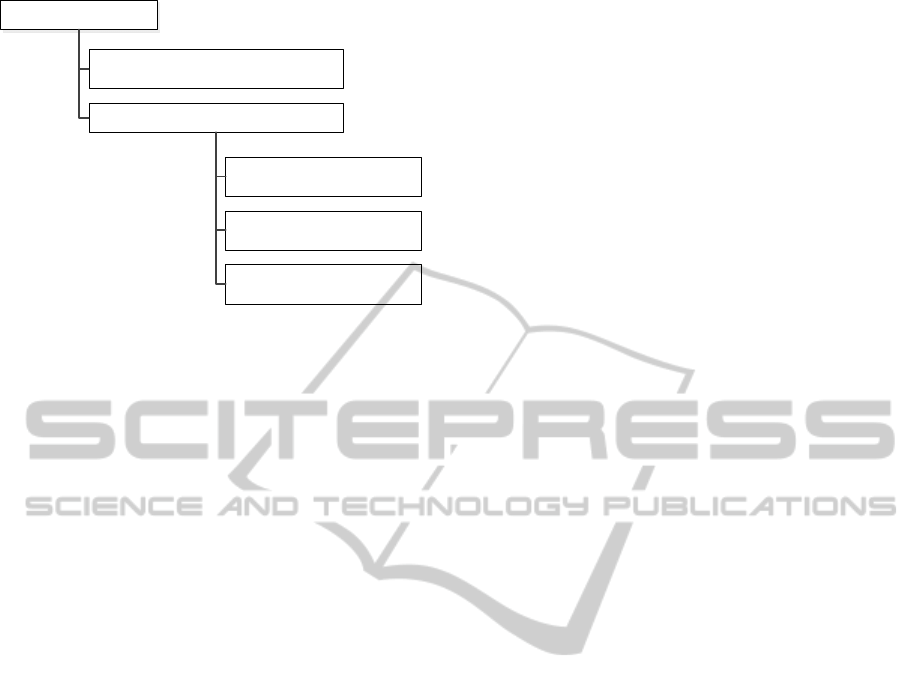
Figure 5: Solutions for the *[HJ]*Q* scenarios.
scenarios, we generated all the possible solutions for
every situation. This systematization allowed the
explicit selection of solution for every ambiguous
situation, giving rise to a set of inter-related
solutions referred to as strategies.
4 SUMMARY AND FUTURE
WORK
This paper presented an analysis and systematization
of the disambiguation process of automatically
created ontology alignments, and proposed the
characterization of ontology matching scenarios
through ten dimensions. As a result, the solutions are
identified per type and characteristics of scenarios.
The scenarios are categorized according to the
associated disambiguation and correction actions
(i.e. discard the correspondence between properties,
create relations between correspondences, or create
correspondences between the domain concepts).
This systematization followed a rigorous and
extensive identification of incompatibilities and
implications between the values of each dimension,
and the exhaustive identification and
characterization of all possible scenarios that can
occur from the initial situation, according to the
actions to be taken.
As a result of this systematization we developed
a semi-automatic system that identifies,
characterizes and solves the alignment scenarios,
transforming them to a data-integration-ready
alignment, based on a user-defined set of corrective
actions (strategies). The experiments carried out
demonstrate the completeness of the
systematization, i.e. all cases of ambiguity are
identified and addressed according to the previously
defined strategy.
We are currently working on the identification of
arbitrary type of correcting actions. In fact, the
identification of an ambiguous situation may lead to
arbitrarily complex actions, including triggering
(new) matching efforts focused on solving the
particular situation. Another trend concerns the
application of the proposed systematization and
disambiguation approach to more complex
scenarios.
ACKNOWLEDGEMENTS
This work is supported by FEDER Funds through
the “Programa Operacional Factores de
Competitividade - COMPETE” program and by
National Funds through FCT “Fundação para a
Ciência e a Tecnologia” under the project: FCOMP-
01-0124-FEDER-PEst-OE/EEI/UI0760/201 and
through the project World Search (QREN11495) of
FEDER.
REFERENCES
Altova, 2012. Altova MapForce – Graphical Data
Mapping, Conversion, and Integration Tool. Available
at: http://www.altova.com/mapforce.html.
Euzenat, J. et al., 2011. Results of the ontology alignment
evaluation initiative 2011. In Proc. of the 6th Int.
Workshop on Ontology Matching. Bonn, Germany.
Euzenat, J. & Shvaiko, P., 2007. Ontology matching 1st
ed., Heidelberg, Germany: Springer-Verlag.
Halevy, A., Rajaraman, A. & Ordille, J., 2006. Data
integration: the teenage years. In Very Large Data
Bases. Seoul, Korea, pp. 9–16.
Meilicke, C., Stuckenschmidt, H. & Tamilin, A., 2007.
Repairing ontology mappings. In Proceedings of the
22nd National Conference on Artificial Intelligence.
AAAI Press, pp. 1408–1413.
NeOn Foundation, 2010. Neon Plugins - NeOn Wiki.
Available at: http://neon-toolkit.org/.
Ritze, D. et al., 2009. A pattern-based ontology matching
approach for detecting complex correspondences. In
Proceedings of the 4th International Workshop on
Ontology Matching (OM). 8th International Semantic
Web Conference (ISWC). Chantilly, USA.
Silva, N. & Rocha, J., 2004. Semantic Web complex
ontology mapping. Web Intelligence and Agent
Systems Journal, 1(3-4), p.235―248.
Snoogle, 2007. Snoggle - A Graphical, SWRL-based
Ontology Mapper. Available at: http://snoggle.sem
webcentral.org/.
*[HJ]*Q* scenario
Discard properties correspondence
(discard)
Create concepts correspondences
Between all the domain
concepts (new3)
Between the super-domain
concepts (new2-ii)
Between the direct-domain
concepts (new2-i)
AutomaticOntologyAlignmentDisambiguationbasedonOntologicalStructuralDimension
167